Abstract
Noise control is one of the most critical technical indicators to improve the performance of intelligent audio recognition system. Based on the noise cancellation technology, a distributed low noise amplification circuit design was proposed, and the PE15-0P technology was applied to realize broadband low noise amplification. The amplifier circuit used diodes and resistors for voltage division, which effectively achieved bias saturation at the transistor and then diode structure. According to the design of low noise amplifier, the noise output characteristics were simulated and analyzed. An audio enhancement method based on noise type recognition was proposed, which can optimize noise estimation by selecting parameter combinations according to noise type, so as to improve the quality and intelligibility of noise frequency signals in various noise environments. From the aspects of hardware and algorithm design, the noise signal was comprehensively reduced, and the accuracy of audio recognition was significantly improved.
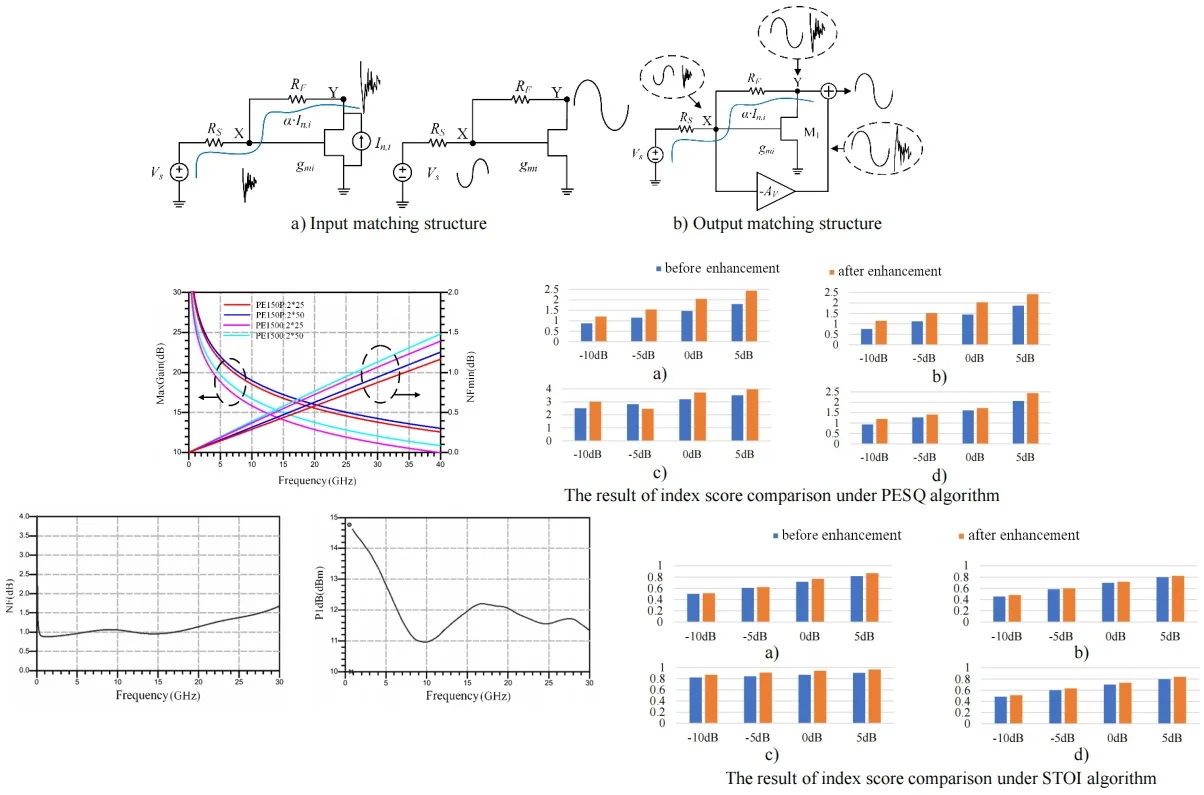
Highlights
- Based on the noise cancellation technology, a distributed low noise amplification circuit design was proposed, and the PE15-0P technology was applied to realize broadband low noise amplification.
- The amplifier circuit used diodes and resistors for voltage division, which effectively achieved bias saturation at the transistor and then diode structure.
- According to the design of low noise amplifier, the noise output characteristics were simulated and analyzed.
1. Introduction
Noise control is one of the most important indicators for evaluating the performance of audio equipment. With the rapid development of artificial intelligence and the widespread application of electronic technology, smart classroom system is increasingly used in English teaching for voice recognition equipment, etc. These applications cannot be separated from classic audio signal processing techniques, such as audio detection technology used to determine the location of target audio and audio enhancement technology used to reduce the influence of environmental noise. In actual work environments, there are often a large number of different types of noise in the process of acquiring and transmitting audio signals, which can interfere with the target signal and cause stronger background noise to mix into the audio signal, obscuring its essential features, which is one of the main reasons for system output errors or errors. In the case of 5 dB SNR, the average recognition accuracy of conventional speech recognition system is less than 50 %, while in the case of 10dB SNR, the average recognition accuracy can be improved to more than 70 %. The participation of different noises puts forward higher requirements for audio detection and enhancement methods. In order to improve the audio accuracy, it is necessary to enhance the noise frequency signal. Existing audio signal enhancement methods rely on statistical features, but their performance deteriorates dramatically when noise conditions change or are mismatched. The combination of broadband technology and low noise amplifier has become an important research direction of low noise amplifier design. There is also an increasing demand for wideband low noise amplifiers. Therefore, a distributed low noise amplifier integrated circuit design based on noise cancellation technology is proposed in this paper, which can improve the accuracy and noise suppression ability of audio equipment through noise recognition enhancement.
2. Design of noise cancellation circuit
2.1. Design of circuit structure
The audio equipment necessitates a low noise amplifier with adequate gain, minimal noise figure, good linearity, and effective input matching. If the input of the low noise amplifier is poorly matched, it may result in passband ripple and stopband attenuation of the prefilter, thereby diminishing receiver performance. In the design of wideband low noise amplifiers, there exists a trade-off between noise and input matching. Certain negative feedback structures can account for the relationship between noise and input matching; however, they pose stability issues. To address this issue, a noise cancellation structure is implemented as depicted in Fig. 1. The principle of the noise cancellation structure is to combine the auxiliary amplifier, so that the useful signal is superimposed in the same direction at the output end, and the noise is reversed superimposed at the output end to cancel. Under the joint action of the auxiliary amplifier and the matching circuit, the signal is superimposed in the same direction at the output end, so the whole circuit amplifies the useful signal and counteracts the noise of the matching circuit transistor, thus realizing the effect of peer superposition of useful signal while noise cancellation. Since noise figure is an important index of low noise amplifier, broadband matching can be realized based on noise cancellation structure. Therefore, the circuit design combined with noise cancellation structure is one of the key points in low noise amplifiers. In the improved design of wideband low noise amplifier, the matching relationship between noise and input is more clear, so the stability problem can be effectively solved.
Fig. 1Structure and composition of shock absorber
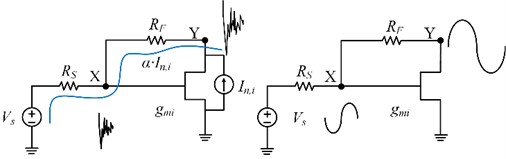
a) Input matching structure
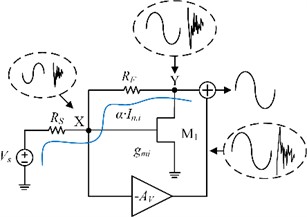
b) Output matching structure
2.2. Process selection
The design provides PE15-0P 0.15 μm GaAspHEMT process, which contains two noise models of MS and CPW transistors, also enhanced power device models. Both transistors have a gate-source voltage range of –0.3-1.2 V and a drain-source voltage range of 0-4 V. In order to select a suitable process to design a wirelessly low noise amplifier, CPW transistors of the PE15-00 process and the PE15-0P process are compared. Under the same transistor size and bias conditions, the maximum gain and minimum noise coefficients of the two processes are shown in Fig. 2. It can be seen that the maximum gain of the CPW transistor of PE15-0P process is higher than that of the CPW transistor of PE15-00 process, and the minimum noise is lower than that of the coplanar waveguide transistor of PE15-00 process under the same size and the same bias conditions. Therefore, the PE15-0P process is used in this design to design broadband low noise amplifier. In order to obtain higher gain and better port isolation, the gain unit adopts common source and common gate structure. Because the MS transistor source is fixed and grounded, it is difficult to design the common source and common gate structure. The CPW transistor source pole is suspended, and the source pole can be flexibly connected to the device, so the CPW transistor with the same noise model is selected.
Fig. 2Process maximum gain and minimum noise figure comparison diagram
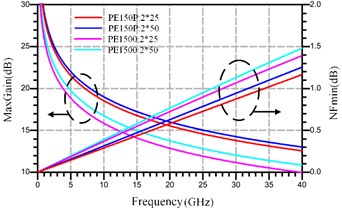
2.3. Design of low noise amplifier
The low frequency noise factor of the diode-feedback distributed low noise amplifier is primarily reduced by increasing the gate resistance. However, the linearity of this structure is compromised due to the diode feedback, which reduces the overall linearity of the amplifier. By utilizing a noise cancellation structure in conjunction with an input matching structure, it is possible to achieve broadband matching without compromising circuit stability. Therefore, consideration should be given to applying noise cancellation technology in the design of distributed low noise amplifiers in order to achieve simultaneous noise reduction. The distributed low noise amplifier based on diode resistance partial voltage reduction is shown in Fig. 3, and the main part of its circuit is designed as a distributed structure. A common source amplifier with resistance feedback is added to the grid terminal of the circuit, and the common source amplifier with resistance feedback is used as a matching structure to achieve good input matching. The resistance-feedback common source amplifier and the distributed structure work together to form a noise canceling structure, which cancels the noise while matching the broadband. Because the bias of the common-source common-gate structure is greater than that of the common-source transistor, the influence of the input matching structure on the output matching and the output on the input is reduced. Reference diode-feedback distributed low noise amplifier structure, using diode and resistor for voltage division, due to the existence of resistance feedback common source amplifier, the diode structure of the transistor is in the saturation region of bias.
Fig. 3Distributed low noise amplifier structure with partial pressure noise reduction
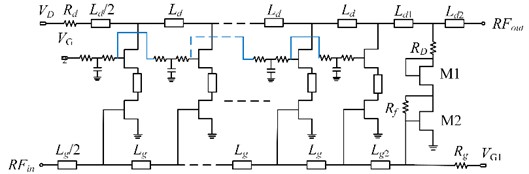
2.4. Noise reduction simulation and analysis
According to the design of low noise amplifier, the noise output characteristics can be simulated and analyzed. The noise figure simulation results are shown in Fig. 4. It can be seen that the minimum noise figure is 0.9 dB, and the noise figure of the denoising distributed low noise amplifier based on diode resistor voltage divider is less than 1.5 dB in the frequency band from 0.5 to 26.5 GHz. The noise figure is less than 1.7 dB at 30 GHz. It can be concluded that the designed low noise amplifier has low noise at low frequencies, which is a great improvement over the conventional distributed amplifier. The output P1dB of distributed low noise amplifier based on diode resistance partial voltage noise reduction is shown in Fig. 5. It can be seen that in the frequency range of 500 MHz-30 GHz, it is 11-14.9 dBm, which has a great improvement effect on noise suppression.
Fig. 4Output change curve of noise figure
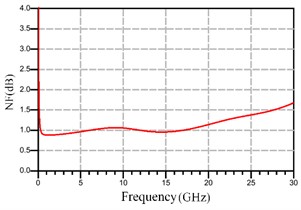
Fig. 5Output change curve of P1dB
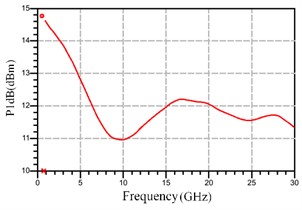
3. Design of audio recognition enhancement scheme
3.1. The selection of noise optimization scheme
The IMCRA-OMLSA audio enhancement method, a statistics-based approach, is utilized. When estimating the noise signal for each frame, full consideration is given to the correlation between each frame of the audio signal. However, due to the diverse statistical characteristics and stability of different types of noise, the correlation between power spectrum, noise spectrum and signal-to-noise ratio of each frame signal varies. In order to adjust this correlation, the basic IMCRA method incorporates smoothing parameters and weight parameters in the prior SNR estimation during calculation. Typically, these three parameters are set based on relevant experience and remain fixed. However, in practical scenarios with various types of noise signals, using fixed parameters for power spectrum estimation may not meet actual needs. Therefore, in order to more accurately calculate the noise power spectrum in different noise environments, an appropriate combination of estimation parameters should be selected according to the specific type of noise. Objective calculation method can be used to evaluate the subjective listening effect of speech. The loudness spectrum of the signal is obtained by auditory transformation during calculation, and then a value is obtained by comparing the loudness spectrum difference between the enhanced speech signal and the pure speech signal, which is approximate to the average opinion score. The audio enhancement method based on noise type recognition has distributed characteristics. First, the audio end point detection method based on spectral subtraction and naive Bayes is used to detect noise frames. Once several consecutive frames are detected as noise frames, the audio type recognition method based on ECAPA-DNN is used to determine the noise type. Then the optimal parameter combination corresponding to the noise type is substituted into IMCRA method to estimate the noise power spectrum. Finally, the OMLSA method is used to estimate the audio frequency to obtain the estimated pure audio signal.
3.2. Index score test results and comparative analysis
According to the functional requirements of audio recognition device, different noise suppression algorithms have been applied and validated, including PESQ and STOI. PESQ can be used to evaluate the subjective listening effect of speech by objective calculation method, and the score value ranges from [–0.5 to 4.5]. The larger the score value in this range, the better the enhancement effect of the method. STOI is a standard used to evaluate the clarity of speech signals by calculating the correlation between the pure speech signal and the enhanced speech signal's short-term envelope. The score is between 0 and 1, and the higher the value in this range, the higher the intelligibility of the signal.
Under random conditions, the PESQ and STOI index scores of noisy speech signals with different types of noise and different SNR before and after enhancement are shown in Fig. 6 and Fig. 7, respectively. It can be seen from the two figures that the PESQ and STOI scores of noisy speech signals with different SNR are improved to a certain extent by the processing of the audio enhancement method based on noise type recognition. When the SNR is –5 dB, 0 dB and 5 dB, the two index scores of the enhanced noisy speech signal are improved. At the SNR of –10 dB, the performance degrades slightly. The PESQ score of noisy speech signal after enhancement is 0.488 higher than that before enhancement, and the STOI score is 0.036 higher than that before enhancement. According to the two objective evaluation indexes in the experimental results, the proposed audio enhancement method based on noise type recognition effectively improves the quality of noisy speech signals.
Fig. 6The result of index score comparison under PESQ algorithm
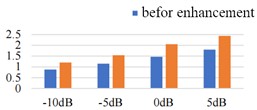
a)
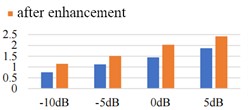
b)
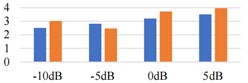
c)
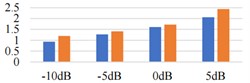
d)
Fig. 7The result of index score comparison under STOI algorithm
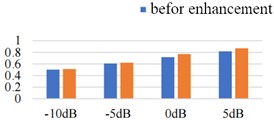
a)
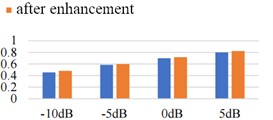
b)
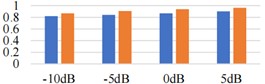
c)
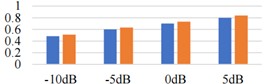
d)
Fig. 8Speech spectra under different algorithms
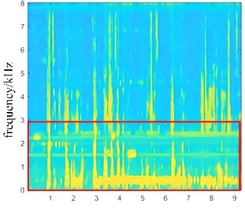
a) Initial signal
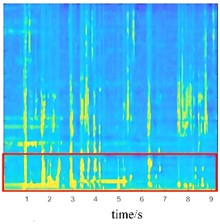
b) PESQ algorithm processed
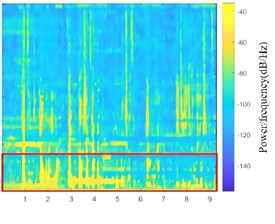
c) STOI algorithm processed
3.3. Spectrogram analysis of speech signals with noise
For Spectrogram analysis of audio, periodogram method was applied in this paper. First, the observed data of the random signal is treated as an energy limited signal, and the Fourier transform can be directly taken to obtain . Then, the square of its amplitude is taken again and divided by as an estimate of the true power spectrum . Its expression is:
In the complex actual scene, the test algorithm can reduce part of the impact of audio noise according to the extracted information. In order to improve the reliability of the comparative analysis, all noises are assumed to be stationary. In the non-stationary noise environment, the error has no obvious effect on the test results. The results of spectral analysis in a noisy environment are shown in Fig. 8. It can be seen that the PESQ and STOI algorithms have a very obvious effect on noise suppression. Through processing, the speech signal distortion is less, the residual noise component is less, and the frequency component of the speech signal is better preserved.
4. Conclusions
1) With the support of the noise cancellation circuit designed and applied, the audio signal can be synchronized through the auxiliary amplifier and matching circuit, and output in the same direction superposition, so the noise reduction circuit not only amplifies the useful signal, but also counteracts the noise of the transistor, thereby effectively improving the quality of the audio signal and providing an important basis for the improvement of the audio recognition ability.
2) Through the simulation and testing of different audio signal noise processing algorithms, it can be shown that the noise recognition method can significantly improve the objective evaluation index under different noise environments. Besides. Using the optimized log-spectral amplitude estimation and target audio estimation, the quality of the noise frequency signal can be improved, especially the intelligibility of the noise frequency signal.
References
-
Q. Yuan et al., “Analysis of effect of pump light wavelength on the magnetic noise suppression of the SERF co-magnetometer,” Sensors and Actuators A: Physical, Vol. 374, No. 1, p. 115471, Aug. 2024, https://doi.org/10.1016/j.sna.2024.115471
-
D. P. Yang et al., “Auxiliary active noise control system based on signal reconstruction,” Mechanical Systems and Signal Processing, Vol. 212, No. 11, p. 111287, Apr. 2024, https://doi.org/10.1016/j.ymssp.2024.111287
-
K. Myagila and H. Kilavo, “A comparative study on performance of SVM and CNN in Tanzania sign language translation using image recognition,” Applied Artificial Intelligence, Vol. 36, No. 1, pp. 105–118, Dec. 2022, https://doi.org/10.1080/08839514.2021.2005297
-
T. H. Khan and K. A. Wahid, “An advanced physiological data logger for medical imaging applications,” EURASIP Journal on Embedded Systems, Vol. 2012, No. 1, pp. 1–14, Dec. 2012, https://doi.org/10.1186/1687-3963-2012-10
-
J. Huchtkoetter and A. Reinhardt, “A study on the impact of data sampling rates on load signature event detection,” Energy Informatics, Vol. 2, No. S1, pp. 1–12, Sep. 2019, https://doi.org/10.1186/s42162-019-0096-9
-
H. Nazki, S. Yoon, A. Fuentes, and D. S. Park, “Unsupervised image translation using adversarial networks for improved plant disease recognition,” Computers and Electronics in Agriculture, Vol. 168, No. 1, p. 105117, Jan. 2020, https://doi.org/10.1016/j.compag.2019.105117
About this article
The authors have not disclosed any funding.
The datasets generated during and/or analyzed during the current study are available from the corresponding author on reasonable request.
The authors declare that they have no conflict of interest.