Abstract
Sound source identification is an important prerequisite for noise control. In recent years, new methods of sound source identification have been developed to improve the robustness of source identification. In this paper, a comprehensive review of noise source identification methods is developed to summarize its developing status and engineering applications. At first, a microphone array which is to acquire the sound pressure field is described. And then, the sound field visualization methods (beamforming and acoustic holography) and their advantages and disadvantages are reviewed. Finally, to improve the identification accuracy of the moving sound source, the hybrid acoustic holography methods with more generality and robustness are reviewed. Through the comparison and summary of the sound source identification methods, we expect this work may provide a potential guidance for the subsequent research studies in the field of sound source identification.
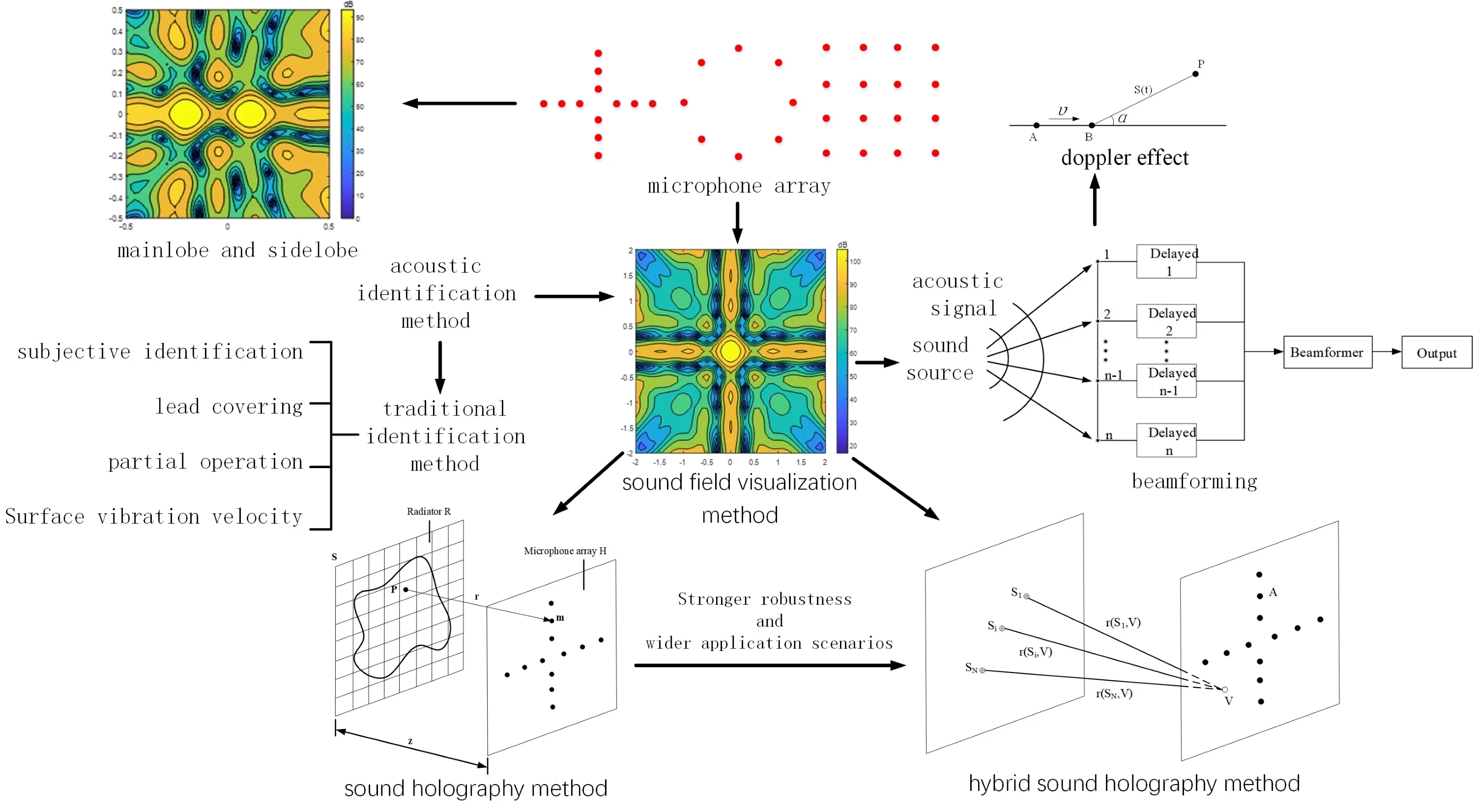
Highlights
- The microphone array, sound field visualization method and hybrid sound holography method with better comprehensive performance are emphasized.
- The merits and demerits of beamforming, acoustic holography and hybrid acoustic holography and their application scenarios are discussed.
- A large number of sound source identification cases are compared and summarized.
1. Introduction
Mechanical vibration of objects is one of the main sources of sound in industrial production, it is particularly important to identify the sound source accurately for noise analysis and control. As the convenience of calculating acoustic information and realizing the location of component sound source, the sound source identification technology is widely used in engineering, such as military, medicine, ocean exploration, electronic communication and so on [1-5]. At present, there are many methods for sound source identification, which can be divided into traditional identification method (such as sound intensity method, surface vibration method, etc.), sound field visualization technology, etc. [6-8]. The traditional identification methods mainly include subjective identification method, lead covering method, partial operation method and surface vibration velocity method. Subjective identification method is greatly affected by human factors, and it is difficult to give quantitative analysis when multiple sound sources exist simultaneously [9]. Lead covering method uses lead plate (sound absorbing material) to cover all sound sources, and then opens a small opening on the sound source to expose it to achieve the purpose of measuring specific sound sources. This method is only suitable for medium and high frequency sources [10]. The partial operation method firstly measures the overall noise condition under the condition that all the components are running at the same time, and then stops the operation of the components to be observed one by one, and calculates the noise contribution of each measured component through theoretical derivation. The operation process of this method is cumbersome, and part of the acoustic contribution may be caused by the noise generated by the consistent natural frequency between components, so the measurement results are unstable and it is easy to get conclusions contrary to the facts [11]. Surface vibration velocity method is to arrange acceleration sensors on the surface of the object that produces vibration, and determine the noise size by the normal vibration velocity and amplitude [12]. As the operation process of traditional identification method is cumbersome, and the measurement results are easy to be disturbed by external factors, additional processing such as sensor arrangement is needed to achieve better noise source identification effect [13, 14], this method has been gradually phased out.
With the continuous development of information technology, sound source identification methods based on signal processing and image identification technology are developed to overcome the defects of traditional sound source identification technology, which can be called sound field visualization methods. These methods employ microphone array arranged in a certain way to collect the phase, amplitude, orientation and other information of the noise source, and then process the data and apply visual graphics to realize the noise source identification. Compared with the traditional identification method, the visualization method of sound field is easy to operate, and corresponding processing methods can be selected for different signals with different characteristics. The visualization method can not only identify surface sound source and internal sound source, but also identify different frequency components of the same sound source. No matter for stationary vibration signal or non-stationary vibration signal, it has good recognition effect.
The sound field visualization methods mainly include beamforming and acoustic holography methods [15]. Beamforming is a very simple and practical array signal processing algorithm in acoustic imaging technology, which is suitable for identification of medium and high frequency sound source and measurement of medium and long distance. But this method is limited by the number of array elements and array aperture, so the mainlobe of the synthesized beam is wider and its resolution is limited by Rayleigh resolution criterion [16]. Although beamforming technology has some limitations, such as the need for a large number of sensors and high cost, it has great engineering application value. Acoustic holography is to measure the sound pressure hologram through the holographic measuring surface surrounding the sound source, and then reconstruct the sound field from the sound pressure of the holographic surface based on the spatial field transformation relationship between the source surface and the holographic surface [17]. This method has strong anti-interference ability, can identify the main noise sources and their frequency characteristics on the object surface in complex sound field environment, which is suitable for the identification of low-frequency noise sources, but the identification ability of high-frequency signals needs to be further improved [18]. At present, sound field visualization methods are widely used in engineering, for instance, used in the fault diagnosis field combining signal processing and image processing technology [19] (as shown in Fig. 1) which can establish a complete array identification system and achieved good results.
Fig. 1Fault diagnosis process based on pattern identification of sound and image
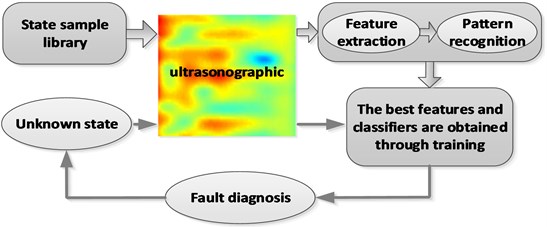
To sum up, traditional identification methods are cumbersome, inefficient and inaccurate, and are difficult to diagnose the faults of precision instruments. The sound field visualization methods have improved the identification efficiency and accuracy and have the advantages of high robustness, strong identification ability, clearly and intuitive identification results. In this paper, the beamforming, acoustic holography and hybrid acoustic holography method are mainly discussed. Firstly, the development process of microphone array and the applicable scenarios of different array types are introduced, then the beamforming algorithm and application examples are discussed; secondly, the development of generalized inverse beamforming is discussed combined with practical cases; thirdly, the basic theory of acoustic holography which focuses on the equivalent source method, static sound source identification and moving sound source identification is introduced; and then the hybrid acoustic holography methods and its advantages and disadvantages in solving practical problems are discussed, which come from the combination of the beamforming, acoustic holography and wave superposition; finally, the development of noise source identification methods based on microphone array is summarized.
The outline of the paper is as follows: Section 2 and Section 3 introduce the beamforming and acoustic holography methods in detail. In Section 4, the hybrid acoustic holography is discussed. The paper ends with the summary.
2. Microphone array
Microphone array is the basis of sound field visualization, which can receive various sound signals and combine these signals with corresponding algorithms to realize the recognition and location of sound source. Sound field visualization technology uses the sound field analysis method for reverse reconstruction, by displaying the reconstruction results in the corresponding image to express the abstract sound field, the location and sound field characteristics of the noise source are directly reflected, which provides convenience for the application of sound source identification in practical engineering. In the following part, the microphone arrays and several common sound field visualization methods are described in detail.
The microphone array is first developed as air defense equipment during World War I. And then, other scholars demonstrated the feasibility of this approach in beamforming through experiments [20]. The experiment results show that different microphone array arrangement produce different degrees of sidelobe effect, and the stronger the sidelobe effect is, the greater the influence on the measurement accuracy. A large number of scholars have adjusted the structure of the sensor array (such as cross (Fig. 2(a)), ring (Fig. 2(b)), rectangle (Fig. 2(c)), etc.). On this basis, Bertsch et al [21] have developed the coordinates of a group of microphone arrays with the function of weakening the sidelobe effect through Monte Carlo simulation, and used the array for noise measurement during aircraft landing. The results show that the phenomenon of false sound source is improved under the weaker sidelobe effect and the array's ability to identify noise source is improved effectively.
Fig. 2Main forms of microphone array
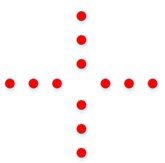
a)
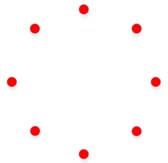
b)
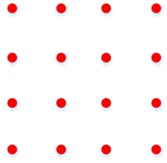
c)
In addition, to explore the relationship between sidelobe effect and measurement accuracy, Hald et al. [22] have designed several groups of two-dimensional microphone arrays with strong sidelobe suppression ability on the basis of orthogonal optimization algorithm; to obtain the fan-shaped wheel array, Hald et al. have optimized the wheel-shaped array in two-dimensional microphone arrays [22, 23], which create hardware conditions for the combination of acoustic holography and beamforming technology; Barsikow et al. [24] have applied the fan-shaped wheel microphone arrays to identify the noise sources of moving trains, and verified the feasibility of the method through experiments, which laid a foundation for the identification of moving sound sources by hybrid acoustic holography. In addition, a technology for acoustic measurement of large equipment using a small number of microphones has been developed, which partly solve the problem of data processing difficulties caused by a large number of arrays. Shah et al [25] have used this technology to measure the body noise of an airbus aircraft model made according to 1:11 and obtained ideal identification results. Starting from the perspective of frequency domain analysis, Harvey et al. [26] have used the frequency domain beamforming algorithm as the theoretical basis, and use the two-dimensional microphone array to measure the noise caused by stable airflow in different environments and realize the sound source location. The measurement results are consistent with the facts, which prove the practicability of the frequency domain method. Although the two-dimensional microphone array can shield the signal interference in the direction of noise source, it can’t achieve good weakening effect on the reflection and background interference behind the array [27]. Therefore, the two-dimensional microphone array is mostly used for indoor sound source identification with silencing equipment.
In conclusion, the design and measurement technology of microphone array has made great progress in practice. At present, a large number of scholars have committed to research this technology.
3. Beamforming
3.1. Beamforming algorithm
Beamforming is an advanced acoustic frontier technology for noise source identification, location and spatial sound field visualization. The expression of the algorithm is formulated as follows:
in which, pi(t) is the signal received by the i microphone in the array of N microphones, it’s also the input signal to the beamformer, wi is the weighting coefficient,τi is the signal delay, b(t) is the output signal of the beamformer.
Beamforming method is a mathematical method to process the acoustic signal collected by microphone array. As shown in Fig. 3, the beamforming is implemented as follows: Firstly, sound field data including target signal and noise are collected by two-dimensional or three-dimensional microphone array arranged in space; secondly, the data obtained in the previous step are processed by linear weighted combination to get the signal output. In this signal, the mainlobe and sidelobe can be separated by the position of the sound source and microphone, and then by strengthening the mainlobe signal and weakening the sidelobe signal, the sound source identification can be realized [28]. This technology is suitable for the measurement of medium and high frequency noise source signals. Compared with other methods, it has more advantages in medium and long distance measurement range.
As the beamforming method has the main functions of improving the signal to noise ratio of acoustic data, giving microphone array directionality, providing the conditions required for sound source identification, etc. To evaluate the performance of beamformer, we usually start with several important indexes such as mainlobe width and response, array gain, sidelobe level, robustness and frequency response. And Optimization of these parameters can achieve the best comprehensive effect. For example, Zhang et al. [29] have improved regularization algorithm by updating the output of the results can focus the mainlobe of the acoustic cloud image to the actual coherent sound source point; Arcondoulis et al. [30] have set up microphone array in irregular shape area, which obtains better identification ability than logarithmic spiral array, and also obtains better results in other performance evaluation indexes, the beamforming method with constant direction obtained after the optimization of the original algorithm can reduce the number of microphone without changing the mainlobe width and the identification performance of the array.
Fig. 3The principle of Beamformers
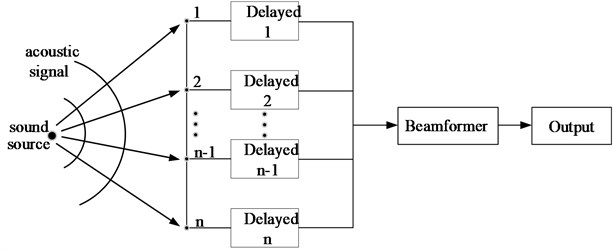
Delay-and-sum is the earliest beamforming algorithm, which is also a classical array signal processing method [31]. This method is simple but inefficient, moreover, the effect is not ideal in reducing the width of the main lobe and weakening the side lobe (as shown in Fig. 4, the mainlobe and sidelobe are exist simultaneously). Too wide mainlobe will affect the resolution of sound source identification, and the appearance of sidelobe will form noise on the sound source image. At present, two methods are usually used to reduce the mainlobe width and weaken the sidelobe effect. One is elimination method, which weakens the sidelobe effect by eliminating the cross spectrum generated by the known sound source in the cross-spectrum matrix of the existing signal. Cross-power spectrum is used to measure the frequency response of unknown linear systems in communication signal processing, it can also be used to estimate the time-delay of systems such as acoustic receiving signals. Rakesh [32] have used this algorithm to study the influence between microphone array size and quantitative aircraft body noise source; Oerlemans [33] identified the noise source of Airbus A360 model wing. Although the cross-power spectrum delay-and-sum algorithm can identify the noise source more accurately, it cannot truly reflect the real intensity of the sound source. Therefore, Hald et al [25] have further improved the cross spectral delay summation algorithm, and introduced the cross spectral difference function between the model sound field and the real sound field into the cross spectral imaging function algorithm. Compared with the cross spectral delay summation algorithm, the improved algorithm considers the difference of signal acquisition between microphones, and can accurately reconstruct the real intensity of sound source. Brooks has proposed a deconvolution acoustic source imaging (DAMAS) method [34], which extracts sound source information by solving linear equations about beamforming output results and real source coordinates with positive constraints. On this basis, Brooks extends the deconvolution sound source imaging method to the noise source identification of three-dimensional sound field, and then gives damas-c and sc-damas algorithms successively; then, some scholars imitate damas2 method, convert spatial convolution into wavenumber domain product, and give fft-nnls method, which improves the calculation efficiency and accuracy of sound field [34].
In addition, when beamforming algorithm is used in underwater acoustic imaging, the far-field imaging ability is well, but the near-field imaging ability is insufficient. Under this background, a pruned distributed parallel subarray (p-dps) algorithm and array design method are used to solve this problem. This method divides the 3D scene into multiple focal lengths according to the distance, transmits signals to different focal length to achieve the purpose of real-time imaging, which can improve the problems of slow calculation speed and low sparsity rate of beamforming technology on sparse array. To make up for the shortcomings of microphone signal pickup performance and signal post-processing technology, guo [35] first uses beamforming algorithm to suppress noise, then uses the optimal improved logarithmic spectral amplitude combined with depth neural network to train the masking value of multi-objective function, and proposes a filtering method to eliminate residual noise signal; the algorithm is robust to speech signal input in non-ideal state.
Fig. 4Mainlobe and sidelobe
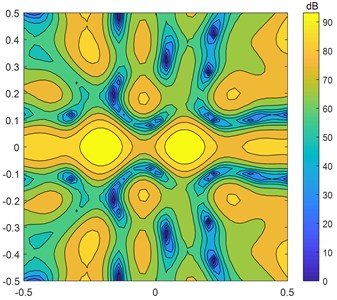
The adaptive beamforming algorithm has made great contribution to improve the robustness and pick-up quality of speech identification technology. Based on the conventional beamforming microphone speech pickup system, Zhang et al. [36] have used the optimization method based on the minimum criterion to optimize the algorithm of the conventional beamforming microphone speech pickup system. The results show that it has made a reasonable compromise between the array gain and other indicators, and the interference signal in the low frequency band has been significantly reduced, the quality of speech pickup is significantly improved. The improved deconvolution sound source imaging algorithm based on convex minimization method is used in simulation experiments. The experimental results show that this method has better spatial resolution and dynamic range. There some scholars use multi-channel point sound source signals to simulate a point sound source with known actual sound source, according to the position of each microphone in the microphone array and combined with the theoretical calculation method of sound field, they obtain the size of each acoustic index that should be collected by each microphone, and then locate the actual sound source through beamforming algorithm, the positioning results are compared with the calibrated results to determine the sound field fitting degree, so as to realize the calibration of microphone array. Philip have used regular or irregular sensor array for beamforming algorithm to effectively identify the sound source of large equipment [37]. The experimental results of a large number of relevant experiments show that the traditional beamforming identification method is difficult to accurately locate the moving sound source. Therefore, some scholars first eliminate the Doppler-effect on the basis of the traditional beamforming algorithm, and then use that to identify the noise source of the vehicle in motion, the results show that the spectral characteristics obtained by the new algorithm are more obvious. Subsequently, the post filter beamforming algorithm based on time difference of arrival (TDOA), adaptive spectrum noise reduction (ASNR) and multi-source selection (MSS) has been proposed successively, which realizes the selection of filtering low-frequency, intermediate frequency or high-frequency noise through manual operation, and improves the signal-to-noise ratio of the output signal.
3.2. Generalized inverse beamforming
The traditional beamforming method has the disadvantages of obvious sidelobe effect and low image resolution. For example, when it is used to identify the two real sound sources located on the quadrant bisector (as shown in Fig. 5), there are two false sound sources appear in the identification results, the identification accuracy often hard to meet the requirements of experiments.
Generalized inverse beamforming (GIB) has attracted much attention because of its accurate positioning and high computational efficiency [38]. By iteratively solving the sound source distribution, the algorithm requires less number of array elements, which reduces the amount of calculation and improves the identification accuracy. Through increasing the order of GIB, the beam sidelobe suppression ability can be doubled, so as to ensure that it has higher spatial resolution and more accurate sound source positioning ability [39]. This method was first proposed by Suzuki [40] to solve the problem of complex sound source identification. Its basic principle is to improve the spatial resolution of the identification results by using the inversion idea in beamforming theory. In the case of known microphone measurement vector p, the intensity vector q of sound source can be inversely calculated by the following formula:
Compared with Damas, the generalized inverse beamforming method has less operation difficulty and simple operation process, and can be used for the identification of different types of noise sources. In the actual measurement, due to the influence of environmental interference, there are usually errors in the data collected by the microphone, which directly affect the measurement results. As there may be no definite solution, non-unique definite solution or even no solution in cyclic iterative inversion, regularization specification is usually used to weaken the identification error of generalized inverse beamforming method. Presezniak [41], Gauthier [42], Zamponi [43], Xu [44], Li [45] and any other scholars have made a lot of research and solved some problems to improve the resolution of generalized inverse beamforming. At present, the spatial resolution of GIB in low-frequency sound band positioning needs to be further improved.
Fig. 5False sound source
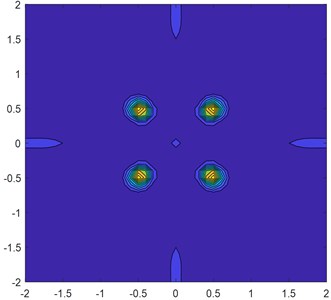
4. Acoustic holography
Based on the concept of optical holography, the hypothesis of acoustic holography was put forward in the 1960s, and the acoustic holography method was formed by constructing a general theoretical framework. The method uses the intensity and phase of sound source to identify the sound source, which has strong anti-interference ability and good robustness in complex acoustic measurement environment. Acoustic holography can be divided into conventional acoustic holography, near-field acoustic holography (NAH) and far-field acoustic holography, the sound pressure on the sound source surface can be reconstructed by the following formula:
when zs in the above formula is replaced by zc, the sound pressure at any position in the space can be reconstructed, the F represents the two-dimensional Fourier transform, F-1 represents the inverse two-dimensional Fourier transform; kx and ky are the wave numbers in x and y directions, respectively; ˜G-1 is the inverse of ˜GD.
Both acoustic holography and beamforming are noise source identification methods with microphone array as hardware equipment, but acoustic holography pays more attention to the phase and amplitude information of the sound wave emitted by the sound source. In NAH, sound pressure is measured by the holographic measuring surface surrounding the source, based on the spatial field transformation relationship between the source surface and the holographic surface, the sound field of the source surface is reconstructed by the sound pressure of the holographic surface. NAH measurement is close to the surface of sound source and retains evanescent wave components, so that the reconstruction accuracy of sound field greatly exceeds Rayleigh resolution criterion, which is incomparable to beamforming technology. The spatial transformation method of sound field signal is shown in Fig. 6.
Fig. 6The schematic diagram of space transformation of acoustic technology
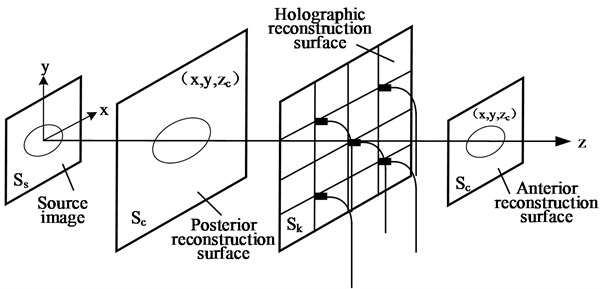
In the practical application of acoustic holography testing technology, we first need to assume two virtual planes: reconstruction plane and holographic plane (or sampling plane). The sound field information such as the amplitude and phase of the noise source is transmitted to the holographic plane through the air and collected by the microphone array. Then the sound field is reconstructed according to the information collected. NAH technology is especially suitable for the identification of low-frequency field source characteristics, the study of scatterer structure surface characteristics and structural modal vibration, as well as the prediction of source radiation power and far-field directivity of large structures. Komine [46] have used NAH to analyze aerodynamic noise. Yuan et al. [47] have developed a method for comprehensive testing and analysis technology, including reference microphone position selection, characteristic identification of incoherent source signals, and visualization of propagation paths of sound sources, contribution evaluation and output prediction of sound sources to system output. Experimental results show that this method is suitable for complex engineering practice.
Although the NAH technology has high reconstruction accuracy and good sound source identification effect, it usually needs to be used in anechoic pools or vast and quiet lakes to achieve good experimental results when it is used for underwater noise source identification [48]. Therefore, this method is greatly affected by environmental conditions. To solve this problem, a NAH noise source identification method is proposed which can be used in complex underwater acoustic field environment. Through experiments in anechoic pool, ideal lake and non-anechoic pool, similar experimental results were obtained, which overcomes the problem that NAH can’t be applied in complex underwater environment [48]. Wang [49] have combined with partial correlation analysis technology proposed an evaluation method for sound insulation performance of engine hood, which provides a guiding direction for improving comfort performance in passenger cars. Jia [50] have developed an acoustic holographic identification technology for fault diagnosis. Firstly, the external sound field of the identified object is reconstructed by microphone array. After obtaining the basic acoustic information, it is compared with the sound pressure and other data under the normal working state of the machine, and the error range is determined according to the sound pressure level, when the error between the test data and the normal data exceeds the predetermined error limit, the system determines it as a fault, so as to realize the fault diagnosis of mechanical equipment [51]. Nagamatsu [52] have found that the resolution of the reconstructed sound field was low when using the acoustic holography method to identify the sound source with a frequency lower than 100Hz. By studying the optimal identification distance and the most applicable sound source frequency band of the NAH algorithm, Wu improved the traditional acoustic holography method and proposed a double NAH method to improve the resolution, this method is better than the original method in identification efficiency and experimental economy. Thomas [53] proposed a wavelet preprocessing method, experimental results show that this method has good applicability, and an objective criterion is proposed to prove the feasibility of this method. Table 1 is a summary of the improvement methods proposed by the above researchers.
There are still some unsolved problems in the practical engineering application of acoustic holography technology. For example, the sound pressure at the holographic measurement point contains not only the sound pressure generated by the target sound source, but also the interference components generated by other background sound sources, so how to remove the influence of these background interference components. If this problem can be solved, the environmental requirements of acoustic holographic measurement technology will be greatly reduced, and it will play a positive role in solving the problem of industrial noise source positioning and identification and the promotion of NAH technology.
Table 1Several improvement methods and applications of NAH
Authors | Method | Contribution |
Yuan et al. [47] | A comprehensive testing-analysis technology | Evaluate and predict the contribution of system output |
Liu et al. [48] | A NAH noise source identification method | Solve the problem that NAH can’t be used in underwater environment |
Wang et al. [49] | A sound insulation performance evaluation method of engine hood | Improving comfort performance in passenger cars |
Jia et al. [50] | An acoustic holographic identification technology | Realize the fault diagnosis of mechanical equipment |
Nagamatsu et al. [52] | A double NAH method | Improve the resolution, identification efficiency and experiment economy |
Thomas et al. [53] | A wavelet preprocessing method | Lessen the effects due to the truncation of the hologram |
4.1. Equivalent source method
4.1.1. Wave superposition method
Wave superposition method was first proposed by Koopmann [54] as a robust algorithm in the full wave-number domain. It mainly studies modern image processing technology and visualization technology, and is used to analyze the radiation and scattering of sound (its principle is shown in Fig. 7), and overcomes two difficulties in solving the non-unique and singular integrals of the equation by the boundary element method based on the Helmholtz integral equation.
Wave superposition method considers that the sound field radiated from the surface of any shape object can be obtained by compounding the sound field generated by its internal alternative sound source [55], and then the research on the sound radiation on the surface of the object can be transformed into the research on the internal alternative sound source of the object, and the physical quantity close to the real sound field can be obtained through the virtual source. With the continuous improvement of wave superposition theory, its attention in the field of noise source identification is increasing [56]. Wu et al [57] have combined the wave superposition method with modern image recognition technology, and simulated the sound source of spherical pulsating signal by adding virtual damping in the virtual source intensity system, so as to propose a new method combining the wave superposition method. The experimental results show that the wave superposition method can be used to identify any shape of sound source, and the best sound pressure acquisition effect can be obtained when two linear microphone arrays are arranged at 90° crossover overlap. Song [58] and Fahnline [59] have calculated the error caused by the wave superposition method, which is used to analyze the acoustic radiation problem and the instability problem in the acoustic radiation calculation, respectively.
Fig. 7Schematic diagram of wave superposition sound field reconstruction
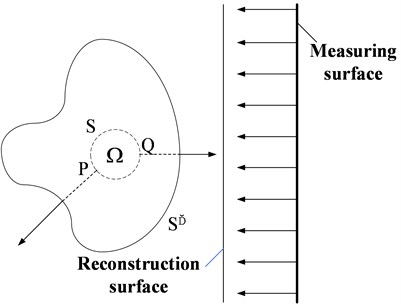
To realize the application of wave superposition method in dynamic sound source identification, Yang et al [60] have established the wave superposition relationship of moving sound field by using the linear Doppler frequency shift characteristics of short-time signal of moving sound source, and proposed a dynamic wave superposition method that can be applied to the field of moving sound source measurement. In this method, the sound source is predicted by beamforming, and then the dynamic superposition equation of sound source strength and sound pressure at the microphone is established based on the prediction results, so as to create the wave superposition method. This method does not need to change the number and array form of microphones. It can’t only ensure the economy of the experiment, but also reduce the difficulty of the experiment. It can obviously suppress the sidelobe effect in the process of sound source reconstruction and improve the problem of false sound sources. After combining the dipole sound source with the wave superposition theory, Song et al. [61] have studied the noise generated during the high-speed running of the train in the outdoor open field and the semi anechoic room respectively, and solved it by using the dual polar sound source of the least square method and genetic algorithm. The simulation experiment shows that this method has an ideal effect on the reconstruction of the sound field, the acoustic quantity decay equations of different trains at different speeds can be obtained, and the sound source position can be accurately analyzed. Aiming at the problem of false sound source in wave superposition, acoustic holography and beamforming, Li et al. [62] have proposed a wave superposition method based on dynamic optimization. This method uses acoustic array equipment to collect acoustic signals, estimates the sound pressure on the surface of the sound source object through the algorithm model, selects the maximum sound pressure point as the equivalent sound source, divides the sound source search area according to the position of the point, and takes the error between the prediction result and the real sound field as the algorithm evaluation index, the genetic algorithm is used for dynamic optimization to continuously reduce the error, realize the sound source reconstruction, and design the simulation experiment. The experimental results show that this technology can greatly weaken the false sound source and sidelobe effect.
4.1.2. Hels method
The implicit least square method (LSM) solves the problems of low efficiency of sound field reconstruction, non-unique equation solution and singular integral in the NAH method based on constant element and boundary element. LSM and wave superposition are numerical calculation methods developed from the idea of equivalent source, but their solutions are obviously different. Hels is a least square method based on Helmholtz equation. When this method was first proposed, it can only provide accurate solutions for the reconstruction points outside the smallest sphere covering irregular sound sources, but only approximate analysis for the inner region of the sphere. Subsequently, a large number of scholars have studied the orthogonal function adaptation approximation of hels. Dastour [63] have given a general construction method of the fourth-order finite difference scheme for Helmholtz equation based on point weight strategy. Cao et al [64] have proposed the generalized eigenvalue method and standard eigenvalue method for function approximation based on Chebyshev polynomials to solve the eigenvalues of helmholtz equation. The expansion term of the basis function has a direct influence on the results of sound field reconstruction, so the reasonable selection of the number of measurement points and expansion terms is particularly critical. Dwarka [65] and Macklin [66] have combined geometric analysis from the perspective of scalability, analyzed the sound field in the smallest sphere covering irregular sound source with trigonometric function and spherical function as basis function, and obtained the scalable convergence of hels.
4.2. Static sound source identification
Theoretically, far-field acoustic holography can calculate acoustic quantities at any position outside the radiation source, while its resolution is limited by half wavelength (λ/2), it is only sensitive to the propagating wave components of low and medium frequency in space, and it is easy to ignore the evanescent wave components with high frequency information. Far field acoustic holography is often used to identify the sound source of large radiation sources such as trains or difficult to access sound generators. Some scholars have applied the method to calculate the distribution of noise sources. Aiming at the problem of low recognition accuracy due to aperture limitation of acoustic holography, an optimization scheme of microphone array and recognition algorithm has been proposed. Lu [67] has introduced grey relational clustering technology on the basis of traditional acoustic fault diagnosis to remove the extracted redundant sound source information. The optimized diagnosis technology has significantly improved the identification efficiency and algorithm robustness. On this basis, he has developed a high identification rate method for weak fault conditions of three-dimensional space sound field. After comparing the actual performance of several vehicle noise source identification methods, zhao et al. [68] have optimized the algorithm according to the problems existing in the experimental data. The optimized experimental results have significantly improved the performance compared with the original reconstruction algorithm.
At present, it is urgent to integrate the idea of sound field separation with the theory of NAH and use it in this field. Mou et al. [69] have combined the idea of sound field separation with the theory of NAH, studied the near-field measurement technology under complex boundary conditions by means of sound pressure measurement, and designed an application system for this field. The sound field separation technology proposed by Deng et al [70] can solve the problem of poor effect of traditional NAH in internal sound source identification. As NAH has the widest application range, the highest resolution of experimental results and the strongest practicability, this technology has become mature and is widely used to identify medium and low frequency noise sources in close range.
4.3. Moving sound source identification
According to the Doppler effect, when the sound source and the measuring point move relative to each other, if the sound source is close to the measuring point, the frequency received by the microphone will be greater than the actual frequency of the sound source; if the sound source is far away from the measuring point, the frequency received by the microphone will be less than the actual frequency of the sound source. Consequently, traditional measurement methods such as sound pressure measurement, sound intensity measurement and coherence function method can only identify the noise source in the static state, but cannot give the measurement results for the moving sound source.
As shown in Fig. 8, a sound source with radiation frequency f moves from point A to point B with a constant speed υ at the initial moment, the measurement point is located at point P. After time t1, the sound signal reaches point B, and at the same time, the sound wave with phase φ is radiated, and α is the included angle between BP and the motion direction. After time t2, the sound wave front of this phase reaches the sound receiving point P, the propagation distance is the length R(t) of BP. Then, the frequency fm0 of the sound wave with phase φ radiated from the sound source at time t1 received by the sound receiving point P at time t is:
in which, M is the ratio of the sound velocity to υ.
Fig. 8Schematic diagram of Doppler effect
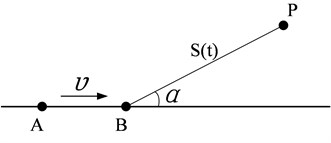
Sarkissian et al. [71] have tried to measure and analyze the noise of moving vehicles by using sound source identification technologies such as microphone array measurement and holographic measurement, but the results of sound field reconstruction and sound source identification are not accurate due to the influence of Doppler-effect. Starting from the Doppler phenomenon of wave, Yang et al. [72] have proposed a theoretical method to eliminate Doppler effect by designing a data acquisition and measurement device and using acoustic holography to deal with motion noise. This method can accurately establish the sound field distribution model of the object surface under the background of the noise generated by the high-speed motion of the object, and provide a new basis and solution for the analysis and improvement of the motion noise. This method can eliminate the Doppler-effect in the frequency domain and locate the sound source in the process of object movement, but it can’t carry out quantitative analysis of sound source. Yang et al. [73] have used the theory of NAH to study the identification of moving sound source again, realized the reconstruction of holographic surface, and eliminated the Doppler-effect in time domain. The new method has higher calculation accuracy and identification efficiency, and improves the disadvantages of over reliance on sound source frequency information in the preliminary work but can only be used for the analysis of single frequency sound sources. However, there is still no effective solution to the problems of sidelobe effect and false sound sources. Some scholars use wavelet transform to analyze the Doppler-effect in the process of moving sound source identification, and put forward corresponding solutions, which breaks the limitation that the identification method needs to predict the real frequency of sound source to eliminate the Doppler-effect, and provides a new idea for solving this kind of problem [74]. Such as Miao et al. [75] put forward a new solution to solve the problem of moving sound source, which can more accurately determine the position and sound pressure of moving sound source. Therefore, this method can also get rid of the limitations of sound source frequency and number, and have no longer need to eliminate Doppler-effect one by one for different frequencies and different sound sources. Vehicle noise measurement experiments show that the acoustic holography reconstruction method of moving sound source can accurately reconstruct the sound field of high-speed moving sound source with different frequency components, which provides a valuable basis for its practical application.
5. Hybrid acoustic holography
Sound source identification methods such as beamforming, acoustic holography and wave superposition have irreplaceable advantages in their respective application fields, but they also have the problems of single function and relatively poor robustness. When the sound field environment changes or the accuracy of sound source identification is required, these methods cannot get satisfactory results. To improve the generality and robustness of sound source identification algorithm, the scholars combine various traditional sound source identification methods and propose a new sound source identification method: hybrid acoustic holography sound source identification method. The principle of hybrid acoustic holography is shown in Fig. 9, select a plane perpendicular to the direction of measurement and larger than the cross section of the object, and a small distance is shifted inward as the equivalent source plane S. The curve represents the projection of the object on the plane S. The grids are evenly divided on plane S (it is appropriate to choose the grid spacing as half of the wavelength corresponding to the highest analysis frequency), and then a candidate equivalent source is configured in each grid, such as P. Because the sound source identified by beamforming is located within the curve, this configuration can not only satisfy the principle of wave superposition method, but also improve the computational efficiency of beamforming.
Fig. 9Schematic diagram of sound pressure structure of holographic surface
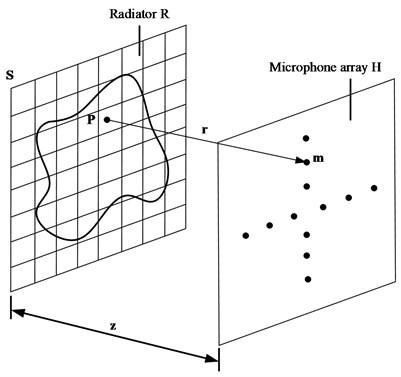
To obtain the free field information, firstly, the reflected field and the sound field synthesized by the free field can be separated, then the noise source can be identified by wave superposition, an algorithm of hybrid wave superposition and holography is obtained. It is easy to operate and practical, and can be used to identify noise sources of arbitrary shapes under specific sound fields [76], and this algorithm can also be used to solve the problem of interface reflection interference in underwater sound source identification. Wang et al. [76] have proposed a combined algorithm of wave superposition and beamforming, which can reconstruct the sound field of noise source with only a few microphones. Zhuo et al. [77] have proposed a combined sound source identification method for three-dimensional positioning of noise sources. Based on microphone array technology, this method draws the distribution map of sound sources on two-dimensional plane, takes the results shown in the map as the coordinates of equivalent sound sources, and solves the spatial coordinates of real sound sources through genetic algorithm, which can better weaken the sidelobe effect and false sound sources. Zheng et al. [78] have proposed a wave superposition hybrid acoustic holography identification algorithm to solve the problems of sidelobe effect and false sound source. Firstly, the maximum sound pressure identified by the beamforming method is taken as the location of the ideal sound source; then the sound intensity is used to solve the equations listed based on the wave superposition theory; and the solution results are used to construct the acoustic holographic surface; finally the sound pressure of the reconstructed surface is calculated according to the results of the holographic surface. The simulation results show that the sound source identification based on the algorithm obviously suppresses the factors interfering with the experimental results such as sidelobe effect.
6. Conclusions
In this paper, a comprehensive review of noise source identification methods (beamforming and NAH methods) is developed to summarize its developing status. Firstly, the advantages and disadvantages of existing beamforming and NAH algorithms are summarized, and the application fields of these methods are discussed. In addition, to solve the problems of beamforming and NAH methods with single function and poor robustness (difficult to balance the accuracy of source identification and sound field reconstruction), hybrid acoustic holography method has been proposed and developed by a large number of scholars, this paper reviews and discusses them which can provide a reference for the research and development of sound source identification.
At present, the sound source identification method based on microphone array has made abundant achievements, but there are still some problems that have not been completely solved. For example, the positioning accuracy of the existing technology under strong reverberation and low SNR needs to be improved; neural network, genetic algorithm and other advanced optimization algorithms can be applied to optimize the structure parameters and weight function of microphone array; the size and shape of microphone array will greatly affect the accuracy of location, by changing the geometric parameters of array and optimizing the arrangement of array microphone, a more reasonable weight coefficient of microphone signal can be sought to improve the resolution of sound source identification.
References
-
B. Mishachandar and S. Vairamuthu, “Diverse ocean noise classification using deep learning,” Applied Acoustics, Vol. 181, p. 108141, Oct. 2021, https://doi.org/10.1016/j.apacoust.2021.108141
-
D. Czopek, D. Gryboś, J. Leszczyński, and J. Wiciak, “Identification of energy wastes through sound analysis in compressed air systems,” Energy, Vol. 239, p. 122122, Jan. 2022, https://doi.org/10.1016/j.energy.2021.122122
-
P. Saha, S. Dash, and S. Mukhopadhyay, “Physics-incorporated convolutional recurrent neural networks for source identification and forecasting of dynamical systems,” Neural Networks, Vol. 144, pp. 359–371, Dec. 2021, https://doi.org/10.1016/j.neunet.2021.08.033
-
S. Kita and Y. Kajikawa, “Fundamental study on sound source localization inside a structure using a deep neural network and computer-aided engineering,” Journal of Sound and Vibration, Vol. 513, p. 116400, Nov. 2021, https://doi.org/10.1016/j.jsv.2021.116400
-
S. Y. Lee, J. Chang, and S. Lee, “Deep learning-based method for multiple sound source localization with high resolution and accuracy,” Mechanical Systems and Signal Processing, Vol. 161, p. 107959, Dec. 2021, https://doi.org/10.1016/j.ymssp.2021.107959
-
L. Liang, S. Chen, and P. Li, “The evaluation of vehicle interior impact noise inducing by speed bumps based on multi-features combination and support vector machine,” Applied Acoustics, Vol. 163, p. 107212, Jun. 2020, https://doi.org/10.1016/j.apacoust.2020.107212
-
B. Barsikow and W. F. King, “On removing the Doppler frequency shift from array measurements of railway noise,” Journal of Sound and Vibration, Vol. 120, No. 1, pp. 190–196, Jan. 1988, https://doi.org/10.1016/0022-460x(88)90344-6
-
K. Chen, M. Li, and C. Li, “Application of coherence analysis study on identification of vehicle noise sources,” Journal of Vibroengineering, Vol. 20, No. 7, pp. 2745–2757, Nov. 2018, https://doi.org/10.21595/jve.2018.19317
-
H. Wu, X. Wei, Y. Zha, and W. Jiang, “Acoustic spatial patterns recognition based on convolutional neural network and acoustic visualization,” The Journal of the Acoustical Society of America, Vol. 147, No. 1, pp. 459–468, Jan. 2020, https://doi.org/10.1121/10.0000618
-
X. Zhao, B. Liu, and H. Shi, “Sound source localization method by using a single moving microphone and inner product calculation,” Applied Acoustics, Vol. 177, No. 4, p. 107919, Jun. 2021, https://doi.org/10.1016/j.apacoust.2021.107919
-
J. Heymann, L. Drude, and R. Haeb-Umbach, “A generic neural acoustic beamforming architecture for robust multi-channel speech processing,” Computer Speech and Language, Vol. 46, pp. 374–385, Nov. 2017, https://doi.org/10.1016/j.csl.2016.11.007
-
M. Snellen, T. C. Gaida, L. Koop, E. Alevizos, and D. G. Simons, “Performance of multibeam echosounder backscatter-based classification for monitoring sediment distributions using multitemporal large-scale ocean data sets,” IEEE Journal of Oceanic Engineering, Vol. 44, No. 1, pp. 142–155, Jan. 2019, https://doi.org/10.1109/joe.2018.2791878
-
L. Chen and S. F. Wu, “A modified Helmholtz equation least squares method for reconstructing vibroacoustic quantities on an arbitrarily shaped vibrating structure,” Journal of Theoretical and Computational Acoustics, Vol. 29, No. 3, p. 21500, Sep. 2021, https://doi.org/10.1142/s2591728521500067
-
H. Wu, D. Li, L. Yu, and W. Jiang, “A boundary element method based near field acoustic holography in noisy environments,” The Journal of the Acoustical Society of America, Vol. 147, No. 5, pp. 3360–3371, May 2020, https://doi.org/10.1121/10.0001225
-
Y. J. R. Gounot and R. E. Musafir, “Simulation of scattered fields: Some guidelines for the equivalent source method,” Journal of Sound and Vibration, Vol. 330, No. 15, pp. 3698–3709, Jul. 2011, https://doi.org/10.1016/j.jsv.2011.03.006
-
W. F. King and D. Bechert, “On the sources of wayside noise generated by high-speed trains,” Journal of Sound and Vibration, Vol. 66, No. 3, pp. 311–332, Oct. 1979, https://doi.org/10.1016/0022-460x(79)90848-4
-
J. Li, Z. Lv, Z. Hou, and Y. Pei, “Comparison of balanced direct search and iterative angular spectrum approaches for designing acoustic holography structure,” Applied Acoustics, Vol. 175, No. 3, p. 107848, Apr. 2021, https://doi.org/10.1016/j.apacoust.2020.107848
-
J. Wang, Z. Zhang, Y. Huang, Z. Li, and Q. Huang, “A 3D convolutional neural network based near-field acoustical holography method with sparse sampling rate on measuring surface,” Measurement, Vol. 177, p. 109297, Jun. 2021, https://doi.org/10.1016/j.measurement.2021.109297
-
J. Hou, W. Jiang, and W. Lu, “Application of a near-field acoustic holography-based diagnosis technique in gearbox fault diagnosis,” Journal of Vibration and Control, Vol. 19, No. 1, pp. 3–13, Jan. 2013, https://doi.org/10.1177/1077546311428634
-
H. Kook, G. B. Moebs, P. Davies, and J. S. Bolton, “An efficient procedure for visualizing the sound field radiated by vehicles during standardized Pass by tests,” Journal of Sound and Vibration, Vol. 233, No. 1, pp. 137–156, May 2000, https://doi.org/10.1006/jsvi.1999.2794
-
L. Bertsch, F. Wolters, W. Heinze, M. Pott-Pollenske, and J. Blinstrub, “System noise assessment of a tube-and-wing aircraft with geared turbofan engines,” Journal of Aircraft, Vol. 56, No. 4, pp. 1577–1596, Jul. 2019, https://doi.org/10.2514/1.c034935
-
J. Hald, “A comparison of compressive equivalent source methods for distributed sources,” The Journal of the Acoustical Society of America, Vol. 147, No. 4, pp. 2211–2221, Apr. 2020, https://doi.org/10.1121/10.0001073
-
J. Hald, “A comparison of iterative sparse equivalent source methods for near-field acoustical holography,” The Journal of the Acoustical Society of America, Vol. 143, No. 6, pp. 3758–3769, Jun. 2018, https://doi.org/10.1121/1.5042223
-
F. A. Dobrowohl, A. J. Milne, and R. T. Dean, “Controlling perception thresholds for changing timbres in continuous sounds,” Organised Sound, Vol. 24, No. 1, pp. 71–84, Apr. 2019, https://doi.org/10.1017/s1355771819000074
-
P. N. Shah, H. Vold, D. Hensley, E. Envia, and D. Stephens, “A high-resolution continuous-scan acoustic measurement method for turbofan engine applications,” Journal of Turbomachinery, Vol. 137, No. 12, p. 12100, Dec. 2015, https://doi.org/10.1115/1.4031341
-
H. Woodsum and C. Woodsum, “Optimized hermetic transform beam-forming of acoustic arrays via cascaded spatial filter arrangements derived using a chimerical evolutionary genetic algorithm,” ICA 2013 Montreal, Vol. 19, No. 1, p. 055040, 2013, https://doi.org/10.1121/1.4800596
-
C. Chesnais, N. Totaro, J.-H. Thomas, and J.-L. Guyader, “Reconstruction and separation of vibratory field using structural holography,” Journal of Sound and Vibration, Vol. 389, pp. 134–152, Feb. 2017, https://doi.org/10.1016/j.jsv.2016.10.042
-
G. Cirrincione, R. R. Kumar, A. Mohammadi, S. H. Kia, P. Barbiero, and J. Ferretti, “Shallow versus deep neural networks in gear fault diagnosis,” IEEE Transactions on Energy Conversion, Vol. 35, No. 3, pp. 1338–1347, Sep. 2020, https://doi.org/10.1109/tec.2020.2978155
-
Z. Zhang, S. Chen, Z. Xu, Y. He, and S. Li, “Iterative regularization method in generalized inverse beamforming,” Journal of Sound and Vibration, Vol. 396, pp. 108–121, May 2017, https://doi.org/10.1016/j.jsv.2017.02.044
-
E. J. G. Arcondoulis and Y. Liu, “Acoustic beamforming array design methods over irregular shaped areas,” Journal of Vibration and Acoustics, Vol. 143, No. 3, p. 03101, Jun. 2021, https://doi.org/10.1115/1.4049943
-
J. Zhang, G.-X. Han, X.-B. Xiao, R.-Q. Wang, Y. Zhao, and X.-S. Jin, “Influence of wheel polygonal wear on interior noise of high-speed trains,” China’s High-Speed Rail Technology, Vol. 15, No. 12, pp. 373–401, 2018, https://doi.org/10.1007/978-981-10-5610-9_20
-
Rakesh. C. et al., “Noise source localization on a small wind turbine using a compact microphone array with advanced beamforming algorithms, part I-A study of aerodynamic noise from blades,” Wind Engineering, Vol. 38, No. 1, pp. 73–88, 2014.
-
S. Buck, S. Oerlemans, and S. Palo, “Experimental characterization of turbulent inflow noise on a full-scale wind turbine,” Journal of Sound and Vibration, Vol. 385, pp. 219–238, Dec. 2016, https://doi.org/10.1016/j.jsv.2016.09.010
-
D. Sun, C. Ma, J. Mei, and W. Shi, “Improving the resolution of underwater acoustic image measurement by deconvolution,” Applied Acoustics, Vol. 165, p. 107292, Aug. 2020, https://doi.org/10.1016/j.apacoust.2020.107292
-
D. Guo, Y. Zhou, Y. Zhou, Y. Wang, F. Chen, and X. Shi, “Numerical and experimental study of gear rattle based on a refined dynamic model,” Applied Acoustics, Vol. 185, No. 2, p. 108407, Jan. 2022, https://doi.org/10.1016/j.apacoust.2021.108407
-
Zhang. Z. et al., “Design of directional sound-capture system based on MEMS microphone array,” Communications Technology, Vol. 51, No. 9, pp. 2241–2246, 2018.
-
P. J. Jackson, F. Jacobsen, P. D. Coleman, and J. Abildgaard Pedersen, “Sound field planarity characterized by superdirective beamforming,” The Journal of the Acoustical Society of America, Vol. 133, No. 5, pp. 3344–3344, May 2013, https://doi.org/10.1121/1.4805659
-
Li. S. et al., “Sound source identification based on functional generalized inverse beamforming,” Journal of Mechanical Engineering, Vol. 52, No. 4, pp. 1–6, 2016.
-
Chen. S. et al., “Modified algorithm for generalized inverse beamforming based on high-order matrix function,” Journal of Vibration and Shock, Vol. 36, No. 10, pp. 98–103, 2017.
-
T. Suzuki, “L1 generalized inverse beam-forming algorithm resolving coherent/incoherent, distributed and multipole sources,” Journal of Sound and Vibration, Vol. 330, No. 24, pp. 5835–5851, Nov. 2011, https://doi.org/10.1016/j.jsv.2011.05.021
-
N. Quaegebeur, T. Padois, P.-A. Gauthier, and P. Masson, “Enhancement of time-domain acoustic imaging based on generalized cross-correlation and spatial weighting,” Mechanical Systems and Signal Processing, Vol. 75, pp. 515–524, Jun. 2016, https://doi.org/10.1016/j.ymssp.2015.12.012
-
A. Pereira, J. Antoni, and Q. Leclère, “Empirical Bayesian regularization of the inverse acoustic problem,” Applied Acoustics, Vol. 97, pp. 11–29, Oct. 2015, https://doi.org/10.1016/j.apacoust.2015.03.008
-
R. Zamponi, P. Chiariotti, G. Battista, C. Schram, and P. Castellini, “3D Generalized inverse beamforming in wind tunnel aeroacoustic testing: application to a counter rotating open rotor aircraft model,” Applied Acoustics, Vol. 163, p. 107229, Jun. 2020, https://doi.org/10.1016/j.apacoust.2020.107229
-
Xu. Z. et al., “Smoothed L0 norm generalized inverse beamforming,” Chinese Journal of Scientific Instrument, Vol. 35, No. 6, pp. 1276–1281, 2014.
-
Li. S. et al., “Generalized inverse beamforming via elastic net regularization,” Chinese Journal of Scientific Instrument, Vol. 36, No. 5, pp. 1170–1176, 2015.
-
A. T. Wall, K. L. Gee, T. B. Neilsen, R. L. Mckinley, and M. M. James, “Military jet noise source imaging using multisource statistically optimized near-field acoustical holography,” The Journal of the Acoustical Society of America, Vol. 139, No. 4, pp. 1938–1950, Apr. 2016, https://doi.org/10.1121/1.4945719
-
G.-Q. Yuan, W.-K. Jiang, and H.-X. Hua, “Hydroacoustic analysis of open cavity subsonic flow based on multiple parameter numerical models,” Journal of Hydrodynamics, Vol. 27, No. 5, pp. 668–678, Oct. 2015, https://doi.org/10.1016/s1001-6058(15)60529-7
-
X. Liu, J. Fan, C. Sun, Y. Yang, and J. Zhuo, “High-resolution and low-sidelobe forward-look sonar imaging using deconvolution,” Applied Acoustics, Vol. 178, No. 12, p. 107986, Jul. 2021, https://doi.org/10.1016/j.apacoust.2021.107986
-
Wang. Q. et al., “Application of partial coherence analysis in interior noise reduction of passenger cars,” Noise and Vibration Control, Vol. 37, No. 3, pp. 112–116, 2017.
-
W. Jia, J. Chen, C. Yang, and J. Li, “Study of sound source identification based on beamforming and patch near-field acoustic holography,” Journal of Computational Acoustics, Vol. 17, No. 3, pp. 219–245, Sep. 2009, https://doi.org/10.1142/s0218396x09003951
-
Peng Chen, Xiang Tian, and Yaowu Chen, “Optimization of the digital near-field beamforming for underwater 3-D sonar imaging system,” IEEE Transactions on Instrumentation and Measurement, Vol. 59, No. 2, pp. 415–424, Feb. 2010, https://doi.org/10.1109/tim.2009.2024368
-
Masao et al., “A study of subsidiary source image reduction in acoustic double holography method,” Transactions of the Japan Society of Mechanical Engineers Series C, Vol. 601, No. 62, 1996.
-
Thomas. J. and Pascal. J., “Wavelet preprocessing for lessening truncation effects in nearfield acoustical holography,” Journal of the Acoustical Society of America, Vol. 118, No. 2, pp. 851–860, 2005.
-
G. H. Koopmann, L. Song, and J. B. Fahnline, “A method for computing acoustic fields based on the principle of wave superposition,” The Journal of the Acoustical Society of America, Vol. 86, No. 6, pp. 2433–2438, Dec. 1989, https://doi.org/10.1121/1.398450
-
L. Geng, X.-Z. Zhang, and C.-X. Bi, “Reconstruction of transient vibration and sound radiation of an impacted plate using time domain plane wave superposition method,” Journal of Sound and Vibration, Vol. 344, pp. 114–125, May 2015, https://doi.org/10.1016/j.jsv.2015.01.046
-
Chen. H. et al., “Sound radiation prediction for underwater structure by field-matching wave superposition method,” Acta Acustica, Vol. 38, No. 2, pp. 137–146, 2013.
-
Wu. S., Xiang. Y., and Li. S., “Position optimization of particular solution sources for distributed source boundary point method by volume velocity-matching,” Chinese Journal of Acoustics, Vol. 34, No. 2, pp. 123–137, 2015.
-
Z. Wang and S. F. Wu, “Helmholtz equation-least-squares method for reconstructing the acoustic pressure field,” The Journal of the Acoustical Society of America, Vol. 102, No. 4, pp. 2020–2032, Oct. 1997, https://doi.org/10.1121/1.419691
-
J. B. Fahnline and G. H. Koopmann, “A numerical solution for the general radiation problem based on the combined methods of superposition and singular‐value decomposition,” The Journal of the Acoustical Society of America, Vol. 90, No. 5, pp. 2808–2819, Nov. 1991, https://doi.org/10.1121/1.401878
-
F. Miao, D. Yang, J. Wen, and X. Lian, “Moving sound source localization based on triangulation method,” Journal of Sound and Vibration, Vol. 385, pp. 93–103, Dec. 2016, https://doi.org/10.1016/j.jsv.2016.09.001
-
L. Song, H. Chen, and B. Li, “Aerodynamic noise separation of an EMU trailer bogie area using train operation tests,” Shock and Vibration, Vol. 2018, No. 12, pp. 1–10, Dec. 2018, https://doi.org/10.1155/2018/7941980
-
Keqiang Li, Sifa Zheng, Diange Yang, Xiaomin Lian, and Masao Nagai, “Modeling and control of active suspensions for MDOF vehicle,” Tsinghua Science and Technology, Vol. 8, No. 2, pp. 224–230, Apr. 2003.
-
H. Dastour and W. Liao, “A generalized optimal fourth-order finite difference scheme for a 2D Helmholtz equation with the perfectly matched layer boundary condition,” Journal of Computational and Applied Mathematics, Vol. 394, p. 113544, Oct. 2021, https://doi.org/10.1016/j.cam.2021.113544
-
L. Cao, Y. Gu, C. Zhang, and Q.-H. Qin, “A meshless Chebyshev collocation method for eigenvalue problems of the Helmholtz equation,” Engineering Analysis with Boundary Elements, Vol. 125, pp. 80–109, Apr. 2021, https://doi.org/10.1016/j.enganabound.2021.01.006
-
V. Dwarka, R. Tielen, M. Möller, and C. Vuik, “Towards accuracy and scalability: combining isogeometric Analysis with deflation to obtain scalable convergence for the Helmholtz equation,” Computer Methods in Applied Mechanics and Engineering, Vol. 377, p. 113694, Apr. 2021, https://doi.org/10.1016/j.cma.2021.113694
-
M. Macklin, K. Erleben, M. Müller, N. Chentanez, S. Jeschke, and T.-Y. Kim, “Primal/Dual descent methods for dynamics,” Computer Graphics Forum, Vol. 39, No. 8, pp. 89–100, 2020, https://doi.org/10.1111/cgf14104
-
W. Lu, W. Jiang, H. Wu, and J. Hou, “A fault diagnosis scheme of rolling element bearing based on near-field acoustic holography and gray level co-occurrence matrix,” Journal of Sound and Vibration, Vol. 331, No. 15, pp. 3663–3674, Jul. 2012, https://doi.org/10.1016/j.jsv.2012.03.008
-
S. Zhao, Z. Chu, Y. Yang, and Y. Zhang, “High-resolution CLEAN-SC for acoustic source identification with spherical microphone arrays,” The Journal of the Acoustical Society of America, Vol. 145, No. 6, pp. EL598–EL603, Jun. 2019, https://doi.org/10.1121/1.5113972
-
Mou. J. et al., “Analysis on the characteristics of UNDEX bubbles under different bound conditions,” Journal of Vibration and Shock, Vol. 33, No. 13, pp. 92–97, 2014.
-
Lu. X. et al., “Analysis and Optimization of vehicle vibration and noise caused by powertrain excitation,” Automobile Applied Technology, Vol. 2, pp. 5–7, 2016.
-
A. Sarkissian, “Extension of measurement surface in near-field acoustic holography,” The Journal of the Acoustical Society of America, Vol. 115, No. 4, pp. 1593–1596, Apr. 2004, https://doi.org/10.1121/1.1645609
-
D. Yang, J. Wen, F. Miao, Z. Wang, X. Gu, and X. Lian, “Complete de-dopplerization and acoustic holography for external noise of a high-speed train,” The Journal of the Acoustical Society of America, Vol. 140, No. 3, pp. 1739–1748, Sep. 2016, https://doi.org/10.1121/1.4962554
-
D. Yang, Z. Wang, B. Li, Y. Luo, and X. Lian, “Quantitative measurement of pass-by noise radiated by vehicles running at high speeds,” Journal of Sound and Vibration, Vol. 330, No. 7, pp. 1352–1364, Mar. 2011, https://doi.org/10.1016/j.jsv.2010.10.001
-
S. Ungnad, D. Sachau, M. Wandel, and C. Thomas, “Experimental noise source identification in a fuselage test environment based on nearfield acoustical holography,” CEAS Aeronautical Journal, Vol. 12, No. 4, pp. 793–802, Nov. 2021, https://doi.org/10.1007/s13272-021-00534-6
-
H. Wu, W. Jiang, and H. Zhang, “A mapping relationship based near-field acoustic holography with spherical fundamental solutions for Helmholtz equation,” Journal of Sound and Vibration, Vol. 373, pp. 66–88, Jul. 2016, https://doi.org/10.1016/j.jsv.2016.03.010
-
Wang. R. et al., “Acoustic field separation technique with single holographic plane based wave superposition algorithm and statistically optimal near-field acoustical holography,” Journal of Vibration and Shock, Vol. 31, No. 22, pp. 112–117, 2012.
-
Zhuo. R. et al., “Research on noise source 3-D localization based on beam-forming and wave superposition method,” Automotive Engineering, Vol. 41, No. 10, pp. 1210–1214, 2019.
-
S. Zheng, J. Dan, P. Hao, and X. Lian, “Decomposition and visualization of vehicle noise sources with transfer path acoustical holography,” Noise Control Engineering Journal, Vol. 62, No. 5, pp. 313–321, Sep. 2014, https://doi.org/10.3397/1/376230