Abstract
Currently, aerodynamic environment prediction research into scramjet-propelled vehicles characterized by NACA0012 under hypersonic conditions is relatively sparse. Two-dimensional external flow field models are established, and then through validation tests, we perform a systematic investigation between simulation parameters and prediction accuracy, and an effective aerodynamic environment prediction simulation scheme under hypersonic conditions is proposed. Unlike under incompressible conditions, the maximum accuracy decline could be attributed to the inappropriate choice of the sharp trailing edge modeling method, but the definition formula is still preferred. In particular, for the two modeling data point sources, Airfoil tools and NACA4, the numerical performance of the latter is better than the former, and the calculation accuracy negatively correlates with the number of data points offered by both of them. Moreover, for the mesh cells near the shock, the cell Reynolds number and aspect ratio values should be no smaller than 16 and not exceed 380, respectively, and the recommended values for the far field distance, the turbulence model and flux type are 16L, Spalart-Allmaras, and ROE flux type. Under hypersonic conditions, the aerodynamic environment characterized by NACA0012 predicts a maximum temperature of approximately 1856.85 °C, with an average temperature change rate of 77 °C/s. Meanwhile, the top sound pressure level and the vibration acceleration could reach up to 145 dB and 182 g, respectively.
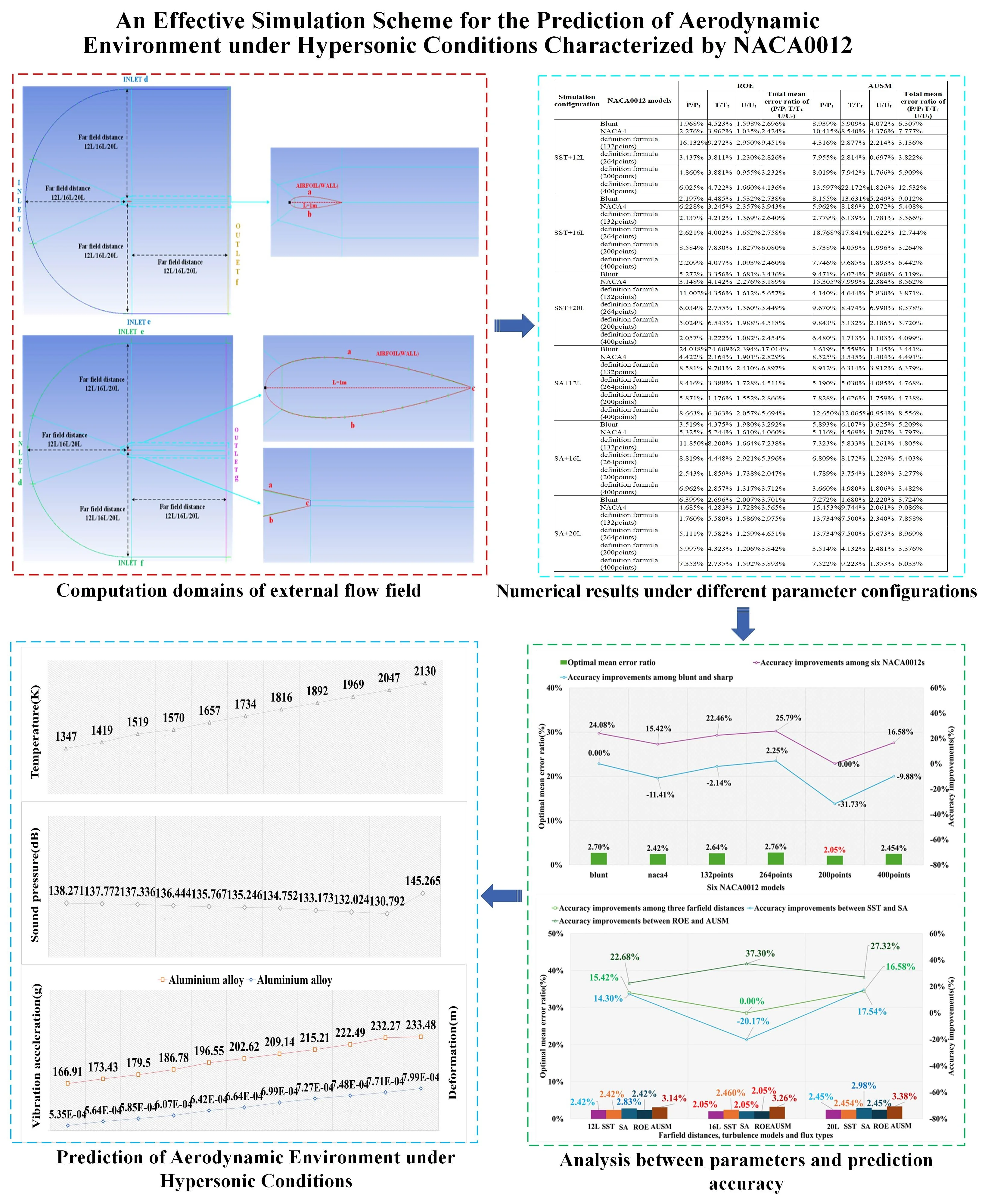
Highlights
- The suitable values of cell Reynolds number and aspect ratio for NACA0012 differ from those for a wedge section.
- The proper simulation parameters for NACA0012 at hypersonic speed differs from that at a low speed.
- The sharp trailing edge is preferred.
- The utilization of advanced materials plays a pivotal role in enhancing structural reliability.
1. Introduction
Adapting to the environment becomes crucial as the hypersonic vehicle encounters increasingly harsh flight conditions. NASA has identified environmental failure as the leading cause of previous vehicle launch crashes, emphasizing the criticality of environment testing. Therefore, it is imperative to first predict the aerodynamic environment experienced by the hypersonic vehicle and subsequently design its structure accordingly. By conducting comprehensive environment testing, potential issues can be identified and addressed to enhance flights’ reliability and adaptability in diverse environments. The predicted results of the aerodynamic environment serve as a fundamental basis for both vehicle design and subsequent environment testing. The accurate prediction of the aerodynamic environment is crucial for the design and optimization of the hypersonic vehicle. The main types of hypersonic vehicle include the ground-to-orbit reentry, the low-orbit reentry and the scramjet-propelled [1] and the reusable reentry vehicle is the leading research object [2]-[7], while the prediction research for the scramjet-propelled vehicle is relatively sparse. Hence, we focus our research on the scramjet-propelled hypersonic vehicle.
Analyzing the flight trajectory and determining the initial atmospheric conditions is crucial for accurate environmental forecasting. As shown in Fig. 1 [8], the scramjet-propelled vehicle is transported by a large aircraft carrier to the designated location. Subsequently, the solid rocket engine propels the vehicle to achieve Mach 0.8 into the climbing phase, which encompasses the ejection, the sub-combustion, and the super-combustion phases, responsible for rapidly increasing the flight altitude and speed and lasts about 34 seconds. Once the vehicle attains the desired altitude and Mach number (30 km and 6.5), it transitions into the cruise phase. Throughout this period, the vehicle will maintain a consistent altitude and velocity, constituting approximately 90 % of its total range. After reaching the designated airspace, it would proceed to initiate the attack phase with precision and accuracy against the intended target. According to Ref. [9], the external flow field could be categorized as either incompressible or compressible based on its velocity. Furthermore, the compressible flow is considered transonic when the Mach number ranges from 0.6 to 1. The flow field is referred to as supersonic when the Mach number falls between 1 and 3, while it is categorized as high supersonic when the Mach number ranges from 3 to 5. Finally, if the Mach number exceeds 5, the flow field is classified as hypersonic. The flight path of the scramjet-propelled vehicle typically encompasses all the aforementioned compressible conditions. Confirmation of specific environmental prediction parameters is required for different flow conditions. Therefore, it is imperative to analyze the simulation parameters' effect on calculation accuracy to ascertain the optimal simulation configuration. Based on the determined optimal simulation configurations, we could more accurately forecast the aerodynamic conditions of the characteristic position of the scramjet-propelled vehicle during flight, enabling enhanced vehicle optimization and thermal protection design.
Fig. 1The typical flight trajectory of the scramjet-propelled hypersonic vehicle
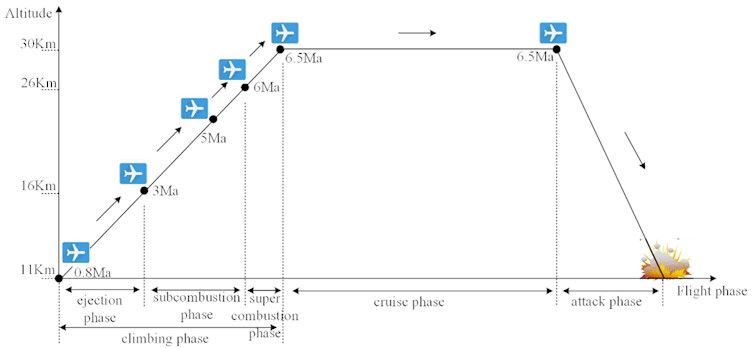
The NACA0012 airfoil is widely employed for investigating the characteristics of the aerodynamic environment [10]-[14], and the analysis of Refs. [15], [16] reveals a significant correlation between numerical accuracy and the trailing edge shape. NACA0012 has sharp and blunt two trailing edge shapes. For the sharp trailing edge shape, Refs. [17], [18] discusses the external flow field properties of dimpled and square dimpled NACA0012s. The studied Mach numbers are 1.7, 2.2, and 2.7. SST k-omega and Spalart-Allmaras (SA) turbulence models are used. The results show there is a positive correlation between Mach number and aerodynamic condition. Based on different ranges of upper and lower surface temperatures, Ref. [19] extends the previous investigations, and the force coefficients are evaluated. Under the conditions of pitching and plunging NACA0012s, Ref. [20] uses SST k-omega and SA at very low speed to study the thermal effect on force coefficients around NACA0012. The conclusion indicates that the lift coefficient is increased, and the drag coefficient is decreased due to the temperature variation between extrados and intrados of the airfoil. Similar research is investigated by Ref. [21], and a spectral analysis demonstrates that as the surface temperature increases, the force coefficient amplitudes decrease. Based on the 0.3 Mach number and SA model, Ref. [22] discusses water droplets impact characteristics on NACA0012 type turbine, and the calculation results show the matching degree with the experimental results is good, and the numerical approach is acceptable. With an asymmetric heating surface, Ref. [23] selects the k-epsilon turbulence model and Mach number 0.7 to evaluate the NACA0012 anti-icing performance. The research conclusions indicate that the aerodynamic performance could be promoted through the extended heating surface of the suction surface. The impacts of Mach number and ambient temperature on the icing shape and icing growth rate are investigated by Ref. [24], which adopts the SA turbulence model. Regarding the blunt trailing edge, existing literature primarily focuses on noise generation and validation of associated prediction methods, while limited attention has been given to investigating the aerodynamic prediction correlation [25], [26].
In summary, the existing research primarily centers on examining the impact of geometrical alterations on the properties of the external flow field based on a trailing edge shape [27]. There is limited investigation into how the shape of the NACA0012 trailing edge affects the precision of aerodynamic predictions, with only a few studies addressing this aspect [28], [29]. But these studies are all focus on incompressible conditions. During the typical trajectory of hypersonic vehicle shown in Fig. 1, over 90 % of the flight path occurs under hypersonic conditions with a typical Mach number exceeding 5, which is the predominant aerodynamic environment encountered by scramjet-propelled vehicle. However, the maximum Mach number achieved in the aforementioned simulations is below 3, indicating potential deviations from established research conclusions regarding simulation parameter selection for hypersonic conditions. To date, we have not found reliable literature analyzing the influence of trailing edge shape under hypersonic conditions. Meanwhile, the existing literature lacks comprehensive details on the various methodologies employed to establish the NACA0012 model, and the associated CFD simulations commonly incorporate a fixed far field distance and turbulence model. Furthermore, the literature reviewed thus far has paid little attention to the appropriate values of crucial grid parameters such as cell Reynolds number [30] and aspect ratio [31] near the shock, which are characterized by NACA0012. Reliable references addressing the impact of these parameters on numerical accuracy are currently lacking. In summary, there is an insufficient investigation on the selection criteria for these key parameters under hypersonic conditions.
In this study, we select the NACA0012 airfoil as the characterized object to generate computational external flow fields. Our objective is to perform simulations to investigate the influence of trailing edge shape, modeling method, far field distance, and turbulence model on prediction accuracy under hypersonic conditions. By comparing numerical results with wind tunnel data, we establish a correlation between prediction accuracy and simulation parameters and identify an optimal simulation configuration for future reference. This research contributes to the field of environmental prediction for hypersonic flight vehicles. Furthermore, based on the identified optimal simulation configuration mentioned above, we are able to predict environmental conditions along the trajectory of hypersonic vehicle during flight. These predictions provide valuable information for vehicle optimization and thermal protection design.
2. Simulation fundamentals
To look for a suitable simulation scheme for the aerodynamic environment prediction, the appropriate parameter values of the grid strategy and numerical method should be identified through validation tests. P is the local static pressure, and Pt is the static pressure of the free stream. T is the local static temperature, and Tt is the static temperature of free stream. U is the local velocity, and Ut is the velocity of free stream. We adopt the wind tunnel data of P/Pt, T/Tt and U/Ut from Ref. [13] as the reference data, where the location range of P/Pt and T/Tt is x/L∈ [–0.007 0] and the location range of U/Ut is y/L∈[0.01 0.1] at x/L = 0.95. The initial values are as follows: Reynolds number (Re) is 10e6, the Mach number (Ma) is 10, Pt is 576 Pa, Tt is 81.2 K, and the wall temperature of airfoil (TW) is 311 K. Ansys ICEM CFD and Ansys fluent are chosen as the meshing tool and the CFD simulation tool, respectively. The calculation process is 16-core parallel, and the precision is double.
2.1. Grid strategy
2.1.1. NACA0012 computational domains
Firstly, we need to choose an appropriate modeling method to design NACA0012 model, which is the basis for establishing computational domain. There exists three NACA0012 modeling methods: NACA4 digital generator, Airfoil tools and definition formula. NACA4 digital generator offers 200 modeling data points and provides the function of the close trailing edge. Therefore, it could be used to design the sharp trailing edge NACA0012 model. Airfoil tools offers 132 modeling data points. The established trailing edge based on this method is not closed and requires a manual connection. So, this method could design the blunt trailing edge. Eq. (1) is the Definition formula, in which the value of x represents the point on the X-axis and the value of y corresponds to the point on the Y-axis. The 200 and 132 X-axis data points offered by NACA4 and Airfoil tools could be substituted into the definition formula to calculate the related Y-axis data points to build the NACA0012 model. In summary, the NACA0012 has two trailing edge shapes, three modeling methods, two data point sources, and different numbers of modeling data points. Therefore, we establish six NACA0012 airfoils, shown in Table 1, to investigate the selection principles of NACA0012 modeling method parameters. Fig. 2 demonstrates the related NACA0012 models, and the airfoil characteristic length (L) is 1 m. There exist significant positional differences between different modeling data points:
Table 1The designed six NACA0012s and the corresponding modeling methods
Trailing edge shape | Modeling method | |
One blunt trailing edge | Airfoil tools (132 points) | |
Five sharp trailing edges | Naca4 (200 points) | |
Definition formula | Adopts 132 points from Airfoil tools | |
Definition formula | We double 132 points to 264 points, then substitute them into definition formula | |
Definition formula | Adopts 200 points from NACA4 | |
Definition formula | We double 200 points to 400 points, then substitute them into definition formula |
After establishing the NACA0012 models, we need to select the proper far field distance to establish the computational domain of the NACA0012 airfoil to perform numerical simulation. Ansys suggests the far field distance should be 12-20 times L [32]. However, Ansys only provides a suggested range without providing specific values or analyzing the impact of different far field distances on numerical calculation accuracy. By selecting far field distances of 12L, 16L, and 20L and conducting validation tests using wind tunnel data, Ref. [29] examines the correlation between the accuracy of the far field distance in the incompressible external flow field. The findings suggest a discernible relationship between the far field distance and numerical accuracy in the incompressible external flow field. In this study, based on the same research object NACA0012, we still select 12L/16L/20L far field distances to create computational domains. Our investigation focuses on examining the relationship between these distances and numerical accuracy under hypersonic conditions, while also comparing them to incompressible external flow fields [33]. Fig. 3 demonstrates the established two trailing edge types of computational domains, where the black dot is the coordinate origin. The INLET and OUTLET serve as the input and output boundaries, respectively, employing the Pressure far field condition. The WALL boundary, highlighted in red, is characterized by the no-slip, isothermal wall condition. The airfoil surfaces serve as the primary source of the turbulence and the mean vorticity, and the accuracy of numerical predictions for turbulence in wall-bounded flows is heavily influenced by the near-wall meshing. Therefore, finer meshing areas are created by further dividing blocks in close proximity to the airfoil surface. As illustrated in Fig. 3, the cyan lines indicate the grid division. For the sharp trailing edge, the block at the end is folded, whereas it remains in place for the blunt trailing edge.
Fig. 2Six NACA0012 models
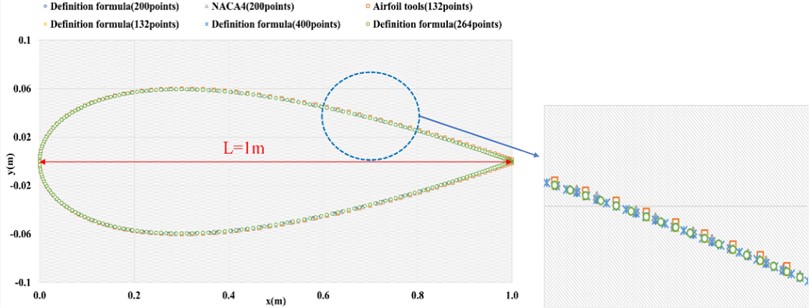
Fig. 3The two types of computational domains
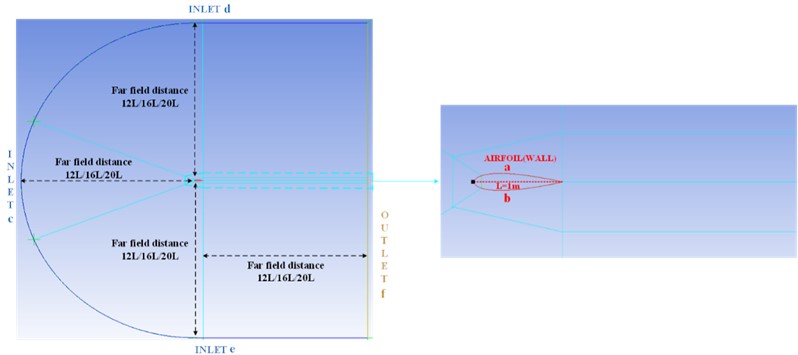
a) Computational domain of the sharp trailing edge and the grid division
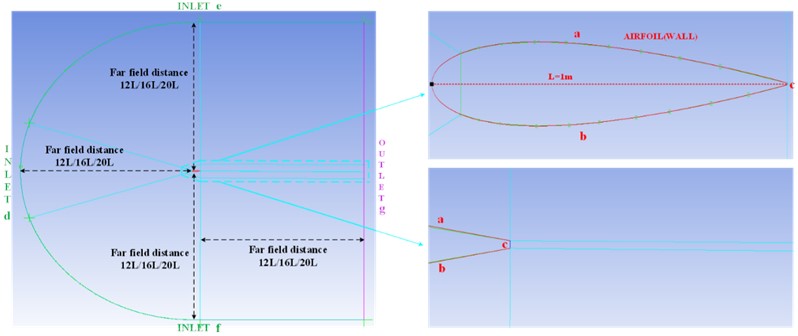
b) Computational domain of the blunt trailing edge and the grid division
2.1.2. Grid parameters
The innermost layer of the near wall is the viscous sublayer, where viscosity has the dominant role in the heat and momentum. The first layer cells of the boundary layer are proposed to exist within the viscous sublayer, with the height value (yH) being directly correlated to y+ and the calculation process is displayed in Fig. 4. The calculations of Re, Ut, air speed (Cair), friction coefficient (Cf), shear stress (τW), friction velocity (μt), and the distance from the wall to the centroid of the wall adjacent cells (yp) are shown from Eqs. (2-8):
where Tt is 81.2 K and Pt is 576 Pa, the flow density (ρ) is about 0.0247 kg/m3. Cair is 180.6 m/s and Ma is 10. Hence, Ut is 1806m/s. Re is 10e06, ρ, L and Ut are substituted into Eq. (2) and flow viscosity (μ) is about 4.46082 Pa·s. Then we could solve yp according to Eqs. (5-8). At last, yH is calculated according to Eq. (9):
Fig. 4The calculation process of yH
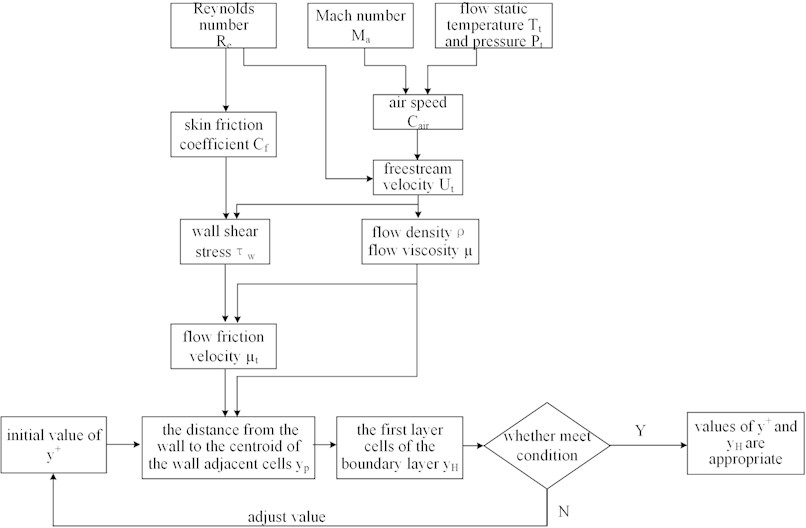
Since the empirical formula (Cf) is used in the above calculation process [34], yH value is an estimate, and it needs to be tested repeatedly through numerical simulation to ensure that the maximum value of y+ at airfoil surface is less than 1 [35]. The initial value of y+ is 1, and yH is 4.6e-5 m could be estimated according to the above Equations. However, tests show that the maximum value of y+ at the airfoil surface exceeds 1 during simulations, which indicates that the initial value of y+ is inappropriate. Through repeated testing, y+ is 0.3 and yH is 1.4e-5 m could meet the condition.
Secondly, it is crucial to evaluate the quality of the mesh utilized in a simulation, encompassing an examination of diverse metrics such as aspect ratio and determinant. In particular, meticulous attention must be paid to the aspect ratio since an excessively small value of yH could potentially lead to a large aspect ratio value. This scenario may result in floating-point overflow or calculation divergence, ultimately leading to simulation failure. In this study, we validate the stability of numerical simulations by conducting CFD tests to determine the appropriate aspect ratio and determinant values. At 12L/16L/20L three far field distances, the maximum aspect ratios and the minimum determinants for the sharp and blunt trailing edge shapes are (2100 0.84), (3310 0.881), (4460 0.873) and (2760 0.894), (3680 0.886), (4770 0.827) respectively. These findings ensure the robustness of our numerical simulations.
Fig. 5Mean error ratios of P/Pt, T/Tt, U/Ut at three far field distances under three grid levels (NACA4)
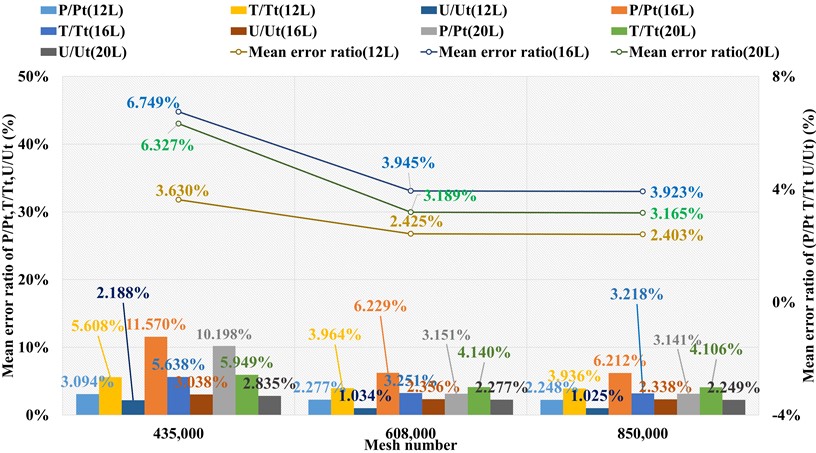
Thirdly, grid independency should be performed to confirm the appropriate total mesh cells number. Three modeling methods are applied to establish three types NACA0012 models: sharp trailing edge (based on NACA4), sharp trailing edge (based on definition formula), and blunt trailing edge (based on Airfoil tools) and we adopt the sharp trailing edge designed by NACA4, combined with the SST k-omega model and ROE flux type as an example. The numerical results of P/Pt, T/Tt, U/Ut at location ranges and related error ratios with wind tunnel data are shown in Fig. 5. At 12L far field distance, the average error ratios of P/Pt, T/Tt, and U/Ut under three grid levels at all calculating locations are (3.094 % 5.608 % 2.188 %), (2.277 % 3.964 % 1.034 %), and (2.248 % 3.936 % 1.025 %). Hence, the total mean error ratios of (P/PtT/TtU/Ut) for the three grid levels are 3.630 %, 2.425 %, and 2.403 %. Similarly, the average error ratios for three grid levels under 16L are (11.570 % 5.638 % 3.038 %), (6.229 % 3.251 % 2.356 %), and (6.212 % 3.218 % 2.338 %) and the corresponding total mean error ratios are 6.749 %, 3.945 %, and 3.923 %. The average error ratios under 20L are (10.198 % 5.949 % 2.835 %), (3.151 % 4.140 % 2.277 %), and (3.141 % 4.106 % 2.249 %) and the corresponding total mean error ratios are 6.327 %, 3.189 %, and 3.165 %. As depicted in Fig. 5, at three far field distance, with the mesh number increases from 608,000 to 850,000, the numerical error ratio hardly changes, and the other two types present similar grid independency performances as shown in Figs. 6 and 7. Therefore, the mesh with 608,000 could meet the requirement of grid independency. The mesh views of the two trailing edge shapes are depicted in Figs. 8-9.
Fig. 6Mean error ratios of P/Pt, T/Tt, U/Ut at three far field distances under three grid levels (Airfoil tools)
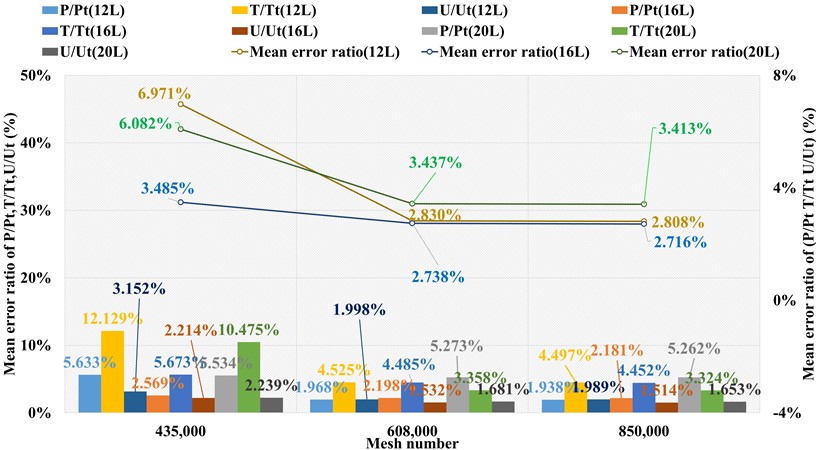
Fig. 7Mean error ratios of P/Pt, T/Tt, U/Ut at three far field distances under three grid levels (Definition formula)
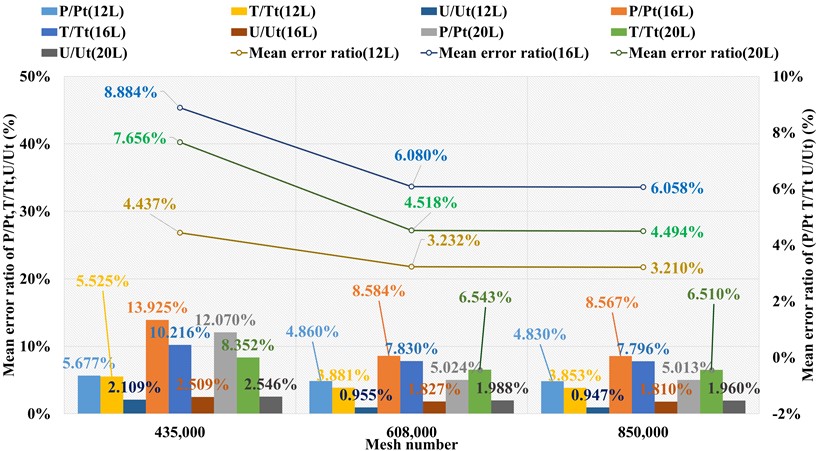
Fig. 8The mesh views of the sharp trailing edge
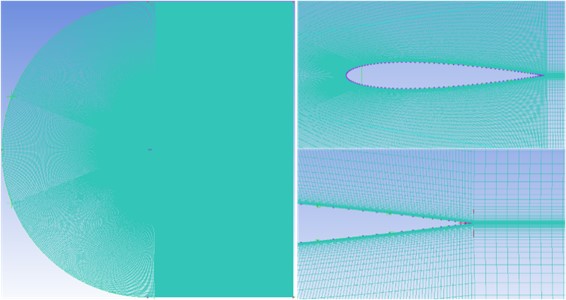
Fig. 9The mesh views of the blunt trailing edge
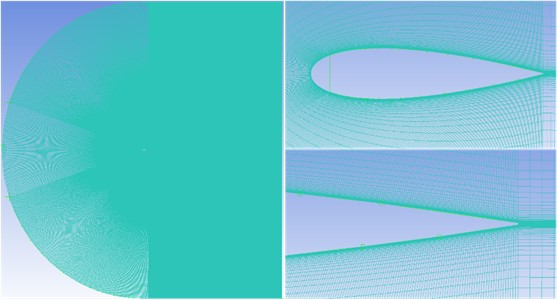
2.2. Numerical method
2.2.1. Turbulence model
When working with transonic fluids, it is necessary to manage compression and heat transfer. This necessitates solving control equations such as mass continuity, momentum (the NS equation), and energy. Since turbulent flow exists, additional transport equations must be solved. Turbulence is defined as unsteady random motion in fluids with medium to high Reynolds numbers, as described by the NS equation. However, direct numerical simulation (DNS) calculations can be time-consuming, necessitating the averaging of the NS equation to reduce turbulence components. The Reynolds Averaged Navier-Stokes (RANS) model is widely used to average the turbulence fluctuation time term. This method employs turbulent viscosity to calculate Reynolds stress and solve the RANS equations. The k-epsilon, SST k-omega, and Spalart-Allmaras (SA) turbulence models are widely accepted and relatively accurate for most numerical simulation applications [36]. However, the k-epsilon model exhibits limited sensitivity to adverse pressure gradients and boundary layer separation, resulting in delayed predictions and separation. Consequently, it is unsuitable for investigating the aerodynamic external flow field in this paper. The k-omega model demonstrates superior performance over the k-epsilon model in predicting adverse pressure gradients and boundary layer flow, showcasing its enhanced capabilities. Furthermore, the SST k-omega model effectively addresses the sensitivity issue of the original k-omega model to freestream conditions, thereby enhancing its applicability [37]. Moreover, the Spalart-Allmaras (SA) turbulence model is specifically tailored for aerospace applications involving wall-bounded flows and exhibits exceptional predictive capabilities for adverse pressure gradient boundary layers [38]. In conclusion, we employ SA and SST k-omega models.
Regarding the INLET boundary, when utilizing the SST k-omega, we employ the intensity and viscosity ratio turbulence method with values of 1 % and 1. In case of adopting the SA model, the turbulent viscosity ratio method is chosen and set its value to 1. The same actions are done for the OUTLET boundary. Furthermore, careful consideration should be given to selecting an appropriate upwind order for modifying turbulent viscosity in relation to the SA model. According to Ref. [30], there exists a situation where the performance of the first order is better than that of the second order. So, we execute a numerical comparison between these two upwind schemes. We still take the sharp trailing edge designed by NACA4, combined with ROE flux type as an example to carry out the analysis. The numerical results of P/Pt, T/Tt, and U/Ut at location ranges and related error ratios with wind tunnel data are shown in Tables 2-4. For the first order, under three far field distances, the mean error ratios of (P/Pt, T/Tt and U/Ut) at all calculating locations are (9.18 % 4.96 % 3.02 %), (9.38 % 7.71 % 2.06 %), and (7.42 % 7.11 % 2.72 %). The corresponding total mean error ratios of (P/PtT/TtU/Ut) are 5.72 %, 6.38 %, and 5.75 %. Similarly, for the second order, the mean error ratios under three far field distances are (4.42 % 2.16 % 1.90 %), (5.33 % 5.24 % 1.61 %), and (4.69 % 4.28 % 1.73 %), and the total mean error ratios are 2.83 %, 4.06 %, and 3.57 %. Hence, the second order upwind is chosen.
Table 2Numerical results comparison of P/Pt between the first-order upwind and second–order upwind of the modified turbulent viscosity
Type of upwind order | x/L locations (m) and P/Pt wind tunnel data (P/Pt numerical results and error ratios) | ||||||||
–0.007 | –0.006 | –0.005 | –0.004 | –0.003 | –0.002 | –0.001 | 0 | ||
103.63 | 114.55 | 117.87 | 119.40 | 121.74 | 122.10 | 123.39 | 123.83 | ||
First-order | 12L | 81.68 | 106.49 | 114.80 | 110.43 | 110.71 | 112.04 | 112.79 | 112.42 |
21.19 % | 7.04 % | 2.60 % | 7.51 % | 9.06 % | 8.24 % | 8.59 % | 9.22 % | ||
16L | 63.99 | 101.22 | 118.76 | 117.55 | 120.54 | 125.16 | 134.83 | 136.33 | |
38.25 % | 11.64 % | 0.76 % | 1.55 % | 0.99 % | 2.51 % | 9.27 % | 10.10 % | ||
20L | 107.09 | 106.96 | 105.16 | 105.66 | 106.74 | 110.22 | 118.13 | 122.88 | |
3.34 % | 6.63 % | 10.79 % | 11.5 % | 12.32 % | 9.73 % | 4.27 % | 0.77 % | ||
Second-order | 12L | 93.15 | 112.16 | 117.71 | 119.13 | 122.20 | 125.88 | 134.72 | 136.43 |
10.11 % | 2.09 % | 0.14 % | 0.23 % | 0.38 % | 3.10 % | 9.18 % | 10.17 % | ||
16L | 106.92 | 112.50 | 115.21 | 115.48 | 115.45 | 115.19 | 113.54 | 107.39 | |
3.18 % | 1.79 % | 2.26 % | 3.28 % | 5.17 % | 5.66 % | 7.99 % | 13.28 % | ||
20L | 102.75 | 106.52 | 113.59 | 117.72 | 122.04 | 126.47 | 136.72 | 136.17 | |
0.85 % | 7.01 % | 3.63 % | 1.41 % | 0.25 % | 3.58 % | 10.8 % | 9.96 % |
Table 3Numerical results comparison of T/Tt between the first-order upwind and second-order upwind of the Modified turbulent viscosity
Type of upwind order | x/L locations (m) and T/Tt wind tunnel data (T/Ttnumerical results and error ratios) | ||||||||
–0.007 | –0.006 | –0.005 | –0.004 | –0.003 | –0.002 | –0.001 | 0 | ||
18.15 | 20.19 | 20.28 | 20.43 | 20.52 | 20.66 | 21.04 | 21.33 | ||
First-order | 12L | 15.34 | 18.71 | 20.36 | 20.78 | 20.86 | 20.79 | 20.39 | 19.33 |
15.50 % | 7.31 % | 0.38 % | 1.70 % | 1.68 % | 0.65 % | 3.11 % | 9.35 % | ||
16L | 12.08 | 17.15 | 20.02 | 20.13 | 20.30 | 20.53 | 20.44 | 20.07 | |
33.47 % | 15.03 % | 1.26 % | 1.49 % | 1.07 % | 0.62 % | 2.83 % | 5.91 % | ||
20L | 19.59 | 19.92 | 19.86 | 19.62 | 19.58 | 20.02 | 20.44 | 14.71 | |
7.96 % | 1.32 % | 2.09 % | 3.96 % | 4.57 % | 3.09 % | 2.87 % | 31.04 % | ||
Second-order | 12L | 17.55 | 19.13 | 20.06 | 20.17 | 20.30 | 20.55 | 20.64 | 20.71 |
3.28 % | 5.24 % | 1.08 % | 1.25 % | 1.08 % | 0.52 % | 1.89 % | 2.92 % | ||
16L | 19.57 | 20.03 | 20.26 | 20.33 | 20.31 | 20.47 | 20.10 | 15.72 | |
7.82 % | 0.79 % | 0.07 % | 0.51 % | 1.03 % | 0.92 % | 4.46 % | 26.3 % | ||
20L | 20.47 | 20.33 | 20.20 | 20.06 | 20.08 | 20.11 | 20.29 | 19.16 | |
12.79 % | 0.68 % | 0.39 % | 1.79 % | 2.17 % | 2.68 % | 3.58 % | 10.18 % |
Table 4Numerical results comparison of U/Ut between the first-order upwind and second-order upwind of the modified turbulent viscosity
Type of upwind order | y/L locations (m) at x/L=0.95 m (U/Utnumerical results and error ratios) | ||||||||||
0.01 | 0.02 | 0.03 | 0.04 | 0.05 | 0.06 | 0.07 | 0.08 | 0.09 | 0.1 | ||
0.6907 | 0.8199 | 0.8556 | 0.8667 | 0.8705 | 0.8711 | 0.8740 | 0.8746 | 0.8771 | 0.8801 | ||
First- order | 12L | 0.6315 | 0.8444 | 0.8823 | 0.8837 | 0.8858 | 0.8899 | 0.8920 | 0.8963 | 0.9020 | 0.8997 |
8.56 % | 2.98 % | 3.13 % | 1.97 % | 1.75 % | 2.16 % | 2.06 % | 2.49 % | 2.84 % | 2.22 % | ||
16L | 0.6392 | 0.8383 | 0.8668 | 0.8606 | 0.8737 | 0.8797 | 0.8883 | 0.8892 | 0.8943 | 0.8998 | |
7.46 % | 2.24 % | 1.31 % | 0.71 % | 0.36 % | 0.98 % | 1.63 % | 1.67 % | 1.96 % | 2.23 % | ||
20L | 0.6487 | 0.8456 | 0.8862 | 0.8895 | 0.8865 | 0.8852 | 0.8904 | 0.8933 | 0.8971 | 0.8983 | |
6.08 % | 3.13 % | 3.57 % | 2.64 % | 1.84 % | 1.61 % | 1.88 % | 2.15 % | 2.29 % | 2.06 % | ||
Second- order | 12L | 0.6405 | 0.8374 | 0.8733 | 0.8777 | 0.8795 | 0.8807 | 0.8820 | 0.8843 | 0.8870 | 0.8887 |
7.26 % | 2.13 % | 2.07 % | 1.27 % | 1.02 % | 1.10 % | 0.92 % | 1.12 % | 1.13 % | 0.97 % | ||
16L | 0.6339 | 0.8357 | 0.8624 | 0.8666 | 0.8707 | 0.8756 | 0.8801 | 0.8851 | 0.8893 | 0.8918 | |
8.23 % | 1.93 % | 0.79 % | 0.01 % | 0.01 % | 0.51 % | 0.69 % | 1.20 % | 1.39 % | 1.32 % | ||
20L | 0.6547 | 0.8416 | 0.8802 | 0.8815 | 0.8795 | 0.8788 | 0.8794 | 0.8816 | 0.8841 | 0.8863 | |
5.21 % | 2.64 % | 2.87 % | 1.71 % | 1.03 % | 0.88 % | 0.62 % | 0.81 % | 0.81 % | 0.70 % |
2.2.2. Flux type and spatial discretization
An appropriate scheme is needed to evaluate the flux component. According to Ref. [39], the cylinder is taken as a research object to investigate the simulation performance of different flux types, and the conclusions demonstrate that the results of the ROE and AUSM are closer to the reference data. Therefore, we adopt these two types to study the capability of the simulation accuracy of aerodynamic prediction characterized by NACA0012 under hypersonic conditions. For the flow discretization, the second order upwind is selected. A suitable gradient calculation scheme is also needed, based on which the cell face scalar values could be constructed. The calculation of related diffusion terms and velocity derivatives can be done. There are three types of schemes (node-based/cell-based/least cell-based). Out of these three, the least cell-based scheme is advantageous because it provides comparable accuracy to the node-based scheme, has fewer computing resources, and avoids spurious oscillations. Hence, the least cell-based with the standard gradient limiter is applied. In addition, when the Mach number is bigger than 5, the density-based solver is employed. Since the Mach number of validation tests is 10, it should be considered whether there exists real gas effect. The air critical pressure (Pc) is 3.77 MPa, and if the ratio of P and Pc is much less than 1, then we could select the ideal gas. During the numerical simulation process, the value of P increases from the initial 576 Pa to the maximum 73728 Pa. The maximum ratio of P and Pc is about 0.019, which satisfies the condition mentioned above. Hence, flow density (ρ) selects the ideal gas.
3. Numerical results and discussion
3.1. Numerical results
Based on the description in Grid strategy and Numerical Method, we apply six NACA0012 models, three far field distances, two turbulence models and two flux types to construct the simulation configurations and a total of seventy-two sets of numerical calculations are carried out. Tables 5-22 demonstrate the numerical results of P/Pt, T/Tt, U/Ut at sampling locations and Figs. 10-15 demonstrate the corresponding numerical error ratio distributions of P/Pt, T/Tt, U/Ut compared with the wind tunnel data. The bold black values in the tables below x/L sampling positions represent the corresponding wind tunnel test data, and the red dashed diamond shapes in the figures indicate wind tunnel data at those positions. Table 23 demonstrates the mean error ratios of P/Pt, T/Tt, U/Ut for six NACA0012 models under different simulation configurations. Through the error ratio comparison, the optimal mean error ratio of (P/PtT/TtU/Ut) of 2.05 % could be achieved based on the configuration of sharp trailing edge (definition formula) + 16L far field distance + SA turbulence model + ROE flux type.
Table 5Numerical results of P/Pt of blunt trailing edge adopting two turbulence models, three far field distances and two flux types
NACA0012 models | x/L (m) and P/Pt wind tunnel data (P/Pt numerical data) | ||||||||
–0.007 | –0.006 | –0.005 | –0.004 | –0.003 | –0.002 | –0.001 | 0 | ||
103.6285 | 114.5508 | 117.8656 | 119.3983 | 121.7447 | 122.1036 | 123.3942 | 123.8330 | ||
SST+12L | ROE | 103.1758 | 114.2245 | 119.3853 | 122.5377 | 123.7579 | 124.7891 | 127.2207 | 128.9688 |
AUSM | 115.2700 | 114.4670 | 115.0006 | 114.7569 | 113.8172 | 111.8231 | 102.0335 | 97.0301 | |
SA+12L | ROE | 6.0925 | 35.2682 | 107.9680 | 118.2251 | 118.7486 | 121.5961 | 129.3444 | 138.5572 |
AUSM | 113.6156 | 114.3060 | 113.3640 | 113.4916 | 114.8708 | 118.5805 | 122.1218 | 124.7841 | |
SST+16L | ROE | 101.3087 | 113.2991 | 119.0844 | 122.3194 | 123.5652 | 124.6285 | 127.1412 | 128.9871 |
AUSM | 126.2989 | 125.2276 | 124.6631 | 126.3320 | 127.7393 | 129.1166 | 123.0015 | 109.6105 | |
SA+16L | ROE | 112.1930 | 112.6418 | 113.9845 | 114.8477 | 115.8725 | 120.3363 | 125.7635 | 127.4569 |
AUSM | 115.5999 | 116.4176 | 117.9377 | 122.8046 | 126.0157 | 129.6497 | 135.4119 | 138.2230 | |
SST+20L | ROE | 108.5238 | 119.5044 | 122.4766 | 125.7646 | 127.0867 | 128.2546 | 131.3245 | 133.7835 |
AUSM | 85.9375 | 103.1402 | 111.8435 | 111.6520 | 111.9114 | 112.0232 | 111.3510 | 110.1554 | |
SA+20L | ROE | 88.2687 | 113.0102 | 122.2196 | 123.6521 | 126.3275 | 128.9982 | 134.4160 | 135.5053 |
AUSM | 108.2922 | 118.8877 | 118.7423 | 122.1060 | 125.9117 | 129.5463 | 140.4347 | 152.9953 |
Table 6Numerical results of T/Tt of blunt trailing edge shape adopting two turbulence models, three far field distances and two flux types
NACA0012 models | x/L (m) and T/Tt wind tunnel data (T/Tt numerical data) | ||||||||
–0.007 | –0.006 | –0.005 | –0.004 | –0.003 | –0.002 | –0.001 | 0 | ||
18.152 | 20.190 | 20.284 | 20.427 | 20.521 | 20.664 | 21.043 | 21.327 | ||
SST+12L | ROE | 19.918 | 20.418 | 20.675 | 20.792 | 20.765 | 20.680 | 20.151 | 17.892 |
AUSM | 20.097 | 20.334 | 20.580 | 20.726 | 20.290 | 19.677 | 18.936 | 17.701 | |
SA+12L | ROE | 1.996 | 7.765 | 18.344 | 18.839 | 18.886 | 18.838 | 18.760 | 21.042 |
AUSM | 18.489 | 18.647 | 18.768 | 18.914 | 19.123 | 19.572 | 19.940 | 20.739 | |
SST+16L | ROE | 19.825 | 20.387 | 20.678 | 20.805 | 20.785 | 20.708 | 20.191 | 17.840 |
AUSM | 20.592 | 20.758 | 20.866 | 20.959 | 20.879 | 20.700 | 18.750 | 5.439 | |
SA+16L | ROE | 20.483 | 20.664 | 20.806 | 20.898 | 20.907 | 20.604 | 19.867 | 19.799 |
AUSM | 21.109 | 21.046 | 20.979 | 21.037 | 21.168 | 21.327 | 21.626 | 18.600 | |
SST+20L | ROE | 18.909 | 20.412 | 20.572 | 20.688 | 20.685 | 20.628 | 20.178 | 18.384 |
AUSM | 17.365 | 19.335 | 19.951 | 20.074 | 20.074 | 20.443 | 20.074 | 15.271 | |
SA+20L | ROE | 16.506 | 19.037 | 20.212 | 20.459 | 20.567 | 20.657 | 20.795 | 20.293 |
AUSM | 18.681 | 19.851 | 20.331 | 20.683 | 20.925 | 21.156 | 20.720 | 21.642 |
Table 7Numerical results of U/Ut of blunt trailing edge adopting two turbulence models, three far field distances and two flux types
NACA0012 models | y/L (m) at x/L= 0.95 m and U/Ut wind tunnel data (U/Ut numerical data) | ||||||||||
0.01 | 0.02 | 0.03 | 0.04 | 0.05 | 0.06 | 0.07 | 0.08 | 0.09 | 0.1 | ||
0.6907 | 0.8199 | 0.8556 | 0.8667 | 0.8705 | 0.8711 | 0.8740 | 0.8746 | 0.8771 | 0.8801 | ||
SST+12L | ROE | 0.5939 | 0.8335 | 0.8538 | 0.8620 | 0.8680 | 0.8733 | 0.8774 | 0.8818 | 0.8855 | 0.8876 |
AUSM | 0.5529 | 0.7516 | 0.7981 | 0.8395 | 0.8628 | 0.8714 | 0.8720 | 0.8719 | 0.8731 | 0.8742 | |
SA+12L | ROE | 0.6364 | 0.8604 | 0.8746 | 0.8881 | 0.8845 | 0.8826 | 0.8825 | 0.8832 | 0.8849 | 0.8861 |
AUSM | 0.6481 | 0.8350 | 0.8642 | 0.8657 | 0.8711 | 0.8769 | 0.8792 | 0.8796 | 0.8802 | 0.8807 | |
SST+16L | ROE | 0.6323 | 0.8421 | 0.8568 | 0.8639 | 0.8695 | 0.8744 | 0.8782 | 0.8823 | 0.8858 | 0.8878 |
AUSM | 0.5359 | 0.7300 | 0.7756 | 0.8271 | 0.8548 | 0.8636 | 0.8694 | 0.8773 | 0.8841 | 0.8879 | |
SA+16L | ROE | 0.6659 | 0.8723 | 0.8732 | 0.8730 | 0.8763 | 0.8807 | 0.8840 | 0.8871 | 0.8897 | 0.8912 |
AUSM | 0.6365 | 0.7868 | 0.7936 | 0.8110 | 0.8432 | 0.8701 | 0.8841 | 0.8928 | 0.8965 | 0.8976 | |
SST+20L | ROE | 0.6212 | 0.8438 | 0.8581 | 0.8646 | 0.8696 | 0.8740 | 0.8775 | 0.8814 | 0.8849 | 0.8871 |
AUSM | 0.5947 | 0.8718 | 0.8651 | 0.8665 | 0.8782 | 0.8853 | 0.8871 | 0.8863 | 0.8866 | 0.8873 | |
SA+20L | ROE | 0.6567 | 0.8747 | 0.8762 | 0.8766 | 0.8772 | 0.8783 | 0.8798 | 0.8823 | 0.8853 | 0.8873 |
AUSM | 0.6249 | 0.8669 | 0.8788 | 0.8777 | 0.8738 | 0.8741 | 0.8762 | 0.8800 | 0.8836 | 0.8858 |
3.1.1. Airfoil tools
The corresponding numerical results and distributions of P/Pt, T/Tt and U/Ut are shown in Tables 5-7 and Fig. 10. Table 23 provides the mean error ratios under different simulation configurations. From the aspect of far field distance, the minimum values are 2.696 %, 2.738 %, and 3.436 % in order. From the aspect of turbulence model, the most favorable outcome for SST is 2.696 %, while that of SA is 3.292 %. For the two flux types, the finest value of ROE is 2.696 % and that of AUSM is 3.441 %. In conclusion, the SST+12L configuration, combined with ROE has achieved the minimum total mean error ratio. Compared with other simulation configurations, the corresponding accuracy improvements are 57.249 %, 84.153 %, 21.642 %, 1.532 %, 70.082 %, 18.089 %, 48.237 %, 21.543 %, 55.935 %, 27.146 %, and 27.602 %, respectively.
Fig. 10The numerical result and error ratio distributions of P/Pt, T/Tt and U/Ut of blunt trailing edge
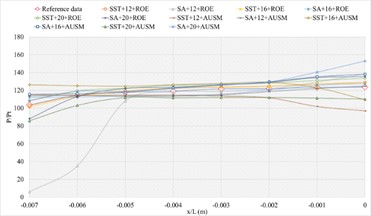
a) Numerical result distribution of P/Pt of the blunt trailing edge
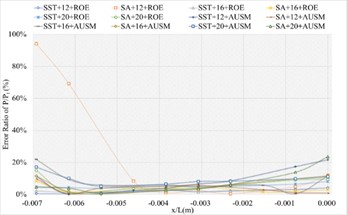
b) Error ratio distribution of P/Pt of the blunt trailing edge
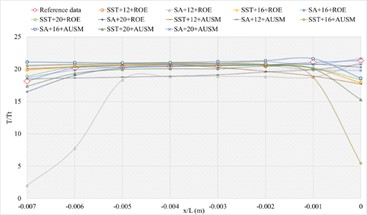
c) Numerical result distribution of T/Tt of the blunt trailing edge
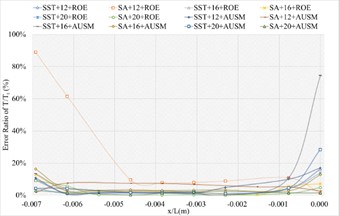
d) Error ratio distribution of T/Tt of the blunt trailing edge
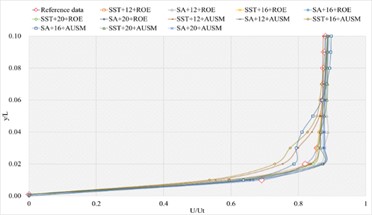
e) Numerical result distribution of U/Ut of the blunt trailing edge
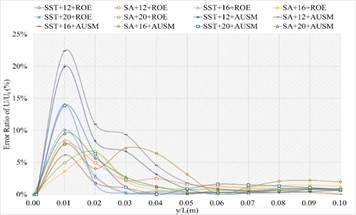
f) Error ratio distribution of U/Ut of the blunt trailing edge
3.1.2. NACA4
The corresponding numerical results and distributions of P/Pt, T/Tt and U/Ut are shown in Tables 8-10 and Fig. 11. Table 23 provides the mean error ratios under different simulation configurations. From the aspect of far field distance, the minimum values are 2.420 %, 3.797 %, and 3.189 % in order. From the aspect of turbulence model, the most favorable outcome for SST is 2.420 %, while that of SA is 2.829 %. For the two flux types, the finest value of ROE is 2.420 % and that of AUSM is 3.797 %.
Table 8Numerical results of P/Pt of sharp trailing edge based on NACA4 adopting two turbulence models, three far field distances and two flux types
NACA0012 models | x/L (m) and P/Pt wind tunnel data (P/Pt numerical data) | ||||||||
–0.007 | –0.006 | –0.005 | –0.004 | –0.003 | –0.002 | –0.001 | 0 | ||
103.6285 | 114.5508 | 117.8656 | 119.3983 | 121.7447 | 122.1036 | 123.3942 | 123.8330 | ||
SST+12L | ROE | 102.6623 | 113.7859 | 119.5540 | 122.7781 | 124.0214 | 125.0571 | 127.6113 | 129.5765 |
AUSM | 125.0601 | 122.6266 | 123.4892 | 130.3869 | 133.7133 | 136.7315 | 138.2960 | 133.3994 | |
SA+12L | ROE | 93.1517 | 112.1581 | 117.7100 | 119.1311 | 122.1984 | 125.8803 | 134.7221 | 136.4293 |
AUSM | 120.0194 | 113.7502 | 110.6636 | 109.2987 | 109.0662 | 109.8360 | 111.0621 | 115.5866 | |
SST+16L | ROE | 90.3901 | 114.5756 | 124.3798 | 125.3509 | 127.3098 | 128.9722 | 132.1900 | 135.2095 |
AUSM | 123.6560 | 124.3612 | 124.9024 | 124.9201 | 124.3482 | 122.1458 | 119.9241 | 118.5962 | |
SA+16L | ROE | 106.9225 | 112.5033 | 115.2072 | 115.4793 | 115.4510 | 115.1886 | 113.5401 | 107.3866 |
AUSM | 118.0516 | 119.6460 | 120.2279 | 120.3639 | 120.1021 | 119.3604 | 115.6705 | 111.5844 | |
SST+20L | ROE | 91.5191 | 108.2494 | 117.8706 | 120.9798 | 122.3012 | 123.3865 | 125.8781 | 127.7352 |
AUSM | 98.4080 | 99.3577 | 98.2276 | 97.5850 | 97.8597 | 100.8383 | 103.7027 | 103.7561 | |
SA+20L | ROE | 102.7478 | 106.5220 | 113.5859 | 117.7168 | 122.0448 | 126.4707 | 136.7221 | 136.1655 |
AUSM | 37.4234 | 73.3389 | 117.5831 | 118.8793 | 121.1027 | 123.9634 | 132.9692 | 140.2752 |
Table 9Numerical results of T/Tt of sharp trailing edge based on NACA4 adopting two turbulence models, three far field distances and two flux types
NACA0012 models | x/L (m) and T/Tt wind tunnel data (T/Tt numerical data) | ||||||||
–0.007 | –0.006 | –0.005 | –0.004 | –0.003 | –0.002 | –0.001 | 0 | ||
18.152 | 20.190 | 20.284 | 20.427 | 20.521 | 20.664 | 21.043 | 21.327 | ||
SST+12L | ROE | 19.860 | 20.361 | 20.640 | 20.773 | 20.761 | 20.693 | 20.219 | 18.605 |
AUSM | 21.769 | 21.834 | 21.924 | 21.832 | 21.495 | 20.869 | 20.137 | 18.077 | |
SA+12L | ROE | 17.555 | 19.131 | 20.061 | 20.174 | 20.297 | 20.552 | 20.642 | 20.707 |
AUSM | 20.927 | 20.926 | 20.499 | 20.374 | 20.430 | 20.417 | 20.365 | 20.634 | |
SST+16L | ROE | 17.182 | 19.264 | 20.271 | 20.609 | 20.704 | 20.696 | 20.454 | 18.930 |
AUSM | 20.769 | 21.064 | 21.367 | 21.568 | 21.237 | 20.772 | 20.092 | 15.503 | |
SA+16L | ROE | 19.570 | 20.031 | 20.265 | 20.325 | 20.309 | 20.469 | 20.101 | 15.717 |
AUSM | 20.084 | 20.383 | 20.633 | 20.941 | 20.775 | 20.338 | 20.090 | 18.476 | |
SST+20L | ROE | 18.834 | 20.122 | 20.647 | 20.833 | 20.832 | 20.610 | 20.262 | 17.108 |
AUSM | 17.580 | 17.965 | 18.472 | 18.797 | 19.136 | 19.811 | 19.313 | 18.382 | |
SA+20L | ROE | 20.471 | 20.327 | 20.202 | 20.064 | 20.075 | 20.107 | 20.287 | 19.159 |
AUSM | 9.741 | 15.713 | 20.595 | 20.631 | 20.719 | 20.863 | 20.630 | 20.683 |
Table 10Numerical results of U/Ut of sharp trailing edge based on NACA4 adopting two turbulence models, three far field distances and two flux types
NACA0012 models | y/L (m) at x/L= 0.95 m and U/Ut wind tunnel data (U/Ut numerical data) | ||||||||||
0.01 | 0.02 | 0.03 | 0.04 | 0.05 | 0.06 | 0.07 | 0.08 | 0.09 | 0.1 | ||
0.6907 | 0.8199 | 0.8556 | 0.8667 | 0.8705 | 0.8711 | 0.8740 | 0.8746 | 0.8771 | 0.8801 | ||
SST+12L | ROE | 0.6547 | 0.8416 | 0.8802 | 0.8815 | 0.8795 | 0.8788 | 0.8794 | 0.8816 | 0.8841 | 0.8863 |
AUSM | 0.6162 | 0.7966 | 0.8333 | 0.8513 | 0.8659 | 0.8776 | 0.8842 | 0.8870 | 0.8870 | 0.8873 | |
SA+12L | ROE | 0.5989 | 0.7913 | 0.8351 | 0.8549 | 0.8648 | 0.8712 | 0.8757 | 0.8803 | 0.8841 | 0.8864 |
AUSM | 0.6515 | 0.8304 | 0.8604 | 0.8849 | 0.8960 | 0.8948 | 0.8907 | 0.8876 | 0.8871 | 0.8872 | |
SST+16L | ROE | 0.6405 | 0.8374 | 0.8733 | 0.8777 | 0.8795 | 0.8807 | 0.8820 | 0.8843 | 0.8870 | 0.8887 |
AUSM | 0.5989 | 0.8126 | 0.8572 | 0.8667 | 0.8717 | 0.8770 | 0.8818 | 0.8871 | 0.8913 | 0.8938 | |
SA+16L | ROE | 0.5957 | 0.7883 | 0.8337 | 0.8542 | 0.8642 | 0.8708 | 0.8755 | 0.8805 | 0.8845 | 0.8869 |
AUSM | 0.6457 | 0.8428 | 0.8642 | 0.8679 | 0.8722 | 0.8775 | 0.8822 | 0.8875 | 0.8917 | 0.8942 | |
SST+20L | ROE | 0.6070 | 0.7921 | 0.8348 | 0.8547 | 0.8650 | 0.8718 | 0.8766 | 0.8813 | 0.8848 | 0.8868 |
AUSM | 0.5712 | 0.7690 | 0.7879 | 0.8170 | 0.8482 | 0.8685 | 0.8784 | 0.8839 | 0.8872 | 0.8889 | |
SA+20L | ROE | 0.6339 | 0.8357 | 0.8624 | 0.8666 | 0.8707 | 0.8756 | 0.8801 | 0.8851 | 0.8893 | 0.8918 |
AUSM | 0.6564 | 0.8019 | 0.8492 | 0.8747 | 0.8793 | 0.8809 | 0.8820 | 0.8828 | 0.8836 | 0.8842 |
In conclusion, the SST+12L configuration, combined with ROE has achieved the minimum mean error ratio. Compared with other simulation configurations, the corresponding accuracy improvements are 68.882 %, 14.453 %, 46.120 %, 38.628 %, 55.249 %, 40.390 %, 36.269 %, 24.114 %, 71.737 %, 32.125 %, and 73.366 %, respectively.
Fig. 11The numerical result and error ratio distributions of P/Pt, T/Tt and U/Ut of sharp trailing edge based on NACA4
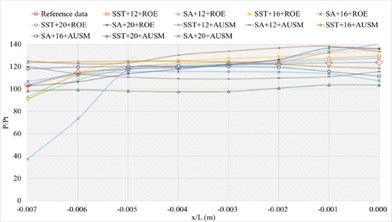
a) Numerical result distribution of P/Pt of the sharp trailing edge based on NACA4
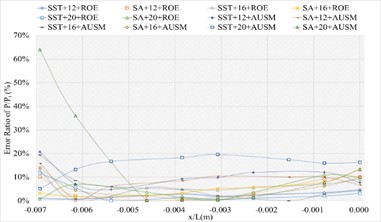
b) Error ratio distribution of P/Pt of the sharp trailing edge based on NACA4
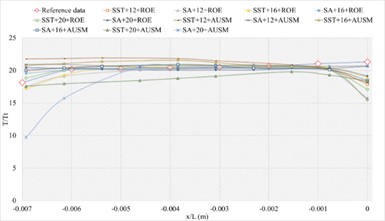
c) Numerical result distribution of T/Tt of the sharp trailing edge based on NACA4
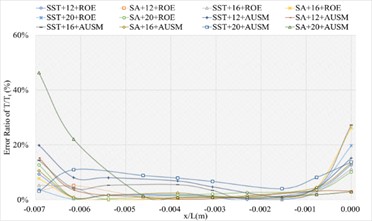
d) Error ratio distribution of T/Tt of the sharp trailing edge based on NACA4
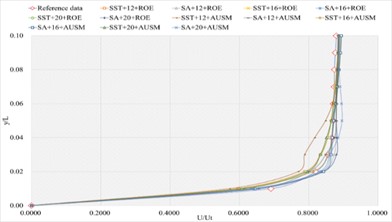
e) Numerical result distribution of U/Ut of the sharp trailing edge based on NACA4
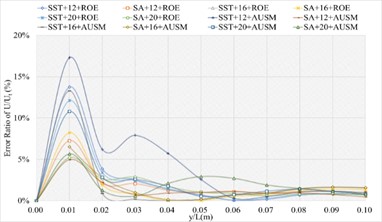
f) Error ratio distribution of U/Utof the sharp trailing edge based on NACA4
3.1.3. Definition formula adopting 132 points
The corresponding numerical results and distributions of P/Pt, T/Tt and U/Ut are shown in Tables 11-13 and Fig.12. Table 23 provides the mean error ratios under different simulation configurations. From the aspect of far field distance, the minimum values are 3.136 %, 2.640 %, and 2.975 % in order. From the aspect of turbulence model, the most favorable outcome for SST is 2.640 %, while that of SA is 2.975 %. For the two flux types, the finest value of ROE is 2.640 % and that of AUSM is 3.136 %. In conclusion, the SST+16L configuration, combined with ROE has achieved the minimum mean error ratio. Compared with other simulation configurations, the corresponding accuracy improvements are72.073 %, 15.821 %, 61.731 %, 58.624 %, 25.987 %, 63.533 %, 45.070 %, 53.338 %, 31.817 %, 11.290 %, and 66.411 %, respectively.
Table 11Numerical results of P/Pt of sharp trailing edge based on definition formula (132 points) adopting two turbulence models, three far field distances and two flux types
NACA0012 models | x/L (m) and P/Pt wind tunnel data (P/Pt numerical data) | ||||||||
–0.007 | –0.006 | –0.005 | –0.004 | –0.003 | –0.002 | –0.001 | 0 | ||
103.6285 | 114.5508 | 117.8656 | 119.3983 | 121.7447 | 122.1036 | 123.3942 | 123.8330 | ||
SST+12L | ROE | 77.9720 | 97.5837 | 103.9596 | 104.2068 | 105.7531 | 108.2004 | 103.7853 | 93.4325 |
AUSM | 122.9772 | 118.5715 | 117.4966 | 120.4748 | 122.3124 | 123.9481 | 126.0108 | 132.5421 | |
SA+12L | ROE | 126.8481 | 127.4266 | 128.1201 | 128.9839 | 128.9838 | 128.9172 | 128.3850 | 127.1818 |
AUSM | 94.8265 | 106.4431 | 109.2256 | 108.2244 | 108.7156 | 108.9666 | 109.8323 | 131.9843 | |
SST+16L | ROE | 102.8513 | 114.0935 | 119.5973 | 122.7339 | 123.9256 | 124.9008 | 127.3781 | 129.2507 |
AUSM | 116.3570 | 118.2875 | 117.5792 | 117.6293 | 119.3575 | 122.0409 | 122.4785 | 121.0955 | |
SA+16L | ROE | 110.5474 | 142.6264 | 133.8498 | 134.7360 | 135.7823 | 135.8110 | 131.7480 | 133.3460 |
AUSM | 126.5742 | 124.6259 | 124.2431 | 126.2068 | 126.8411 | 127.7232 | 129.5196 | 127.2727 | |
SST+20L | ROE | 89.3645 | 103.6540 | 106.7764 | 103.4379 | 104.8373 | 109.8622 | 112.3890 | 112.5213 |
AUSM | 121.9440 | 118.9971 | 117.6949 | 118.6125 | 119.4331 | 121.7822 | 125.1382 | 132.7346 | |
SA+20L | ROE | 103.6814 | 114.6149 | 117.3158 | 116.9946 | 118.5445 | 122.0046 | 125.1439 | 132.9575 |
AUSM | 67.4088 | 111.4342 | 132.0675 | 125.9648 | 128.9382 | 132.7707 | 143.0583 | 153.6435 |
Table 12Numerical results of T/Tt of sharp trailing edge based on definition formula (132 points) adopting two turbulence models, three far field distances and two flux types
NACA0012 models | x/L (m) and T/Tt wind tunnel data (T/Tt numerical data) | ||||||||
–0.007 | –0.006 | –0.005 | –0.004 | –0.003 | –0.002 | –0.001 | 0 | ||
18.152 | 20.190 | 20.284 | 20.427 | 20.521 | 20.664 | 21.043 | 21.327 | ||
SST+12L | ROE | 15.133 | 16.859 | 18.502 | 19.281 | 20.050 | 20.660 | 19.174 | 18.030 |
AUSM | 19.944 | 20.075 | 20.212 | 20.495 | 20.646 | 20.841 | 21.900 | 19.971 | |
SA+12L | ROE | 20.816 | 20.841 | 20.869 | 20.909 | 20.932 | 20.995 | 20.806 | 10.720 |
AUSM | 17.497 | 18.624 | 19.302 | 19.408 | 19.484 | 19.593 | 19.665 | 18.654 | |
SST+16L | ROE | 19.873 | 20.384 | 20.656 | 20.781 | 20.761 | 20.683 | 20.173 | 18.277 |
AUSM | 18.708 | 19.203 | 19.657 | 19.851 | 20.044 | 20.498 | 20.571 | 14.955 | |
SA+16L | ROE | 16.122 | 20.522 | 22.666 | 23.641 | 23.466 | 20.439 | 19.578 | 20.710 |
AUSM | 21.319 | 21.230 | 21.041 | 20.932 | 20.794 | 20.698 | 21.178 | 17.974 | |
SST+20L | ROE | 17.271 | 19.272 | 20.199 | 20.584 | 20.672 | 20.630 | 19.591 | 17.817 |
AUSM | 21.116 | 21.097 | 21.012 | 20.946 | 20.862 | 20.764 | 20.399 | 20.262 | |
SA+20L | ROE | 18.608 | 19.239 | 19.496 | 19.593 | 19.692 | 19.713 | 19.929 | 18.019 |
AUSM | 11.876 | 16.908 | 20.229 | 20.249 | 20.367 | 20.476 | 19.791 | 21.239 |
Table 13Numerical results of U/Ut of sharp trailing edge based on definition formula (132 points) adopting two turbulence models, three far field distances and two flux types
NACA0012 models | y/L (m) at x/L= 0.95 m and U/Ut wind tunnel data (U/Ut numerical data) | ||||||||||
0.01 | 0.02 | 0.03 | 0.04 | 0.05 | 0.06 | 0.07 | 0.08 | 0.09 | 0.1 | ||
0.6907 | 0.8199 | 0.8556 | 0.8667 | 0.8705 | 0.8711 | 0.8740 | 0.8746 | 0.8771 | 0.8801 | ||
SST+12L | ROE | 0.6204 | 0.7708 | 0.8074 | 0.8356 | 0.8626 | 0.8760 | 0.8801 | 0.8817 | 0.8831 | 0.8839 |
AUSM | 0.6358 | 0.7639 | 0.8122 | 0.8516 | 0.8696 | 0.8724 | 0.8729 | 0.8748 | 0.8781 | 0.8802 | |
SA+12L | ROE | 0.6643 | 0.7866 | 0.8004 | 0.8270 | 0.8482 | 0.8617 | 0.8699 | 0.8765 | 0.8810 | 0.8835 |
AUSM | 0.6456 | 0.7430 | 0.7751 | 0.8323 | 0.8619 | 0.8598 | 0.8560 | 0.8571 | 0.8617 | 0.8649 | |
SST+16L | ROE | 0.6048 | 0.7918 | 0.8350 | 0.8536 | 0.8629 | 0.8690 | 0.8737 | 0.8786 | 0.8829 | 0.8855 |
AUSM | 0.6191 | 0.8289 | 0.8634 | 0.8757 | 0.8805 | 0.8792 | 0.8783 | 0.8799 | 0.8829 | 0.8849 | |
SA+16L | ROE | 0.6488 | 0.8317 | 0.8708 | 0.8756 | 0.8751 | 0.8780 | 0.8819 | 0.8864 | 0.8898 | 0.8918 |
AUSM | 0.7046 | 0.8616 | 0.8639 | 0.8585 | 0.8626 | 0.8700 | 0.8758 | 0.8810 | 0.8846 | 0.8867 | |
SST+20L | ROE | 0.6297 | 0.8086 | 0.8422 | 0.8566 | 0.8647 | 0.8707 | 0.8753 | 0.8803 | 0.8846 | 0.8871 |
AUSM | 0.6331 | 0.7768 | 0.7970 | 0.8270 | 0.8533 | 0.8659 | 0.8709 | 0.8750 | 0.8787 | 0.8810 | |
SA+20L | ROE | 0.6689 | 0.8531 | 0.8696 | 0.8693 | 0.8744 | 0.8798 | 0.8833 | 0.8867 | 0.8900 | 0.8920 |
AUSM | 0.6855 | 0.7899 | 0.7990 | 0.8160 | 0.8456 | 0.8684 | 0.8788 | 0.8834 | 0.8859 | 0.8872 |
Fig. 12The numerical result and error ratio distributions of P/Pt, T/Tt and U/Ut of sharp trailing edge based on definition formula (132 points)
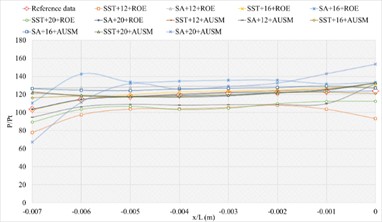
a) Numerical result distribution of P/Pt of the sharp trailing edge based on definition formula (132 points)
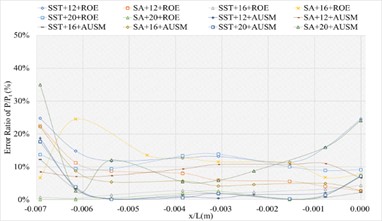
b) Error ratio distribution of P/Pt of the sharp trailing edge based on definition formula (132 points)
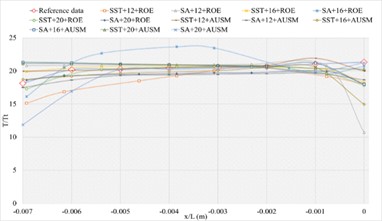
c) Numerical result distribution of T/Tt of the sharp trailing edge based on definition formula (132 points)
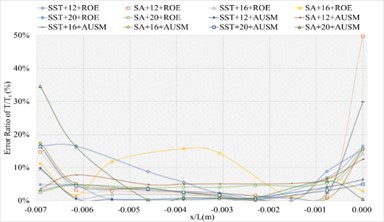
d) Error ratio distribution of T/Tt of the sharp trailing edge based on definition formula (132 points)
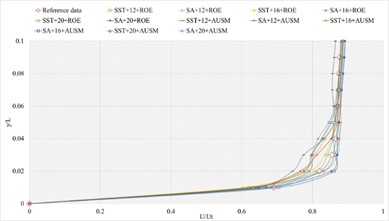
e) Numerical result distribution of U/Ut of the sharp trailing edge based on definition formula (132 points)
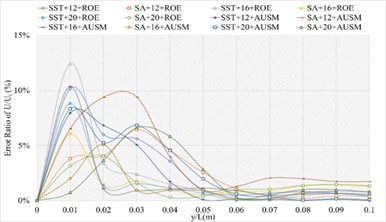
f) Error ratio distribution of U/Ut of the sharp trailing edge based on definition formula (132 points)
3.1.4. Definition formula adopting 264 points
The corresponding numerical results and distributions of P/Pt, T/Tt and U/Ut are shown in Tables 14-16 and Fig. 13. Table 23 provides the mean error ratios under different simulation configurations. From the aspect of far field distance, the minimum values are 2.826 %, 2.758 %, and 3.449 % in order. From the aspect of turbulence model, the most favorable outcome for SST is 2.758 %, while that of SA is 4.511 %. For the two flux types, the finest value of ROE is 2.758 % and that of AUSM is 3.822 %. In conclusion, the SST+16L configuration, combined with ROE has achieved the minimum mean error ratio. Compared with other simulation configurations, the corresponding accuracy improvements are 2.388 %, 27.832 %, 38.853 %, 42.156 %, 78.356 %, 48.882 %, 48.951 %, 20.037 %, 67.078 %, 40.690 %, and 69.247 %, respectively.
Table 14Numerical results of P/Pt of sharp trailing edge based on definition formula (264 points) adopting two turbulence models, three far field distances and two flux types
NACA0012 models | x/L (m) and P/Pt wind tunnel data (P/Pt numerical data) | ||||||||
–0.007 | –0.006 | –0.005 | –0.004 | –0.003 | –0.002 | –0.001 | 0 | ||
103.6285 | 114.5508 | 117.8656 | 119.3983 | 121.7447 | 122.1036 | 123.3942 | 123.8330 | ||
SST+12L | ROE | 119.7998 | 119.7281 | 120.2691 | 122.1656 | 122.9093 | 123.4018 | 123.1883 | 122.8126 |
AUSM | 106.5388 | 105.0963 | 104.8083 | 104.9926 | 105.3805 | 110.8957 | 115.5403 | 123.2807 | |
SA+12L | ROE | 111.6394 | 109.2246 | 109.1835 | 109.8389 | 109.8331 | 118.7383 | 132.1181 | 148.5604 |
AUSM | 117.0965 | 117.1610 | 115.3581 | 114.1848 | 114.3075 | 116.5136 | 120.0602 | 131.7145 | |
SST+16L | ROE | 104.9188 | 114.8591 | 120.1705 | 123.3651 | 124.5840 | 125.6013 | 128.1834 | 130.1437 |
AUSM | 14.2166 | 57.3405 | 121.6925 | 120.0211 | 121.3962 | 123.1041 | 126.7583 | 131.6546 | |
SA+16L | ROE | 113.7103 | 113.9911 | 115.5370 | 115.9321 | 115.4393 | 113.6081 | 102.4842 | 91.1783 |
AUSM | 112.5245 | 111.4754 | 109.5819 | 108.2756 | 109.2258 | 112.8401 | 115.5227 | 120.5992 | |
SST+20L | ROE | 96.4637 | 118.4568 | 124.6484 | 125.9054 | 127.6129 | 129.0491 | 132.1726 | 135.1249 |
AUSM | 108.7255 | 108.7527 | 108.7589 | 108.7113 | 108.6527 | 108.5267 | 107.3855 | 104.1970 | |
SA+20L | ROE | 120.4941 | 120.9398 | 121.3385 | 121.6577 | 121.5770 | 121.3591 | 116.5592 | 114.0342 |
AUSM | 67.4088 | 111.4342 | 132.0675 | 125.9648 | 128.9382 | 132.7707 | 143.0583 | 153.6435 |
Table 15Numerical results of T/Tt of sharp trailing edge based on definition formula (264 points) adopting two turbulence models, three far field distances and two flux types
NACA0012 models | x/L (m) and T/Tt wind tunnel data (T/Tt numerical data) | ||||||||
–0.007 | –0.006 | –0.005 | –0.004 | –0.003 | –0.002 | –0.001 | 0 | ||
18.152 | 20.190 | 20.284 | 20.427 | 20.521 | 20.664 | 21.043 | 21.327 | ||
SST+12L | ROE | 20.265 | 20.399 | 20.523 | 20.751 | 20.862 | 20.961 | 20.889 | 18.934 |
AUSM | 19.513 | 19.793 | 20.230 | 20.351 | 20.493 | 20.652 | 19.449 | 20.337 | |
SA+12L | ROE | 20.653 | 20.674 | 20.662 | 20.620 | 20.616 | 20.787 | 19.673 | 21.209 |
AUSM | 21.407 | 21.550 | 21.067 | 20.836 | 20.643 | 20.455 | 20.339 | 20.312 | |
SST+16L | ROE | 19.961 | 20.385 | 20.629 | 20.749 | 20.733 | 20.661 | 20.186 | 18.623 |
AUSM | 4.826 | 11.009 | 19.659 | 19.813 | 19.921 | 19.972 | 19.857 | 20.080 | |
SA+16L | ROE | 19.189 | 19.449 | 20.030 | 20.322 | 20.471 | 20.266 | 19.039 | 18.611 |
AUSM | 20.044 | 20.421 | 20.526 | 20.373 | 20.137 | 19.901 | 20.219 | 12.186 | |
SST+20L | ROE | 17.859 | 19.600 | 20.384 | 20.631 | 20.707 | 20.658 | 20.402 | 18.762 |
AUSM | 20.354 | 20.487 | 20.628 | 20.896 | 20.986 | 20.840 | 19.309 | 13.045 | |
SA+20L | ROE | 20.566 | 20.638 | 20.702 | 20.772 | 20.770 | 20.691 | 20.248 | 13.594 |
AUSM | 11.876 | 16.908 | 20.229 | 20.249 | 20.367 | 20.476 | 19.791 | 21.239 |
Table 16Numerical results of U/Ut of sharp trailing edge based on definition formula (264 points) adopting two turbulence models, three far field distances and two flux types
NACA0012 models | y/L (m) at x/L= 0.95 m and U/Ut wind tunnel data (U/Ut numerical data) | ||||||||||
0.01 | 0.02 | 0.03 | 0.04 | 0.05 | 0.06 | 0.07 | 0.08 | 0.09 | 0.1 | ||
0.6907 | 0.8199 | 0.8556 | 0.8667 | 0.8705 | 0.8711 | 0.8740 | 0.8746 | 0.8771 | 0.8801 | ||
SST+12L | ROE | 0.6410 | 0.8225 | 0.8565 | 0.8686 | 0.8747 | 0.8778 | 0.8798 | 0.8823 | 0.8851 | 0.8868 |
AUSM | 0.6903 | 0.8497 | 0.8722 | 0.8704 | 0.8689 | 0.8708 | 0.8734 | 0.8766 | 0.8795 | 0.8811 | |
SA+12L | ROE | 0.7094 | 0.8025 | 0.8217 | 0.8538 | 0.8764 | 0.8850 | 0.8868 | 0.8865 | 0.8868 | 0.8870 |
AUSM | 0.6704 | 0.7601 | 0.7867 | 0.8084 | 0.8293 | 0.8443 | 0.8526 | 0.8581 | 0.8615 | 0.8633 | |
SST+16L | ROE | 0.6214 | 0.8083 | 0.8430 | 0.8569 | 0.8646 | 0.8702 | 0.8743 | 0.8787 | 0.8826 | 0.8849 |
AUSM | 0.6101 | 0.8230 | 0.8696 | 0.8692 | 0.8699 | 0.8727 | 0.8755 | 0.8792 | 0.8831 | 0.8855 | |
SA+16L | ROE | 0.6535 | 0.7837 | 0.7866 | 0.8165 | 0.8505 | 0.8676 | 0.8753 | 0.8814 | 0.8857 | 0.8881 |
AUSM | 0.6940 | 0.8642 | 0.8756 | 0.8650 | 0.8656 | 0.8721 | 0.8776 | 0.8826 | 0.8859 | 0.8877 | |
SST+20L | ROE | 0.6307 | 0.8099 | 0.8438 | 0.8580 | 0.8660 | 0.8718 | 0.8761 | 0.8809 | 0.8850 | 0.8874 |
AUSM | 0.6090 | 0.7029 | 0.7321 | 0.7638 | 0.7972 | 0.8300 | 0.8515 | 0.8658 | 0.8733 | 0.8771 | |
SA+20L | ROE | 0.6877 | 0.8064 | 0.8123 | 0.8399 | 0.8625 | 0.8713 | 0.8750 | 0.8784 | 0.8814 | 0.8833 |
AUSM | 0.6916 | 0.7442 | 0.7411 | 0.7565 | 0.7934 | 0.8282 | 0.8475 | 0.8582 | 0.8653 | 0.8693 |
Fig. 13The numerical result and error ratio distributions of P/Pt, T/Tt and U/Ut of sharp trailing edge based on definition formula (264 points)
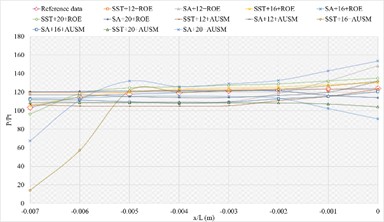
a) Numerical result distribution of P/Pt of the sharp trailing edge based on definition formula (264 points)
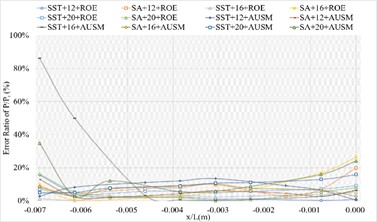
b) Error ratio distribution of P/Pt of the sharp trailing edge based on definition formula (264 points)
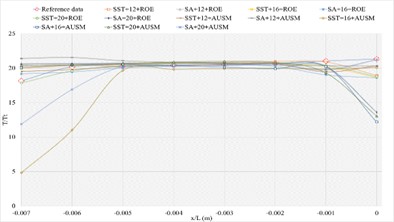
c) Numerical result distribution of T/Tt of the sharp trailing edge based on definition formula (264 points)
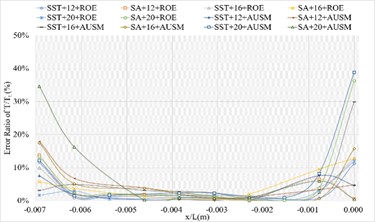
d) Error ratio distribution of T/Tt of the sharp trailing edge based on definition formula (264 points)
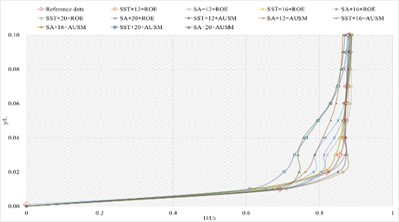
e) Numerical result distribution of U/Ut of the sharp trailing edge based on definition formula (264 points)
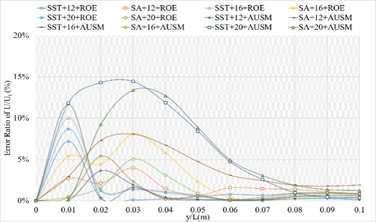
f) Error ratio distribution of U/Ut of the sharp trailing edge based on definition formula (264 points)
3.1.5. Definition formula adopting 200 points
The corresponding numerical results and distributions of P/Pt, T/Tt and U/Ut are shown in Tables 17-19 and Fig. 14. Table 23 provides the mean error ratios under different simulation configurations. From the aspect of far field distance, the minimum values are 2.866 %, 2.047 %, and 3.376 % in order. From the aspect of turbulence model, the most favorable outcome for SST is 3.264 %, while that of SA is 2.047 %. For the two flux types, the finest value of ROE is 2.047 % and that of AUSM is 3.264 %. In conclusion, the SA+16L configuration, combined with ROE has achieved the minimum mean error ratio. Compared with other simulation configurations, the corresponding accuracy improvements are 36.670 %, 65.361 %, 28.590 %, 56.800 %, 66.338 %, 37.299 %, 37.549 %, 54.702 %, 64.219 %, 46.723 %, and 39.367 %, respectively.
Table 17Numerical results of P/Pt of sharp trailing edge based on definition formula (200 points) adopting two turbulence models, three far field distances and two flux types
NACA0012 models | x/L (m) and P/Pt wind tunnel data (P/Pt numerical data) | ||||||||
–0.007 | –0.006 | –0.005 | –0.004 | –0.003 | –0.002 | –0.001 | 0 | ||
103.6285 | 114.5508 | 117.8656 | 119.3983 | 121.7447 | 122.1036 | 123.3942 | 123.8330 | ||
SST+12L | ROE | 95.2490 | 121.0420 | 122.3321 | 123.1870 | 124.9979 | 126.5275 | 129.6336 | 132.2661 |
AUSM | 129.6510 | 127.9943 | 126.6424 | 127.8131 | 128.1751 | 127.3835 | 125.5274 | 122.0080 | |
SA+12L | ROE | 88.1361 | 106.7947 | 112.6416 | 113.1061 | 114.1654 | 117.0814 | 120.5537 | 127.4284 |
AUSM | 92.6078 | 127.9824 | 128.0527 | 127.0169 | 128.0833 | 129.2793 | 131.2632 | 133.4659 | |
SST+16L | ROE | 70.0133 | 107.5888 | 126.7207 | 128.4036 | 128.3239 | 127.5474 | 127.2913 | 126.4086 |
AUSM | 123.2248 | 121.5232 | 118.3046 | 119.8646 | 121.7615 | 123.6976 | 125.7666 | 124.9552 | |
SA+16L | ROE | 101.5952 | 111.8097 | 117.2720 | 117.7607 | 118.0276 | 118.0976 | 117.8711 | 119.7389 |
AUSM | 119.8220 | 114.3523 | 111.4593 | 112.6008 | 114.4394 | 119.3344 | 121.2641 | 122.1085 | |
SST+20L | ROE | 101.8103 | 112.8437 | 115.7255 | 114.2609 | 114.3502 | 113.7401 | 110.6038 | 114.4976 |
AUSM | 129.1016 | 125.3428 | 123.7512 | 125.0469 | 126.9627 | 128.7558 | 133.7061 | 144.7895 | |
SA+20L | ROE | 121.1185 | 120.9658 | 120.7203 | 120.3242 | 119.6663 | 118.7319 | 115.1396 | 110.0396 |
AUSM | 119.1405 | 120.4283 | 120.6966 | 122.0053 | 122.8732 | 123.2574 | 123.3283 | 121.9717 |
Table 18Numerical results of T/Tt of sharp trailing edge based on definition formula (200 points) adopting two turbulence models, three far field distances and two flux types
NACA0012 models | x/L (m) and T/Tt wind tunnel data (T/Tt numerical data) | ||||||||
–0.007 | –0.006 | –0.005 | –0.004 | –0.003 | –0.002 | –0.001 | 0 | ||
18.152 | 20.190 | 20.284 | 20.427 | 20.521 | 20.664 | 21.043 | 21.327 | ||
SST+12L | ROE | 16.551 | 19.384 | 20.448 | 20.622 | 20.666 | 20.651 | 20.270 | 18.759 |
AUSM | 20.727 | 21.044 | 21.272 | 21.549 | 21.585 | 20.283 | 19.882 | 16.591 | |
SA+12L | ROE | 17.981 | 19.476 | 20.274 | 20.411 | 20.527 | 20.688 | 20.812 | 20.568 |
AUSM | 14.238 | 19.106 | 20.805 | 20.829 | 20.869 | 20.741 | 20.767 | 20.866 | |
SST+16L | ROE | 11.797 | 17.598 | 20.550 | 21.057 | 21.280 | 21.064 | 20.717 | 20.642 |
AUSM | 19.688 | 20.028 | 20.234 | 20.448 | 20.627 | 20.889 | 21.297 | 17.054 | |
SA+16L | ROE | 19.274 | 19.891 | 20.245 | 20.439 | 20.516 | 20.648 | 20.695 | 20.217 |
AUSM | 20.448 | 20.500 | 20.518 | 20.514 | 20.404 | 20.293 | 20.064 | 19.781 | |
SST+20L | ROE | 17.608 | 18.326 | 18.700 | 18.845 | 19.056 | 19.702 | 19.834 | 19.830 |
AUSM | 20.882 | 21.048 | 21.204 | 21.310 | 21.056 | 20.465 | 20.164 | 20.226 | |
SA+20L | ROE | 20.707 | 20.751 | 20.773 | 20.766 | 20.769 | 20.760 | 20.145 | 19.683 |
AUSM | 18.929 | 19.318 | 19.726 | 19.914 | 20.181 | 20.654 | 20.752 | 17.893 |
Table 19Numerical results of U/Ut of sharp trailing edge based on definition formula (200 points) adopting two turbulence models, three far field distances and two flux types
NACA0012 models | y/L (m) at x/L= 0.95 m and U/Ut wind tunnel data (U/Ut numerical data) | ||||||||||
0.01 | 0.02 | 0.03 | 0.04 | 0.05 | 0.06 | 0.07 | 0.08 | 0.09 | 0.1 | ||
0.6907 | 0.8199 | 0.8556 | 0.8667 | 0.8705 | 0.8711 | 0.8740 | 0.8746 | 0.8771 | 0.8801 | ||
SST+12L | ROE | 0.6634 | 0.8368 | 0.8552 | 0.8629 | 0.8684 | 0.8730 | 0.8766 | 0.8808 | 0.8844 | 0.8866 |
AUSM | 0.6104 | 0.8114 | 0.8678 | 0.8737 | 0.8693 | 0.8687 | 0.8720 | 0.8782 | 0.8843 | 0.8877 | |
SA+12L | ROE | 0.7054 | 0.8717 | 0.8779 | 0.8770 | 0.8751 | 0.8748 | 0.8762 | 0.8796 | 0.8837 | 0.8866 |
AUSM | 0.7205 | 0.8669 | 0.8665 | 0.8694 | 0.8760 | 0.8803 | 0.8825 | 0.8848 | 0.8872 | 0.8887 | |
SST+16L | ROE | 0.6519 | 0.8574 | 0.8755 | 0.8749 | 0.8743 | 0.8756 | 0.8784 | 0.8830 | 0.8877 | 0.8905 |
AUSM | 0.6017 | 0.8144 | 0.8655 | 0.8726 | 0.8749 | 0.8764 | 0.8785 | 0.8823 | 0.8863 | 0.8889 | |
SA+16L | ROE | 0.7092 | 0.8739 | 0.8681 | 0.8692 | 0.8746 | 0.8796 | 0.8830 | 0.8863 | 0.8891 | 0.8908 |
AUSM | 0.6745 | 0.8656 | 0.8790 | 0.8745 | 0.8693 | 0.8685 | 0.8698 | 0.8734 | 0.8780 | 0.8816 | |
SST+20L | ROE | 0.6450 | 0.8527 | 0.8731 | 0.8746 | 0.8760 | 0.8791 | 0.8821 | 0.8857 | 0.8889 | 0.8909 |
AUSM | 0.5981 | 0.7864 | 0.8374 | 0.8651 | 0.8692 | 0.8700 | 0.8726 | 0.8775 | 0.8826 | 0.8858 | |
SA+20L | ROE | 0.6860 | 0.8558 | 0.8776 | 0.8731 | 0.8713 | 0.8733 | 0.8767 | 0.8819 | 0.8868 | 0.8898 |
AUSM | 0.7078 | 0.7781 | 0.8094 | 0.8215 | 0.8393 | 0.8573 | 0.8664 | 0.8720 | 0.8762 | 0.8786 |
Fig. 14The numerical result and error ratio distributions of P/Pt, T/Tt and U/Ut of sharp trailing edge based on definition formula (200 points)
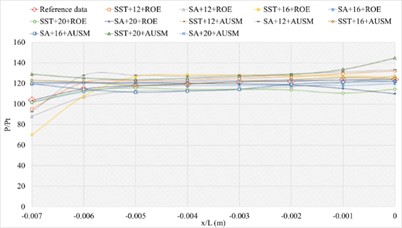
a) Numerical result distribution of P/Pt of the sharp trailing edge based on definition formula (200 points)
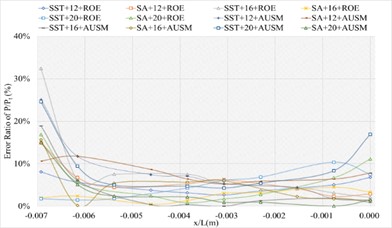
b) Error ratio distribution of P/Pt of the sharp trailing edge based on definition formula (200 points)
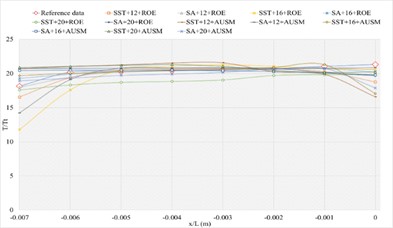
c) Numerical result distribution of T/Tt of the sharp trailing edge based on definition formula (200 points)
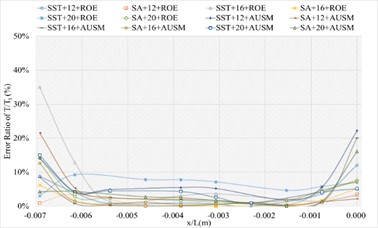
d) Error ratio distribution of T/Ttof the sharp trailing edge based on definition formula (200 points)
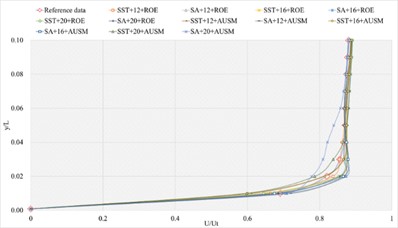
e) Numerical result distribution of U/Ut of the sharp trailing edge based on definition formula (200 points)
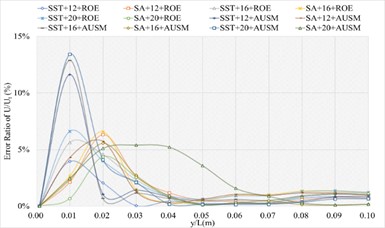
f) Error ratio distribution of U/Ut of the sharp trailing edge based on definition formula (200 points)
3.1.6. Definition formula adopting 400 points
The corresponding numerical results and distributions of P/Pt, T/Tt and U/Ut are shown in Tables 20-22 and Fig. 15. Table 23 provides the calculated mean error ratios under different simulation configurations. From the aspect of far field distance, the minimum values are 4.136 %, 2.460 %, and 2.454 % in order. From the aspect of turbulence model, the most favorable outcome for SST is 2.454 %, while that of SA is 3.482 %. For the two flux types, the finest value of ROE is 2.454 % and that of AUSM is 3.482 %. In conclusion, the SST+20L configuration, combined with ROE has achieved the minimum mean error ratio. Compared with other simulation configurations, the corresponding accuracy improvements are 40.670 %, 80.420 %, 56.911 %, 71.324 %, 0.240 %, 61.911 %, 33.899 %, 29.537 %, 40.134 %, 36.977 %, and 59.327 %, respectively.
Table 20Numerical results of P/Pt of sharp trailing edge based on definition formula (400 points) adopting two turbulence models, three far field distances and two flux types
NACA0012 models | x/L (m) and P/Pt wind tunnel data (P/Pt numerical data) | ||||||||
–0.007 | –0.006 | –0.005 | –0.004 | –0.003 | –0.002 | –0.001 | 0 | ||
103.6285 | 114.5508 | 117.8656 | 119.3983 | 121.7447 | 122.1036 | 123.3942 | 123.8330 | ||
SST+12L | ROE | 121.5352 | 121.5892 | 121.8436 | 122.3692 | 122.4129 | 122.2378 | 116.3225 | 108.3289 |
AUSM | 104.5392 | 104.3224 | 104.0669 | 103.6346 | 103.5846 | 103.5758 | 98.7789 | 94.0892 | |
SA+12L | ROE | 120.7982 | 120.4667 | 120.3445 | 119.5408 | 117.6898 | 114.7833 | 100.4419 | 102.2569 |
AUSM | 106.9614 | 106.0633 | 105.1678 | 103.4016 | 102.6120 | 101.9376 | 101.4844 | 103.5008 | |
SST+16L | ROE | 103.1487 | 114.1292 | 119.7196 | 122.8894 | 124.0935 | 125.0836 | 127.5916 | 129.4911 |
AUSM | 119.4194 | 119.4679 | 118.3171 | 116.7076 | 114.0483 | 110.7948 | 107.6613 | 109.6274 | |
SA+16L | ROE | 84.7344 | 105.5092 | 115.0007 | 116.0551 | 119.1564 | 123.3079 | 135.0119 | 138.4577 |
AUSM | 115.7716 | 116.3642 | 117.5177 | 118.0496 | 118.5284 | 119.1038 | 118.8542 | 116.6833 | |
SST+20L | ROE | 101.7360 | 113.5344 | 119.1353 | 122.2907 | 123.5090 | 124.5382 | 126.9285 | 128.7087 |
AUSM | 111.4175 | 120.3351 | 118.6102 | 120.8620 | 125.6442 | 130.3150 | 137.6943 | 143.5232 | |
SA+20L | ROE | 85.6777 | 105.7869 | 113.6548 | 114.4745 | 117.0368 | 120.6551 | 134.5757 | 138.7417 |
AUSM | 86.0844 | 105.3288 | 117.5366 | 116.7220 | 118.1160 | 123.6655 | 136.1005 | 146.2670 |
Table 21Numerical results of T/Tt of sharp trailing edge based on definition formula (400 points) adopting two turbulence models, three far field distances and two flux types
NACA0012 models | x/L (m) and T/Tt wind tunnel data (T/Tt numerical data) | ||||||||
–0.007 | –0.006 | –0.005 | –0.004 | –0.003 | –0.002 | –0.001 | 0 | ||
18.152 | 20.190 | 20.284 | 20.427 | 20.521 | 20.664 | 21.043 | 21.327 | ||
SST+12L | ROE | 20.476 | 20.586 | 20.682 | 20.862 | 20.944 | 20.889 | 20.056 | 18.964 |
AUSM | 19.928 | 20.157 | 20.330 | 20.269 | 19.400 | 16.884 | 8.214 | 3.902 | |
SA+12L | ROE | 20.513 | 20.606 | 20.155 | 19.781 | 19.622 | 19.593 | 19.235 | 18.366 |
AUSM | 18.219 | 18.129 | 18.075 | 18.023 | 18.011 | 18.089 | 18.158 | 16.021 | |
SST+16L | ROE | 19.882 | 20.374 | 20.645 | 20.770 | 20.751 | 20.675 | 20.176 | 18.464 |
AUSM | 21.466 | 21.187 | 20.172 | 19.808 | 19.637 | 19.458 | 19.065 | 14.682 | |
SA+16L | ROE | 17.053 | 18.914 | 20.053 | 20.139 | 20.244 | 20.500 | 20.609 | 20.531 |
AUSM | 20.506 | 20.677 | 20.858 | 21.126 | 21.139 | 20.820 | 20.126 | 19.176 | |
SST+20L | ROE | 19.831 | 20.385 | 20.670 | 20.797 | 20.776 | 20.697 | 20.190 | 18.259 |
AUSM | 18.831 | 19.597 | 20.131 | 20.380 | 20.680 | 20.935 | 21.276 | 20.721 | |
SA+20L | ROE | 17.312 | 19.017 | 19.999 | 20.080 | 20.182 | 20.470 | 20.599 | 20.551 |
AUSM | 17.093 | 18.685 | 19.730 | 19.768 | 19.813 | 19.906 | 19.975 | 12.296 |
Table 22Numerical results of U/Ut of sharp trailing edge based on definition formula (400 points) adopting two turbulence models, three far field distances and two flux types
NACA0012 models | y/L (m) at x/L= 0.95 m and U/Ut wind tunnel data (U/Ut numerical data) | ||||||||||
0.01 | 0.02 | 0.03 | 0.04 | 0.05 | 0.06 | 0.07 | 0.08 | 0.09 | 0.1 | ||
0.6907 | 0.8199 | 0.8556 | 0.8667 | 0.8705 | 0.8711 | 0.8740 | 0.8746 | 0.8771 | 0.8801 | ||
SST+12L | ROE | 0.6754 | 0.8645 | 0.8788 | 0.8759 | 0.8754 | 0.8778 | 0.8805 | 0.8837 | 0.8867 | 0.8885 |
AUSM | 0.6956 | 0.8018 | 0.7984 | 0.8265 | 0.8641 | 0.8782 | 0.8813 | 0.8818 | 0.8821 | 0.8823 | |
SA+12L | ROE | 0.7301 | 0.8741 | 0.8743 | 0.8742 | 0.8761 | 0.8785 | 0.8806 | 0.8834 | 0.8863 | 0.8880 |
AUSM | 0.7055 | 0.8441 | 0.8732 | 0.8773 | 0.8712 | 0.8689 | 0.8696 | 0.8732 | 0.8779 | 0.8809 | |
SST+16L | ROE | 0.6532 | 0.8335 | 0.8533 | 0.8617 | 0.8677 | 0.8725 | 0.8763 | 0.8805 | 0.8844 | 0.8867 |
AUSM | 0.5980 | 0.8120 | 0.8621 | 0.8703 | 0.8747 | 0.8769 | 0.8778 | 0.8797 | 0.8828 | 0.8850 | |
SA+16L | ROE | 0.6972 | 0.8703 | 0.8708 | 0.8670 | 0.8689 | 0.8743 | 0.8789 | 0.8833 | 0.8870 | 0.8892 |
AUSM | 0.7106 | 0.8780 | 0.8699 | 0.8702 | 0.8764 | 0.8799 | 0.8816 | 0.8844 | 0.8878 | 0.8900 | |
SST+20L | ROE | 0.6505 | 0.8288 | 0.8516 | 0.8607 | 0.8669 | 0.8721 | 0.8762 | 0.8808 | 0.8850 | 0.8875 |
AUSM | 0.6226 | 0.7503 | 0.7943 | 0.8283 | 0.8415 | 0.8480 | 0.8542 | 0.8626 | 0.8699 | 0.8743 | |
SA+20L | ROE | 0.6937 | 0.8678 | 0.8749 | 0.8770 | 0.8783 | 0.8798 | 0.8816 | 0.8844 | 0.8877 | 0.8898 |
AUSM | 0.7392 | 0.8062 | 0.8433 | 0.8700 | 0.8778 | 0.8755 | 0.8724 | 0.8716 | 0.8730 | 0.8741 |
Fig. 15The numerical result and error ratio distributions of P/Pt, T/Tt and U/Ut of sharp trailing edge based on definition formula (400 points)
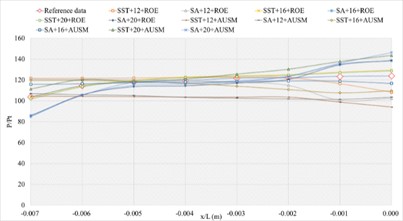
a) Numerical result distribution of P/Pt of the sharp trailing edge based on definition formula (400 points)
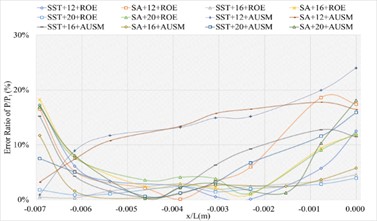
b) Error ratio distribution of P/Pt of the sharp trailing edge based on definition formula (400 points)
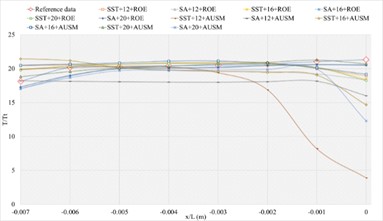
c) Numerical result distribution of T/Tt of the sharp trailing edge based on definition formula (400 points)
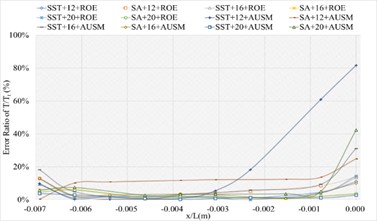
d) Error ratio distribution of T/Tt of the sharp trailing edge based on definition formula (400 points)
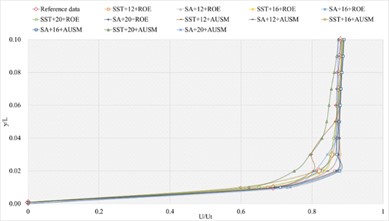
e) Numerical result distribution of U/Ut of the sharp trailing edge based on definition formula (400 points)
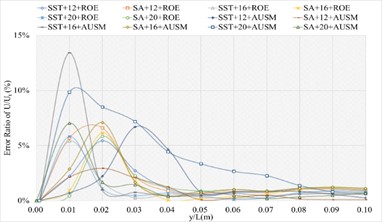
f) Error ratio distribution of U/Ut of the sharp trailing edge based on definition formula (400 points)
Table 23The mean error ratios for the six NACA0012 models under different simulation configurations
Simulation configuration | NACA0012 models | ROE (%) | AUSM (%) | ||||||
P/Pt | T/Tt | U/Ut | Total mean error ratio of (P/Pt, T/Tt, U/Ut) | P/Pt | T/Tt | U/Ut | Total mean error ratio of (P/Pt, T/Tt, U/Ut) | ||
SST+12L | Blunt | 1.968 | 4.523 | 1.598 | 2.696 | 8.939 | 5.909 | 4.072 | 6.307 |
NACA4 | 2.276 | 3.962 | 1.035 | 2.424 | 10.415 | 8.540 | 4.376 | 7.777 | |
Definition formula (132 points) | 16.132 | 9.272 | 2.950 | 9.451 | 4.316 | 2.877 | 2.214 | 3.136 | |
Definition formula (264 points) | 3.437 | 3.811 | 1.230 | 2.826 | 7.955 | 2.814 | 0.697 | 3.822 | |
Definition formula (200 points) | 4.860 | 3.881 | 0.955 | 3.232 | 8.019 | 7.942 | 1.766 | 5.909 | |
Definition formula (400 points) | 6.025 | 4.722 | 1.660 | 4.136 | 13.597 | 22.172 | 1.826 | 12.532 | |
SST+16L | Blunt | 2.197 | 4.485 | 1.532 | 2.738 | 8.155 | 13.631 | 5.249 | 9.012 |
Naca4 | 6.228 | 3.245 | 2.357 | 3.943 | 5.962 | 8.189 | 2.072 | 5.408 | |
Definition formula (132 points) | 2.137 | 4.212 | 1.569 | 2.640 | 2.779 | 6.139 | 1.781 | 3.566 | |
Definition formula (264 points) | 2.621 | 4.002 | 1.652 | 2.758 | 18.768 | 17.841 | 1.622 | 12.744 | |
Definition formula (200 points) | 8.584 | 7.830 | 1.827 | 6.080 | 3.738 | 4.059 | 1.996 | 3.264 | |
Definition formula (400points) | 2.209 | 4.077 | 1.093 | 2.460 | 7.746 | 9.685 | 1.893 | 6.442 | |
SST+20L | Blunt | 5.272 | 3.356 | 1.681 | 3.436 | 9.471 | 6.024 | 2.860 | 6.119 |
NACA4 | 3.148 | 4.142 | 2.276 | 3.189 | 15.305 | 7.999 | 2.384 | 8.562 | |
Definition formula (132 points) | 11.002 | 4.356 | 1.612 | 5.657 | 4.140 | 4.644 | 2.830 | 3.871 | |
Definition formula (264 points) | 6.034 | 2.755 | 1.560 | 3.449 | 9.670 | 8.474 | 6.990 | 8.378 | |
Definition formula (200 points) | 5.024 | 6.543 | 1.988 | 4.518 | 9.843 | 5.132 | 2.186 | 5.720 | |
Definition formula (400 points) | 2.057 | 4.222 | 1.082 | 2.454 | 6.480 | 1.713 | 4.103 | 4.099 | |
SA+12L | Blunt | 24.038 | 24.609 | 2.394 | 17.014 | 3.619 | 5.559 | 1.145 | 3.441 |
NACA4 | 4.422 | 2.164 | 1.901 | 2.829 | 8.525 | 3.545 | 1.404 | 4.491 | |
Definition formula (132 points) | 8.581 | 9.701 | 2.410 | 6.897 | 8.912 | 6.314 | 3.912 | 6.379 | |
Definition formula (264 points) | 8.416 | 3.388 | 1.728 | 4.511 | 5.190 | 5.030 | 4.085 | 4.768 | |
Definition formula (200 points) | 5.871 | 1.176 | 1.552 | 2.866 | 7.828 | 4.626 | 1.759 | 4.738 | |
Definition formula (400 points) | 8.663 | 6.363 | 2.057 | 5.694 | 12.650 | 12.065 | 0.954 | 8.556 | |
SA+16L | Blunt | 3.519 | 4.375 | 1.980 | 3.292 | 5.893 | 6.107 | 3.625 | 5.209 |
NACA4 | 5.325 | 5.244 | 1.610 | 4.060 | 5.116 | 4.569 | 1.707 | 3.797 | |
Definition formula (132 points) | 11.850 | 8.200 | 1.664 | 7.238 | 7.323 | 5.833 | 1.261 | 4.805 | |
Definition formula (264 points) | 8.819 | 4.448 | 2.921 | 5.396 | 6.809 | 8.172 | 1.229 | 5.403 | |
Definition formula (200 points) | 2.543 | 1.859 | 1.738 | 2.047 | 4.789 | 3.754 | 1.289 | 3.277 | |
Definition formula (400 points) | 6.962 | 2.857 | 1.317 | 3.712 | 3.660 | 4.980 | 1.806 | 3.482 | |
SA+20L | Blunt | 6.399 | 2.696 | 2.007 | 3.701 | 7.272 | 1.680 | 2.220 | 3.724 |
Naca4 | 4.685 | 4.283 | 1.728 | 3.565 | 15.453 | 9.744 | 2.061 | 9.086 | |
Definition formula (132 points) | 1.760 | 5.580 | 1.586 | 2.975 | 13.734 | 7.500 | 2.340 | 7.858 | |
Definition formula (264 points) | 5.111 | 7.582 | 1.259 | 4.651 | 13.734 | 7.500 | 5.673 | 8.969 | |
Definition formula (200 points) | 5.997 | 4.323 | 1.206 | 3.842 | 3.514 | 4.132 | 2.481 | 3.376 | |
Definition formula (400 points) | 7.353 | 2.735 | 1.592 | 3.893 | 7.522 | 9.223 | 1.353 | 6.033 |
3.2. Discussion
3.2.1. Cell Reynolds number and aspect ratio
The cell Reynolds number (Rcell) of near-wall mesh cells close to the shock are crucial in affecting the numerical error ratio. Taking blunt cylinder as the characteristic object, Ref. [30] points out that the Rcell value of the near shock wave grid cells should be no less than 8. Moreover, Ref. [31] shows that the aspect ratio of wall cells near the shock significantly impacts simulation performance. In this paper, based on the existing research, to investigate the influences of Rcell and aspect ratio of the near shock wave wall grid cells with NACA0012 as the characteristic object, the Rcell of near shock wave wall grid cells is first analyzed while keeping the total mesh number unchanged. Based on the optimal simulation configuration conclude in Section 3.1, we apply three Rcell values of 16, 8, and 4 and the related y+ and yHvalues are (0.3 1.4e-5 m), (0.15 7e-6 m), and (0.08 3.5e-6 m). Three numerical simulations are performed and the corresponding mean error ratios of (P/Pt, T/Tt, U/Ut) are (2.54 % 1.86 % 1.74 %), (2.62 % 2.09 % 2.01 %), and (6.38 % 4.77 % 2.47 %) respectively, as shown in Table 24. Therefore, the optimal results are obtained by Rcell value of 16. Next, to study the influence of aspect ratio on numerical accuracy, we made changes to the wall cells' aspect ratio near the shock while keeping the following conditions constant: (1) The total number of mesh cells remained the same. (2) The cell Reynolds number remained the same. (3) The aspect ratio changes were made only in small wall regions near the shock. The aspect ratio value of the near-wall mesh close to the shock at the optimal Rcell value is 380, through double and halve operations we select four aspect ratio values of 760, 380, 190, and 95. Another four simulations are executed and the corresponding comparison of error ratios are described in Table 25. When the aspect ratio is 380, the minimum simulation result is achieved, which is 2.05 %. Then with the further increase of the aspect ratio, the error ratio is also increased. Compared with the other three aspect ratios, the accuracy improvements are 63.97 %, 46.75 % and 65.37 %. In summarize, unlike the suggestions proposed in the existing research characterized by blunt cylinder, the suitable value for Rcell characterized by NACA0012 should be no smaller than 16, reducing this value will decrease numerical accuracy. Similar situation applies to the aspect ratio, smaller value would not lead to better numerical calculation and the recommended value is 380.
Table 24Comparison of numerical error ratios under three cell Reynolds numbers
Cell Reynolds number | P/Pt | T/Tt | U/Ut | Mean error ratio |
16 (y+=0.3) | 2.54 % | 1.86 % | 1.74 % | 2.05 % |
8 (y+=0.15) | 2.62 % | 2.09 % | 2.01 % | 2.24 % |
4 (y+=0.08) | 6.38 % | 4.77 % | 2.47 % | 4.54 % |
Table 25Comparison of numerical error ratios under four aspect ratios
Aspect ratio | P/Pt | T/Tt | U/Ut | Mean error ratio | Accuracy improvement among aspect ratios |
95 | 4.78 % | 6.26 % | 6.04 % | 5.69 % | 63.97 % |
190 | 4.35 % | 3.49 % | 3.71 % | 3.85 % | 46.75 % |
380 | 2.54 % | 1.86 % | 1.74 % | 2.05 % | 0.00 % |
760 | 8.56 % | 4.07 % | 5.13 % | 5.92 % | 65.37 % |
3.3. Trailing edge shape and modeling method
Fig. 16 depicts the optimal numerical error ratios comparison among six NACA0012 model, where the left ordinate indicates the numerical error ratio displayed in a column graph, and the right ordinate indicates the accuracy improvement displayed in a line chart. Fig. 17 adopts the same settings. From the aspect of trailing edge shape, based on Airfoil tools, the designed blunt trailing edge’s numerical performance is worse than that of other types of sharp trailing edge (the sharp trailing edge adopting 264 data points definition formula is excluded), with the numerical accuracy decreasing by 11.41 %, 2.14 %, 31.73 % and 9.88 %, respectively. For the three modeling methods, the corresponding finest numerical error ratio is 2.7 %, 2.42 % and 2.05 % in order. It is worth noting that although the smallest error ratio could be obtained using the definition formula of 200 data points, the numerical results of the airfoil designed based on NACA4 are better in other cases. The correlation between the number and source of data points of the definition formula and the calculation precision is further analyzed. Airfoil tools offers 132 data points, with the data points increasing to 264, the numerical accuracy decreases by 4.55 %. NACA4 offers 200 data points, and the increase in data amount also results in a decrease in numerical accuracy of 19.71 %. When using 200 and 400 data points, the optimal numerical error ratios are 2.05 % and 2.454 %, respectively. These ratios are superior to those based on 132 and 264 data points. In summarize, unlike existing research conclusions [28-29], firstly, there exists a significant decrease in accuracy may occur due to an incorrect shape of the trailing edge in NACA0012, the maximum value of which is up to 31.73 %. The sharp trailing edge is recommended, and the number of data points adopted for NACA0012 modeling is the key to the selection of definition formula or NACA4. Secondly, the performance of data points provided by NACA4 is superior to that provided by Airfoil tools, and there is no positive correlation between the data points number and the calculation accuracy. Lastly, it is recommended to use the definition formula that utilizes NACA4's 200 points to design the sharp trailing edge shape.
3.3.1. Far field distance, turbulence model and flux type
Fig. 17 depicts the optimal numerical error ratios comparison among far field distances, turbulence models and flux types. As to the far field distance, the numerical precision increases by 15.42 %, with the far field distance increasing from 12L to 16L. But with the far field distance reaching 20L, the simulation precision declines by 19.88 %. When considering the turbulence model, the SST k-omega model proves to be more effective than the SA model at far field distances of 12L and 20L.
Fig. 16The optimal numerical error ratio comparison among six NACA0012 models
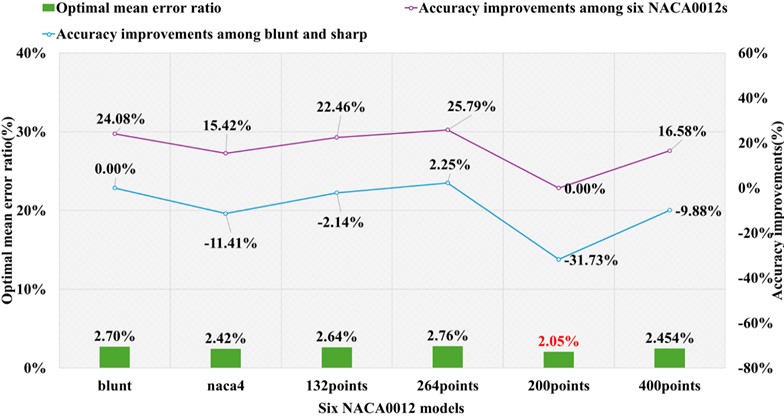
Fig. 17The optimal numerical error ratio comparison among far field distances, turbulence models, and flux types
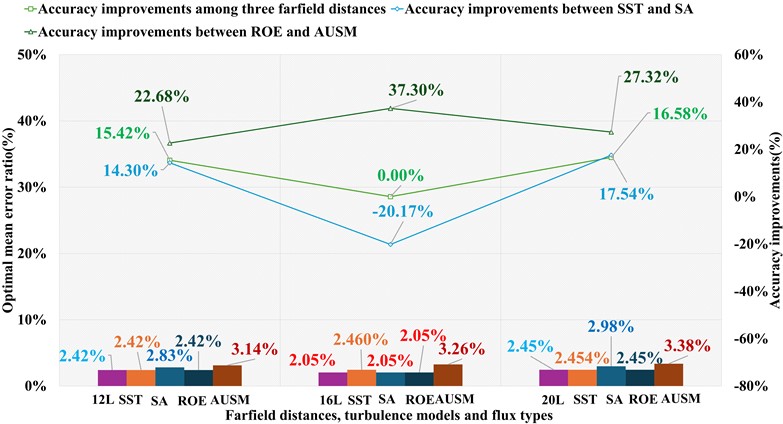
The numerical accuracy is also enhanced by 14.3 % and 17.54 %, respectively. However, at 16L far field distance, the SA model obtains the smallest error ratio. From the perspective of flux type, compared with AUSM, the numerical performance of ROE is better. The maximum increase is 37.3 %, and the minimum increase is 22.68 %. In summary, unlike the research conclusions in Ref. [29], firstly, the increase in far field distance is not necessarily positively correlated with the calculation accuracy. Keeping 16L far field distance is recommended. Secondly, the turbulence model selection is associated with the distance of the far field, and according to the ideal value of far field distance, it is recommended to prioritize the SA model. Lastly, the ROE flux type is preferred. Therefore, under hypersonic conditions, the preferred simulation configurations of NACA0012 are the sharp trailing edge (definition formula adopting 200 data points) + 16L + SA turbulence model + ROE, with the Rcell and aspect ratio values of near-wall mesh near the shock are 16 and 380.
4. Simulation scheme and aerodynamic environment prediction
To simplify the analysis, it is assumed that the flight speed is increased uniformly, and the acceleration process could be completed instantaneously without considering the influence of fuel and engine performance. According to the flight path described in Fig. 1, the flight process is divided into sub-phases in seconds. Maintain a constant speed within each sub-phase and complete the acceleration process instantly when entering the next sub-phase. The hypersonic conditions can be divided into 11 sub-phases, which first undergo the accelerated flight for 10 s (hypersonic 1-hypersonic 10), and then maintain the steady flight at the same height and speed when the flow velocity reaches 6.5 Ma (hypersonic 11). According to the conclusion drawn from the detailed discussion in Section 3.2, an effective simulation scheme for the aerodynamic environment prediction under hypersonic conditions characterized by NACA0012 is shown in Table 26. The applied computational external flow field is shown in Fig. 3(a).
Table 26The simulation scheme under hypersonic conditions characterized by NACA0012
Simulation scheme | Values | |
Hypersonic conditions | hypersonic1 M = 5.100 Pt = 3467 Pa Tt = 219.65 K μ = 1.438e-5 Pa·s ρ = 0.055 kg/m3 | |
hypersonic2 M = 5.250 Pt = 3218 Pa Tt = 220.15 K μ = 1.441e-5 Pa·s ρ = 0.051 kg/m3 | ||
hypersonic3 M = 5.400 Pt = 2972 Pa Tt = 220.65 K μ = 1.444e-5 Pa·s ρ = 0.047 kg/m3 | ||
hypersonic4 M = 5.550 Pt = 2753 Pa Tt = 221.15 K μ = 1.446e-5 Pa·s ρ = 0.043 kg/m3 | ||
hypersonic5 M = 5.700 Pt = 2549 Pa Tt = 221.65 K μ = 1.449e-5 Pa·s ρ = 0.040 kg/m3 | ||
hypersonic6 M = 5.850 Pt = 2361 Pa Tt = 222.15 K μ = 1.452e-5 Pa·s ρ = 0.037 kg/m3 | ||
hypersonic7 M = 6.000 Pt = 2188 Pa Tt = 222.65 K μ = 1.454e-5 Pa·s ρ = 0.034 kg/m3 | ||
hypersonic8 M = 6.125 Pt = 1880 Pa Tt = 223.54 K μ = 1.459e-5 Pa·s ρ = 0.029 kg/m3 | ||
hypersonic9 M = 6.250 Pt = 1610 Pa Tt = 224.53 K μ = 1.465e-5 Pa·s ρ = 0.025 kg/m3 | ||
hypersonic10 M = 6.375 Pt = 1390 Pa Tt = 225.52 K μ = 1.470e-5 Pa·s ρ = 0.021 kg/m3 | ||
hypersonic11 M = 6.500 Pt = 1197 Pa Tt = 226.51 K μ = 1.475e-5 Pa·s ρ = 0.018 kg/m3 | ||
Grid strategy | hypersonic1 Cair = 297 m/s Ut = 1516 m/s y+= 0.3 (Rcell= 16) yH= 2e-6 m as ratio = 380 | |
hypersonic2 Cair = 298 m/s Ut = 1562 m/s y+= 0.3 (Rcell= 16) yH= 2e-6 m as ratio = 380 | ||
hypersonic3 Cair = 298 m/s Ut = 1608 m/s y+= 0.3 (Rcell= 16) yH= 2e-6 m as ratio = 380 | ||
hypersonic4 Cair = 298 m/s Ut = 1655 m/s y+= 0.3 (Rcell= 16) yH= 2e-6 m as ratio = 380 | ||
hypersonic5 Cair = 299 m/s Ut = 1702 m/s y+= 0.3 (Rcell= 16) yH= 2e-6 m as ratio = 380 | ||
hypersonic6 Cair = 299 m/s Ut = 1748 m/s y+= 0.3 (Rcell= 16) yH= 2e-6 m as ratio = 380 | ||
hypersonic7 Cair = 299 m/s Ut = 1795 m/s y+= 0.3 (Rcell= 16) yH= 2e-6 m as ratio = 380 | ||
hypersonic8 Cair = 300 m/s Ut = 1836 m/s y+= 0.3 (Rcell= 16) yH= 2e-6 m as ratio = 380 | ||
hypersonic9 Cair = 300 m/s Ut = 1878 m/s y+= 0.3 (Rcell= 16) yH= 2e-6 m as ratio = 380 | ||
hypersonic10 Cair = 301m/s Ut = 1920 m/s y+= 0.3 (Rcell= 16) yH= 2e-6 m as ratio = 380 | ||
hypersonic11 Cair = 302m/s Ut = 1961 m/s y+= 0.3 (Rcell= 16) yH= 2e-6 m as ratio = 380 | ||
Numerical method | Turbulence model | Spalart-allmaras: turbulent viscosity ratio 1 |
Materials | Density: ideal-gas; viscosity: sutherland law; Cp (j/kg-k): 1006.43 | |
Solver | Density-based solver adopting ROE flux type | |
Gradient: least-squares cell-based; Flow: second-order upwind | ||
Modified turbulent viscosity: second-order upwind |
Fig. 18The aerodynamic environment prediction under hypersonic condition characterized by NACA0012
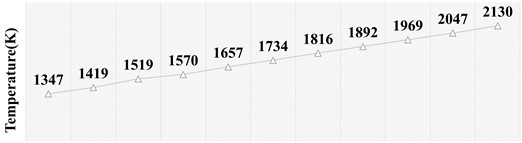
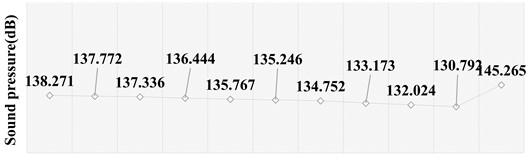
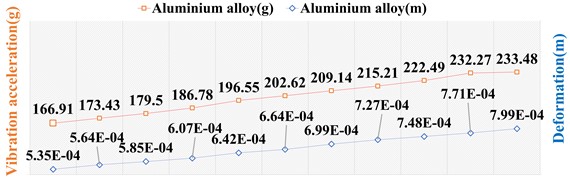
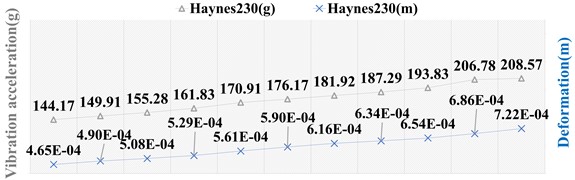
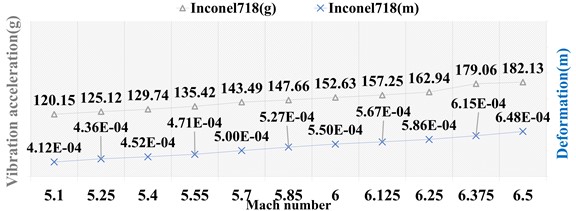
The aerodynamic environment prediction characterized by NACA0012 under hypersonic conditions is shown in Fig. 18. Firstly, the change of aerodynamic heat is analyzed. The maximum aerodynamic heat rises to 2130 K (1856.85 °C), which was consistent with the aerodynamic heat descriptions in Refs. [40], [41]. The temperature increases by 847 °C, with an average temperature change rate of about 77 °C/s. With the flow velocity increases, the aerodynamic thermal variation amplitude and average change rate are higher. The temperature extreme point is in front of the leading edge, and the temperature at the leading edge is higher than that at the trailing edge. Then, the aerodynamic sound pressure is analyzed. The minimum and maximum sound pressure levels are 130.8 dB and 145.3 dB. Fig. 19 demonstrates the distribution of frequencies in acoustic signals. Only in hypersonic 3 the middle and high frequencies above 100 Hz have contribution to the sound signal, and the middle and high frequencies in other substages are basically negligible. In general, it is the low-frequency signal within 100 Hz dominate under hypersonic conditions. Finally, the vibration analysis is conducted, and we first need to ascertain the material composition of the NACA0012 airfoil. The X43 A and X51 A are representative scramjet-propelled hypersonic vehicles, with the airfoil of the former utilizing haynes230 and that of the latter employing inconel718. In this paper, we adopt these two materials and the standard aluminium to analyze the influence of materials on vibration. Fig. 18 shows that Inconel718 has better compression and temperature resistance and could withstand relatively minimum average vibration acceleration and deformation amplitude. The NACA0012 with Inconel718 alloy experiences a maximum vibration acceleration ranges of 120 g to 182 g. Compared to aluminium alloy, the adoption of Inconel718 results in a vibration/deformation optimization range of 21.99 % to 28.02 % and 18.90 % to 22.99 %, respectively. As the flow velocity and temperature increase, both vibration acceleration and deformation also increase, leading to a decrease in the optimization degree achieved by Inconel718. Compared with aluminium alloy, Inconel718 still could bring a minimum optimization amplitude of 21.99 % for vibration acceleration and 18.90 % for deformation. The utilization of novel materials can yield considerable enhancements in structural reliability. To sum up, the scramjet-propelled vehicle encounters significant aerodynamic challenges under hypersonic conditions during the flight.
Fig. 19Distribution of acoustic signals of different frequencies under hypersonic conditions characterized by NACA0012
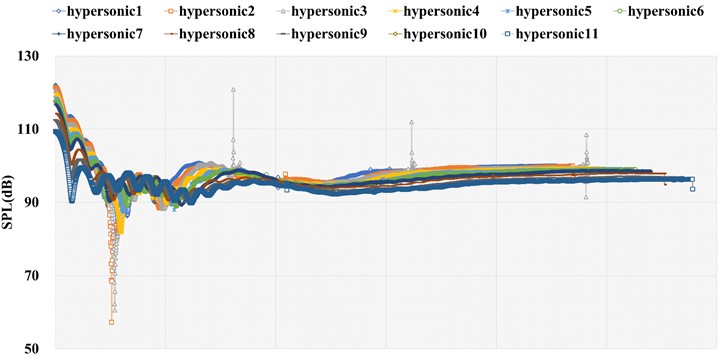
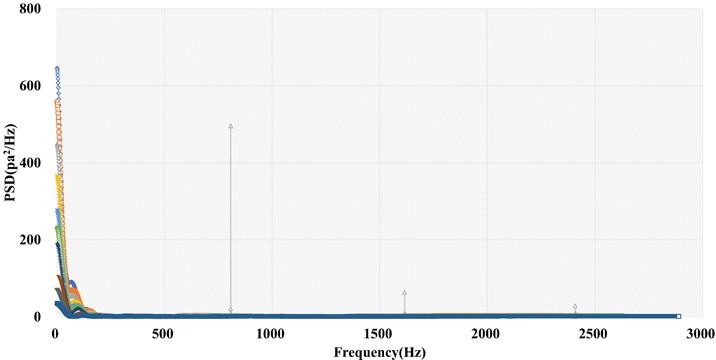
5. Conclusions
Aiming at the insufficient research on the aerodynamic environment prediction of scramjet-propelled vehicles characterized by NACA0012 under hypersonic conditions, in this paper, based on the wind tunnel experimental data, a comprehensive analysis is performed to study the influences of key simulation parameters on numerical accuracy and an effective simulation scheme for aerodynamic environment prediction under hypersonic conditions characterized by NACA0012 is proposed. In Section 2, we introduce the adopted grid strategy and numerical method. In Section 3, based on six NACA0012 models, three far field distances, two turbulence models, and two flux types, with additional three cell Reynolds numbers, and four aspect ratios, CFD simulations are conducted and the internal relationship between simulation parameters and numerical accuracy is discussed by comparing the numerical results with the wind tunnel data. Characterized by NACA0012, the optimal simulation configuration under hypersonic conditions is derived and the corresponding aerodynamic environment prediction is carried out in Section 4. Through systematic analysis, the study findings are as follows:
1) Compared with the blunt trailing edge, better numerical results could be obtained with the sharp trailing edge. It's worth noting that an incorrect sharp trailing edge modeling method could result in a higher numerical error ratio than a blunt trailing edge. Therefore, it's essential to select the sharp trailing edge modeling method with great care. Preference is given to the definition formula for designing the sharp trailing edge. The source and number of data points used by the definition formula would directly affect the numerical results. In this paper, the data points used in the definition formula are derived from Airfoil tools and NACA4, and the numerical analysis indicates that NACA4’s data points perform better. These two modeling data point sources have a negative correlation between the data points number and the numerical accuracy.
2) Unlike under incompressible conditions, the recommended values for far field distance and flux type are 16L and ROE flux type, respectively. In particular, the appropriate modified turbulent viscosity for Spalart-Allmaras turbulence model is second order upwind. Moreover, unlike taking the blunt cylinder as the characterized object, the proposed values of cell Reynolds number and aspect ratio of airfoil mesh cells close to the shock are no smaller than 16 and no larger than 380, respectively.
3) The extreme aerodynamic environments place high demands on ground environment testing. The temperature of heating device can reach 1900 ℃ and the maximum temperature rise rate can reach 77 ℃/s. The vibration table supports a maximum vibration acceleration of 182 g and the maximum sound pressure level of the sound test reverberation room reaches 145 dB, mainly containing low-frequency signals within 100 Hz. In addition, the influence of aerodynamic heat on the structural vibration increases with the increase of flow velocity, indicating thermal vibration testing should be conducted jointly during environmental testing to better evaluate the structural stability of the vehicle.
4) The utilization of advanced materials like Haynes230 and Inconel718 plays a pivotal role in enhancing structural reliability. Although the optimization efficacy of these materials diminishes with escalating flow velocity and aerodynamic heat, the growth remains considerable. Inconel718 having better compression and heat resistance performance, which is recommended for airfoil design.
5) The research conclusions proposed in this paper provide the basis for the parameter selection and simulation scheme design for predicting the extreme aerodynamic environment experienced by the hypersonic vehicle during the flight. Researchers could obtain more accurate environmental extremes, conduct more efficient structural design and ground testing, avoid redundant protection design, reduce costs, and improve efficiency.
6) The flight trajectory of hypersonic vehicle spans transonic, supersonic, high supersonic and hypersonic four stages. In this paper, we discuss the hypersonic conditions occupying over 90 % of the flight external flow field, while the other three external flow field are not investigated. To further improve the predictive accuracy of vehicle flight environment and provide more accurate numerical references for vehicle design and optimization, future research directions should be firstly to analyze the internal relationships between simulation parameters and numerical accuracy under the other three external flow fields in order, then perform a complete flight environment prediction based on the optimal simulation configurations concluded under four external flow fields.
References
-
B. C. Ai, Computational Aerodynamics of Near-Space Hypersonic Vehicles. Beijing: Science Press, 2020.
-
J. Lee and O. Rho, “Accuracy of AUSM+ scheme in hypersonic blunt body flow calculations,” in 8th AIAA International Space Planes and Hypersonic Systems and Technologies Conference, Apr. 1998, https://doi.org/10.2514/6.1998-1538
-
K. Kitamura, E. Shima, Y. Nakamura, and P. L. Roe, “Evaluation of Euler fluxes for hypersonic heating computations,” AIAA Journal, Vol. 48, No. 4, pp. 763–776, Apr. 2010, https://doi.org/10.2514/1.41605
-
I. Di Venuta, I. Petracci, M. Angelino, A. Boghi, and F. Gori, “Numerical simulation of mass transfer and fluid flow evolution of a rectangular free jet of air,” International Journal of Heat and Mass Transfer, Vol. 117, pp. 235–251, Feb. 2018, https://doi.org/10.1016/j.ijheatmasstransfer.2017.10.030
-
F. Qu, D. Sun, and C. Yan, “A new flux splitting scheme for the Euler equations II: E-AUSMPWAS for all speeds,” Communications in Nonlinear Science and Numerical Simulation, Vol. 57, pp. 58–79, Apr. 2018, https://doi.org/10.1016/j.cnsns.2017.09.002
-
F. Chen, H. Liu, and S. Zhang, “Time-adaptive loosely coupled analysis on fluid-thermal-structural behaviors of hypersonic wing structures under sustained aeroheating,” Aerospace Science and Technology, Vol. 78, pp. 620–636, Jul. 2018, https://doi.org/10.1016/j.ast.2018.05.015
-
F. Qu, J. Chen, D. Sun, J. Bai, and G. Zuo, “A grid strategy for predicting the space plane’s hypersonic aerodynamic heating loads,” Aerospace Science and Technology, Vol. 86, pp. 659–670, Mar. 2019, https://doi.org/10.1016/j.ast.2019.01.049
-
X. Zheng, Z. S. Liu, Y. Yang, J. C. L. Lei, and Z. X., “Research on climb-cruise global trajectory optimization for RBCC Hypersonic Vehicle,” Missiles Space Veh, Vol. 47, pp. 1–8, 2018, https://doi.org/10.7654/j.issn.1004-7182.20180201
-
“Speed of Sound Interactive.” Nasa, https://www1.grc.nasa.gov/beginners-guide-to-aeronautics/speed-of-sound-interactive
-
C. L. Ladson, “Effects of independent variation of Mach and Reynolds numbers on the low-speed aerodynamic characteristics of the NACA 0012 airfoil section,” National Aeronautics and Space Administration, Scientific and Technical Information Division, WDC, 1988.
-
E. Turkel, R. Swanson, V. Vatsa, and J. White, “Multigrid for hypersonic viscous two – and three-dimensional flows,” in 10th Computational Fluid Dynamics Conference, Jun. 1991, https://doi.org/10.2514/6.1991-1572
-
B. Epstein and A. Nachshon, “An ENO Navier-Stokes solver applied to 2-D subsonic, transonic and hypersonic aerodynamic flows,” in 32nd Aerospace Sciences Meeting and Exhibit, Jan. 1994, https://doi.org/10.2514/6.1994-303
-
J. P. Singh, “Evaluation of Jameson-Schmidt-Turkel dissipation scheme for hypersonic flow computations,” Journal of Aircraft, Vol. 33, No. 2, pp. 286–290, Mar. 1996, https://doi.org/10.2514/3.46935
-
I. H. Abbott and A. E. Von Doenhoff, Theory of Wing Sections: Including a Summary of Airfoil Data. New York: Dover publications, INC, 2012.
-
J. C. Vassberg and A. Jameson, “In pursuit of grid convergence for two-dimensional Euler solutions,” Journal of Aircraft, Vol. 47, No. 4, pp. 1152–1166, Jul. 2010, https://doi.org/10.2514/1.46737
-
B. Diskin, J. L. Thomas, C. L. Rumsey, and A. Schwöppe, “Grid-convergence of Reynolds-averaged Navier-stokes solutions for benchmark flows in two dimensions,” AIAA Journal, Vol. 54, No. 9, pp. 2563–2588, Sep. 2016, https://doi.org/10.2514/1.j054555
-
P. B. Devi, V. Paulson, V. Madhanraj, and D. A. Shah, “Heat transfer and temperature effects on a dimpled NACA0012 airfoil with various angles of attack,” International Journal of Ambient Energy, Vol. 39, No. 8, pp. 783–786, Nov. 2018, https://doi.org/10.1080/01430750.2017.1341432
-
P. Booma Devi and D. A. Shah, “Effect of square dimples on the temperature distribution and heat transfer coefficient of an NACA0012 airfoil,” International Journal of Ambient Energy, Vol. 40, No. 7, pp. 754–757, Oct. 2019, https://doi.org/10.1080/01430750.2017.1399450
-
D. R. Joseph, P. B. Devi, and M. Gopalsamy, “Investigation on effect of square dimples on NACA0012 airfoil with various Reynolds numbers,” International Journal of Ambient Energy, Vol. 42, No. 4, pp. 397–402, Mar. 2021, https://doi.org/10.1080/01430750.2018.1531267
-
N. Bekka, R. Bessaïh, M. Sellam, and A. Chpoun, “Numerical study of heat transfer around the small scale airfoil using various turbulence models,” Numerical Heat Transfer, Part A: Applications, Vol. 56, No. 12, pp. 946–969, Dec. 2009, https://doi.org/10.1080/10407780903508005
-
D. F. Hinz, H. Alighanbari, and C. Breitsamter, “Influence of heat transfer on the aerodynamic performance of a plunging and pitching NACA0012 airfoil at low Reynolds numbers,” Journal of Fluids and Structures, Vol. 37, pp. 88–99, Feb. 2013, https://doi.org/10.1016/j.jfluidstructs.2012.08.012
-
J. Cheng, Y. B. Lai, L. Wang, H. Wu, and L. Li, “Study on the icing characteristics of typical wind turbine,” (in Chinese), Journal of Hebei University of Science and Technology, Vol. 40, pp. 9–14, 2019, https://doi.org/10.7535/hbkd.2019yx01002
-
K. Fukudome, Y. Tomita, S. Uranai, H. Mamori, and M. Yamamoto, “Evaluation of anti-icing performance for an NACA0012 airfoil with an asymmetric heating surface,” Aerospace, Vol. 8, No. 10, p. 294, Oct. 2021, https://doi.org/10.3390/aerospace8100294
-
Q. L. Guo, J. J. Niu, B. An, W. M. Sang, and F. Zhou, “Numerical simulation of ice crystal icing under mixed-phase conditions,” (in Chinese), Acta Aerodynamic Sinica, Vol. 39, No. 2, pp. 168–175, 2021, https://doi.org/10.7638/kqdlxxb-2020.0182
-
K. Hokeun, “Numerical simulation of aeroacoustic noise at low mach number flows by using the finite difference Lattice Boltzmann method,” Journal of Advanced Marine Engineering and Technology, Vol. 28, No. 5, pp. 717–727, 2004.
-
W. Arias Ramírez and W. R. Wolf, “Effects of trailing edge bluntness on airfoil tonal noise at low Reynolds numbers,” Journal of the Brazilian Society of Mechanical Sciences and Engineering, Vol. 38, No. 8, pp. 2369–2380, Feb. 2015, https://doi.org/10.1007/s40430-015-0308-6
-
N. Rezazadeh, D. Perfetto, A. de Luca, and F. Caputo, “Ensemble learning for estimating remaining useful life: incorporating linear, KNN, and Gaussian process regression,” in Lecture Notes in Mechanical Engineering, pp. 201–212, Apr. 2024, https://doi.org/10.1007/978-3-031-52649-7_16
-
R. Perrin, P. Rattanasiri, E. Lamballais, and S. Yooyen, “Influence of the trailing edge shape on the aerodynamic characteristics of an airfoil at low Re number using RANS,” in IOP Conference Series: Materials Science and Engineering, Vol. 886, No. 1, p. 012021, Jul. 2020, https://doi.org/10.1088/1757-899x/886/1/012021
-
L. Yang and G. Zhang, “Analysis of influence of different parameters on numerical simulation of NACA0012 incompressible external flow field under high Reynolds numbers,” Applied Sciences, Vol. 12, No. 1, p. 416, Jan. 2022, https://doi.org/10.3390/app12010416
-
X. Zhang, C. Yan, W. Yang, and H. B. He, “Investigation of the grid-dependency in heat transfer simulation for hypersonic vehicle,” Tactical Missile Technolgy, Vol. 3, pp. 21–27, 2016, https://doi.org/10.16358/j.issn.1009-1300.2016.03.05
-
F. Qu, D. Sun, and G. Zuo, “A study of upwind schemes on the laminar hypersonic heating predictions for the reusable space vehicle,” Acta Astronautica, Vol. 147, pp. 412–420, Jun. 2018, https://doi.org/10.1016/j.actaastro.2018.03.046
-
“ICEM CFD Hexa 2D Airfoil meshing,” https://www.youtube.com/watch?v=tyrbscuh9re&t=107s (accessed Mar. 2023).
-
D. Perfetto, C. Pezzella, V. Fierro, N. Rezazadeh, A. Polverino, and G. Lamanna, “FE modelling techniques for the simulation of guided waves in plates with variable thickness,” Procedia Structural Integrity, Vol. 52, pp. 418–423, Jan. 2024, https://doi.org/10.1016/j.prostr.2023.12.042
-
H. Schlichting and K. Gersten, Boundary Layer Theory. Berlin: Elsevier BV, 2016.
-
“Ansys fluent theory guide,” Ansys, Canonsburg, PA 15317.
-
N. N. Smirnov, O. G. Penyazkov, K. L. Sevrouk, V. F. Nikitin, L. I. Stamov, and V. V. Tyurenkova, “Detonation onset following shock wave focusing,” Acta Astronautica, Vol. 135, pp. 114–130, Jun. 2017, https://doi.org/10.1016/j.actaastro.2016.09.014
-
F. R. Menter, “Two-equation eddy-viscosity turbulence models for engineering applications,” AIAA Journal, Vol. 32, No. 8, pp. 1598–1605, Aug. 1994, https://doi.org/10.2514/3.12149
-
P. Spalart and S. Allmaras, “A one-equation turbulence model for aerodynamic flows,” 30th Aerospace Sciences Meeting and Exhibit, p. 1994, Jan. 1992, https://doi.org/10.2514/6.1992-439
-
J. Z. Liu, C. Yan, L. Ke, and S. T. Zhang, “Research on scheme effect of computational fluid dynamics in aerothermal,” Journal of Beijing University of Aeronautics and Astronautics, Vol. 29, No. 11, pp. 1022–1025, 2003.
-
L. Shang, D. Wu, Y. Pu, H. Wang, F. Wang, and Z. Gao, “Experimental research on thermal insulation performance of lightweight ceramic material in oxidation environment up to 1700 °C,” Ceramics International, Vol. 42, No. 2, pp. 3351–3360, Feb. 2016, https://doi.org/10.1016/j.ceramint.2015.10.129
-
D. F. Wu, L. J. Lin, W. J. Wu, and C. C. Sun, “Experimental research on thermal/vibration test of lightweight insulation material for hypersonic vehicle under extreme-high-temperature environment up to 1500 °C,” Acta Aeronautica and Astronautica Sinica, Vol. 41, pp. 216–225, 2020.
About this article
This research is supported by Anhui Provincial Department of Education Natural Science Key Project (2023AH053014) and Anhui Public Security College Excellent Scientific Research and Innovation Team Project (2023GADT06).
The datasets generated during and/or analyzed during the current study are available from the corresponding author on reasonable request.
Fangli Ding: Conceptualization, methodology, investigation, resources, validation, visualization, writing-original draft preparation. Lu Yang: Conceptualization, methodology, investigation, formal analysis, resources, validation, visualization, writing-review and editing.
The authors declare that they have no conflict of interest.