Abstract
Robotics and automation have developed as key technologies for supply chain management as a result of the increased demand for quicker and more effective supply chains. Robotics and automation improve supply chain management by lowering long-haul expenses, boosting work and usage strength, reducing errors, declining repetitive stock checks, updating orchestrating, taking care of times, and assembling induction to the problematic and hazardous places. Robotics aids in design, creation, etc. Automation helps to do tasks that are often done by people through the use of self-operating physical machines, computer software, and other technology. Despite being widely accepted as a tool to aid in decision-making, supply chain management (SCM) has very seldom used AI and ML. This article investigates several AI and ML sub-fields that are best suited for resolving real-world SCM-related issues in order to fully realize the potential benefits of AI and Ml for SCM. In doing so, this article examines the track record of successful AI and ML applications to supply chain management and highlights the most fruitful SCM domains to apply AI and ML. And also find out the how robotics and automations helps in warehouse management. The most recent developments in robotics and automation for supply chain management are thoroughly reviewed in this paper. We first give a general overview of the difficulties that supply chain management faces before going over the many ways that robotics and automation are used at various points along the supply chain. Additionally, we go over the advantages of robots and automation in supply chain management, including higher efficiency, accuracy, lower costs, and improved safety. Lastly, we discuss some of the present drawbacks and difficulties associated with robots and automation in supply chain management and suggest some possible directions for further investigation.
1. Introduction
An essential part of the global economy, the supply chain sector is in charge of shipping, distributing, and delivering commodities to customers all over the world. Increasing demand, growing expenses, and the need for increased efficiency and speed are just a few of the issues the sector must overcome. By enhancing the precision, speed, and dependability of supply chain processes, robotics, and automation technologies have the potential to address many of these issues.. New and creative business models are developed as e-business expands. According to Da Silveira and Cagliano, modern supply chain specialists deal with "Omni channel retailing," "complex global supply chains," and "consignment inventory." As a result, e-commerce enterprises are investing heavily in the adoption of supply chain technology based on robotics and automation. The autonomous robot is capable of perception, decision-making, and action in response to changes in the surrounding environment. Mechatronics and automation is a versatile innovation that can be quickly used to computerize use cases across various industry/SCM segments because it is adaptable, painless, and versatile [1].
Obtaining, acquiring, changing, assembling, and operation-related movements of all kinds are planned out and made to happen in SCM. For this engagement, channel partners including providers, go-betweens, suppliers for outside operations, and clients must coordinate and work together. A strategy for dealing with the board that integrates market interest both inside and outside of organizations is to inventory network the executives. When SCM manages various tasks, it benefits the provider, retailer, and client. Organizations for invention, obtaining, item improvement, and calculated arrangements might use this dynamic cycle to effectively prepare workouts. The cycle can also be used to improve a variety of options. The most typical approach of thinking about all points of view when exploring options at various levels leads to beneficial arrangements.
SCM is a crucial component of modern corporate operations. According to research, the most significant and influential companies or sectors have claimed that SCM is quite probably the most competitive industry, underscoring the connection with other sectors, advertising, creation, and coordinated operations [2]. Purchasing raw materials from the manufacturer is the second phase in SCM after sourcing the raw materials, which is the first step. The manufacturing unit purchases the raw materials from the supplier and transforms them into the finished product in accordance with the desired product type. Then, based on demand, the wholesaler distributes these goods from the stock to the retailer, who then sells it to the client. Every participant in the supply chain network, from suppliers to retailers, must communicate with one another effectively. A strong supply chain network should be present in the flow that is shown in the Fig. 1 because it is essential for businesses and industries.
Fig. 1Supply chain management
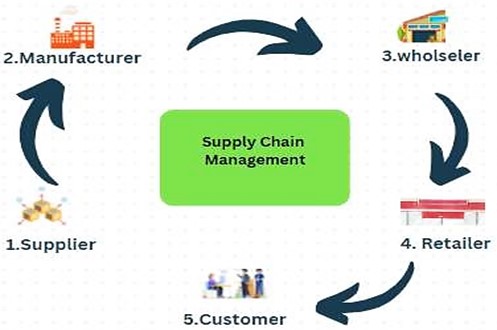
2. Evolution of supply chain management
This advancement is portrayed by the joining of discrete undertakings which were expanding from 1960, in the 1960s the framework was profoundly divided so it was a basic region for efficiency upgrades. Operations assignments even though have stayed comparative, they first solidified into two particular capabilities for Example materials on the board and actual circulation during the years the 1970s and 1980s. In the year1990s the interaction moved further as globalization induced a useful mix and rise of strategies in an obvious sense [3]. Every part of the creation network ended up being fundamental from a lone organization perspective. The total incorporation became conceivable with the assistance of data and correspondence advancements. This aids in the coordinated administration and data control, finance, and merchandise stream, and another scope of creation and dissemination framework was conceivable. Complex grouping of exercises building esteem catch and intensity made inventory network the board complex. The developing degree of mechanization as of late in-store network has been a prevailing component of the development of the two materials the executives and circulation frameworks. This digitalization is remarkable, especially inside the circulation habitats which have encountered a noteworthy street towards the away, material dealing with and bundling. Enhancements in data and Correspondence innovations have made it conceivable that two closures of the sequential construction system became incorporated into strategies and production networks. The improvements of high rack amassing ended up not being set in stone, or the inside advancement of packs by AGVs robots were early verbalizations of vital planning. Earlier tasks were a development isolated around the giving, warehousing, creation, and movement capacities, an enormous piece of them being truly free [3]. With the new affiliation and the board principles, firms followed a more planned approach, as such noting the impending interest in versatility without raising costs. As creation ended up being logically isolated, practices associated with its organization were combined. Spatial irregularity transformed into a symptom of economies of scale in movement. Fig. 2 tells about the how supply chain evolved from 1960-2010.
Fig. 2The Evolution of supply chain management
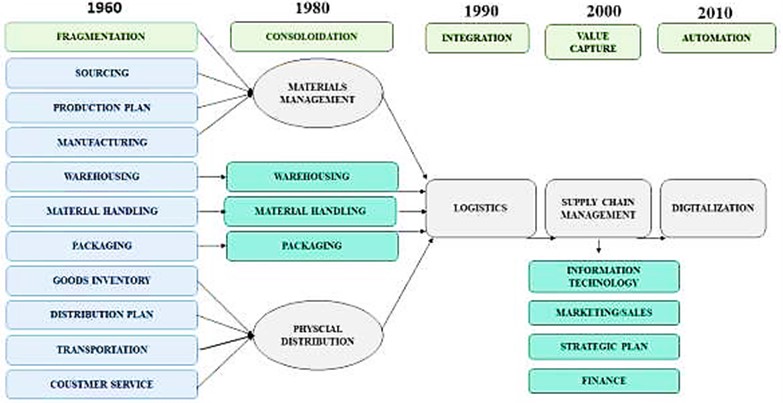
3. Use of robotics and automation in the supply chain in recent times
Fig. 3Recent use of robotics and automation in SCM from (2016-2020) [4]
![Recent use of robotics and automation in SCM from (2016-2020) [4]](https://static-01.extrica.com/articles/23349/23349-img3.jpg)
Robots and atomization exercises utilized in the acquirement and operations chain over the most recent four years have had an expanded speed as far as their utilization in the production network of the executives. 2017 had a development pace of the utilization of this innovation by 5.71 % contrasted with 2016. In 2018 the utilization of this innovation diminished by 8.11 % contrasted with 2017. In 2019 contrasted with the earlier year (2018) in which it diminished by 5.88 %. In 2020, contrasted with 2019 the ease of use pace of this innovation expanded by a level of 21.88 %. Looking at a long time from 2016 to 2020 the convenience pace of mechanization and mechanical technology innovation is expanding with a level of 11.43 % [4]. The graph below tells about the recent use of robotics and automation in supply chain management.
4. Robotics and automation in the supply chain
That advanced as we undoubtedly already know, robots and automation are used in each phase of the production network’s flow, which runs from supplier to client, to ensure that everything moves smoothly and quickly. In the SCM industry, automation mostly congregates to estimate, request arrange, and advance. These areas increase customer satisfaction and enhance assessments of cycles and resources. Since they may function in many SCM environments, expert-based structures can be thought of as the most likely areas of automation in SCM. However, for the most part, automotive use affects practical levels like checking, creation, and stockroom exercises. Automobile use can also have a negative impact on strategical, vital, and utilitarian powerful levels. Companies invest significant sums of money in research and development when using current computer-based intelligence technology and determining the best programmed solutions for commercial activities due to increasing intensity, request vulnerability, and stockpile risk. Additionally, it improves quality and the customer experience. Organizations can reduce waste and become more supportable by using exact gauges. They can also increase purchasing and lower expenditures. Automated robots equipped with cameras can recognize objects and materials and expedite picking. Information that is essential to all gatherings can be updated gradually, doing away with the need for challenging, mistakeprone compromise processes. It can also be updated further to handle other tasks to be accomplished and changed correctly to make it on time. . In the past, there was only a sequential computerized construction system. However, there are currently a few important viewpoints in the production network, a variety of emotionally supportive networks, and specialized equipment that are available for automating the procedures of marketing and dealing, as well as the relationships with the providers. The main goal of these numerous initiatives is to computerise every step of the production network, from the acquisition of raw materials to delivery to the final consumer [5]. This will enable us to serve our customers more effectively, efficiently, and quickly. As a result, automation initiatives that first started on the factory floor have now reached the whole network of stores.
The stockpiles was helped by developing innovations to anchor in each area and control all of the activities. These developments support mechanized binding supply.
4.1. Automation and robotics in the management of warehouses
By ensuring that goods are stored, picked, and delivered in a timely and effective manner, warehouse management plays a crucial function in the supply chain. However, warehouse management, which includes many responsibilities including inventory control, material handling, and order fulfilment, maybe a challenging and labour-intensive procedure. Numerous difficulties in warehouse management might affect the facility's overall effectiveness and efficiency [6]. Among the principal difficulties are:
– Picking and filling orders ineffectively and slowly.
– Poor inventory control and management.
– Demand planning and forecasts that are inaccurate.
– Improper and dangerous material and product handling.
– Inadequate quality assurance and testing.
Fig. 4Market size for warehouse automation worldwide from 2012 to 2026 [6]
![Market size for warehouse automation worldwide from 2012 to 2026 [6]](https://static-01.extrica.com/articles/23349/23349-img4.jpg)
4.2. Robotics and automation applications in warehouse management
By automating repetitive and time-consuming processes, increasing accuracy and safety, and enabling real-time visibility and control across the warehouse, robotics and automation have the ability to alleviate many of these issues. The following are some of the major uses of robots and automation in warehouse management:
– Automated transportation and material handling.
– Mobile autonomous robots for order fulfilment and picking.
– Automated inventory control and management.
– Automated inspection and quality control.
– Virtual and augmented reality for training and simulation.
– Automated Storage and Retrieval Systems (AS/RS).
4.2.1. Automated storage and retrieval systems (AS/RS)
Automated AS/RS systems are used to store and retrieve goods from warehouses. It is a collection of computer-controlled systems that help computerise stock management and store/recover goods upon request. The help in creating these frameworks makes it easier to work with quick item recovery and placement [7]. They operate as cranes, move along set rails, can surely traverse across item pathways and vertical levels, remove, and store objects. It is used to speed up order fulfillment and project management of supplies in a stockroom environment. The picture figure illustrates the AS/RS system.
Fig. 5Automated storage capacity and recovery frameworks (AS/RS) [8]
![Automated storage capacity and recovery frameworks (AS/RS) [8]](https://static-01.extrica.com/articles/23349/23349-img5.jpg)
4.2.2. Automated guided vehicles (AGVs)
AGVs are autonomous transportation systems that move goods inside of warehouses and manufacturing facilities. Typically, they are used to transport goods from one place to another, like from a storage area to a loading dock. AGVs replace physically demanding forklifts and pick trucks and help with material, supply, and stock movement. Some AGVs have independent paths that are marked out and separated from one another by wires, pretty strips, sensors, and tracks. In order to avoid impediments, another method makes use of a camera, LIDAR infrared technology, as well as other fundamental sensor technology, navigation technology, and system integration technology. AGVs in a warehouse are shown in the figure below.
Fig. 6Automated guided vehicles (AGVs) [9]
![Automated guided vehicles (AGVs) [9]](https://static-01.extrica.com/articles/23349/23349-img6.jpg)
4.2.3. Collaborative robots (Cobots)
These versatile, semi-independent robots were created to aid human employees in carrying out various tasks in a warehouse environment. A portion of the cobots patrol the stockroom floor in search of human pickers and serve as adaptable storage containers for orders that have been picked [9]. These cobots control work operations by driving partners, shifting loads, and using the distribution centre. A perfect path through the office is ensured by the sensors’ coordination, which allows them to recognize obstacles like boxes and snags. By organizing or picking at stations, cobots can deliver picked requests to workers in various locations across the stockroom, speeding up the process of satisfying customer demands. Cobots are seen in the figure below.
Fig. 7Collaborative robots (cobots) [10]
![Collaborative robots (cobots) [10]](https://static-01.extrica.com/articles/23349/23349-img7.jpg)
4.2.4. Control and supervision
Prior to the PC era, basic regulators were used to completing the continuing control of specific pieces of equipment, such as the machine, processing machine, and robot. At this time in the PC era, programmable regulators have taken the place of basic control frameworks. Plant automation networks have emerged with the emergence of the neighbourhood (LANs); we now have cell regulators managing various machines and production line regulators regulating a few of these cells. By using assembling regulators and stockroom-the-board systems, it is possible to process the natural material into a phone and have the finished product stored in an automated stockpiling and recovery system (ASRS). The development of administrative control frameworks and continual control frameworks has been aided by programmed control. With so many projects underway, mechanization and improving the productivity of working units inside the confines of a manufacturing facility have taken centre stage. After finishing their specific tasks, such as manufacturing robots, all robots that are integrated with one another work on information transfer. The robot that transported the material also works side by side with the subsequent robot to continue supplying the material for work. The illustration below demonstrates how several technologies are utilized for control and supervision. This plant has been automated for control and supervision, as seen in Fig. 8.
Fig. 8Control and supervision [11]
![Control and supervision [11]](https://static-01.extrica.com/articles/23349/23349-img8.jpg)
4.2.5. Goods-to-Person technology (G2P)
The modern high-volume operation-based e-commerce model, where online purchases are made up of specific products, is ideally suited for this technology. As a result, G2P is particularly helpful for fulfilling split-case orders, which require that individual units be picked up from separate locations and put into a shipping tote or carton. Traditional picking involved people going to a good model where each picker goes to a certain product to pick it. This caused labour-intensive time wastage that Seduced the quantity of orders completed. In the G2P approach, as opposed to the P2G model, the good is brought to the person rather than the other way around. The individually stored objects are mechanically taken out of storage and delivered to a picker or pick station. The emphasis at pick stations and packing stations is on ergonomics and high productivity because the picker does not have to walk. Model increases the number of orders completed per person and reduces the amount of time wasted between picks (Kim, 2015). There are many various types of G2P technology, but they are all founded on the same fundamentals: SKUs are brought by an automated system to a fixed pick station, not the other way around. There are many G2P systems that are incredibly effective currently on the market. Numerous component-based technologies, such as pallet based or tote-/carton-based systems, high-density storage systems, robots, horizontal and vertical carousels, and vertical lift modules, can be incorporated into these G2P solutions. Additionally, these systems are adaptable enough to be scaled for changes in increased or decreased product production.
5. Artificial intelligence (AI) and machine learning (ML) in supply chain
Artificial intelligence (AI) and machine learning (ML) have become effective technologies for enhancing supply chain management. The way businesses organize, carry out, and improve their supply chain activities has been revolutionized by the integration of various technologies. Accurate demand forecasting is one of the most important advantages of ML and AI in supply chain management. ML algorithms can reliably estimate demand by examining historical sales data and other factors, allowing businesses to optimize their inventory levels and decrease stock outs. As a result, clients are more satisfied as they receive their products fully and on time. By analysing factors like weather, traffic, and road conditions, ML and AI can also aid in the optimization of transportation routes [12].
5.1. Predicting the behaviour of customers
Consumers lack confidence and frequently behave emotionally. Nevertheless, the supply chain's performance is reliant on customer information and activity. Numerous spreadsheet-based techniques for predicting client behaviour were put forth, but as big data proliferated, they were shown to be outdated. Because they are not scalable for large-scale data, spreadsheet models for demand forecasting generally fail. They bring to light the supply chain management intricacies and uncertainties, which cannot be extracted, examined or handled using straightforward statistical techniques like moving averages or exponential smoothing [12]. This disorderly pattern might cause confusion within your team and a decline in production. Many organizations find it difficult to predict variable order volumes. But with AI and ML, we can predict client behaviour inconsistencies more accurately and early in such circumstances.
5.2. Forecasting demand is made easier by predictive skills
Demand forecasting is a branch of predictive analysis where businesses predict the demand for goods and shipments across the supply chain, even in the face of unforeseen circumstances. The multitude of parameters that affect demand in SC makes conventional methodologies, such as spreadsheet models, statistical models, moving averages, and exponential smoothing, which were previously addressed, limiting and overly simplistic, and erroneous. In this way, projections might only give a limited picture of how supply networks would experience variations in demand. Additionally, the non-linear nature of the unexplained demand variations could be attributed to their being nothing more than statistical noise. As a result, traditional or basic models are unable to represent crucial and non-linear aspects. Fortunately, machine learning offers algorithms that can map significant non-linear features and convert them into variables that can aid in understanding the past, accurately forecasting future events, helping them to enhance decision-making processes regarding cash flow, risk assessment, capacity planning, and workforce planning, as well as satisfy customer demands.AI Tools used in demand forecasting is shown in the Fig. 9.
Fig. 9AI Tools in demand forecasting
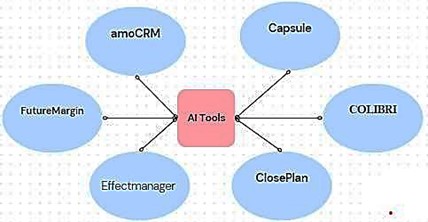
5.3. Predictive analytics for demand forecasting: retail chain forecast
The case concerns a furniture company’s Retail Chain (RC), whose estimates rely on based on consumer behaviour and the environment. The business foresaw the daily demand for several models on display in one of its physical stores. The models take into account many factors, such as the date and time of the purchase and the number of things purchased, to establish the sales trend. The company is now able to identify the seasonality and pattern of purchase behaviour in the data by utilising several ML models and AI tools. The company has seen that during the holiday season, sales are up. There is a correlation between the two, and the sales also rise or fall depending on the day’s weather and news event. The firm has now begun to understand the cause and effect link for foretelling future demand as a result of the climatic conditions (such as temperature, rainfall levels for a city, and data of mergers and acquisitions) and economic time-series data set that have been carefully selected.
5.4. Best routing option
In order to lower the costs associated with their current supply chain flows for 240 manufacturing facilities worldwide and 110 distribution centres, a company that deals in energy management, automation solutions, spanning hardware, software, and services, wanted to examine potential opportunities to assimilate new business units that they had recently acquired. The company developed a supply chain predictive model that could automatically generate the optimum routing alternatives for a massive raw materials supply chain, which comprises transformers the size of a big room and circuit breakers small enough to fit on a store shelf. They incorporated data from several business units into machine learning and artificial intelligence models to feed information about the enterprise supply chain, such as transportation tariffs and policies, information about product shipping routes, etc. Data engineers first created a technology for data extraction that could gather business data from all ERP systems, validate it, and then “clean” the data. More than 150 initial scenarios, 130,000 flow and routing constraints, and 200,000 transportation policy data points were examined by the customised model, which was able to identify $9.32 million (8 million Euros) in annual savings that could possibly be attained by changing product flow in the supply chain.
5.5. Convolution neural networks
CNNs are a particular kind of algorithm that is typically used for image recognition, but it turns out that they are also very helpful for forecasting. The best-known use of CNNs is to extract significant patterns and features from a dataset. Because of this, CNNs are particularly trustworthy when used to solve classification and regression issues [13]. One benefit of CNNs is that they share parameters, therefore they require fewer hyper parameters and less supervision than other classification algorithms. CNN is one of the most used algorithms in supply chain management because of this. Here are a few scenarios in which CNNs are employed.
5.6. Classification of images
The classification of a picture is accomplished by image recognition. It is quite useful in the supply chain since it can quickly classify various products and divide them properly. Product and component faults, abnormalities, and quality problems can be found using fAI image classification. Photos of the objects on the assembly line can be taken using cameras and sensors, and AI algorithms can classify these photos to find any deviations from the acceptable standards. This helps to minimise the likelihood that customers will receive subpar goods by ensuring that only high-quality products move forward in the supply chain. Technology that uses visual recognition can help with efficient inventory management. Artificial intelligence (AI) can automatically ascertain stock levels, identify low-stock conditions, and even forecast when things need to be reordered by analysing photographs of products on shelves, in warehouses, or on pallets. This improves inventory management and lowers stock outs.
5.6.1. Detecting objects
You can instantly recognize various items with the aid of object detection. In supply chain management, numerous items are encountered simultaneously. These items require a lot of effort and money to separate manually. Without the involvement of a person, object detection can help you swiftly identify and classify things, improving the accuracy of image classification. Several warehouse operations, including truck loading and unloading, item sorting, and shelf placement, can be automated using object detection. Robots with cameras and object detection software can quickly recognise and handle objects, eliminating the need for physical labour and speeding up operations. Real-time tracking of the movement of commodities is aided by object detection. To identify goods, count numbers, and keep tabs on changes in inventory levels, cameras and sensors can keep an eye on shelves and storage locations. This precise and automated method optimises inventory management by minimising errors and stock outs. Systems for detecting objects can help with picking and packing procedures. They direct workers to the appropriate products, ensuring that the appropriate goods are chosen for each order. Because of the increased order accuracy and quicker fulfilment, customers are more satisfied. The supply chain can use object detection by deploying cameras, sensors, and AI algorithms that can precisely identify and track items. To fully reap the rewards of object detection technology, integration with current systems and procedures is essential. Supply chain management has the potential to become even more effective, accurate, and agile as technology develops.
5.6.2. Segmentation of images
Another approach that uses CNN to generate a pixel-wise mask around the item itself and determine its dimensions is image segmentation. The below figure shows the example of image classification object detection and image segmentation.
Fig. 10Image classification, object detection, and image segmentation [14]
![Image classification, object detection, and image segmentation [14]](https://static-01.extrica.com/articles/23349/23349-img10.jpg)
Segmentation makes it possible to thoroughly evaluate products for flaws and anomalies. The ability to analyse each segment independently enables a more thorough evaluation of product quality. This helps find flaws and guarantee that only top-notch goods move forward in the supply chain. Monitoring product placement and stock levels is made easier by segmenting photos of store shelves. Retailers may determine which products are in short supply, which require restocking, and how various products are performing in terms of positioning and visibility by analysing shelf segments. Barcodes, QR codes, and other labels on goods or packages can be extracted and recognised with the use of segmentation. To accurately track and manage goods as they move through the supply chain, this is essential.
5.7. Representation learning
Another crucial machine learning technique is representation learning. Patterns and features are extracted using representation learning to:
– Develop generative models to produce realistic scenarios.
– Reduce dimensionality by comprehending the data.
A VAE auto encoder is utilized in representation learning, and a CNN architecture is used to compress the data so that latent variables or principal variables are present. With the help of these variables, we can comprehend how the data behaves and use it to build simulations that can stop businesses from suffering significant financial losses.
5.8. Nature language processing
NLP (natural language processing) addresses.
5.8.1. Sentiment analysis
Every business needs client feedback, which is typically found in the review sections of each product. It can be tiresome to manually go through each review and categorize it as good, poor, or anything in between. Based on user reviews and ratings, businesses can distinguish between good and bad products using sentiment analysis. This enhances the user experience. Gather pertinent text information from a variety of sources, such as social networking sites, consumer reviews, feedback forms, emails, and other supply-chain contact channels. To make the acquired text data ready for analysis, clean and pre-process it by removing extraneous details, special characters, and formatting. Give the text data sentiment labels, usually in the forms of positive, negative, or neutral [15]. More subtle categories like “very positive” or “slightly negative” may be used by some sophisticated systems. Early warnings about possible concerns can be obtained by monitoring public perception of supply chain disruptions or problems. Supply chain managers can take proactive steps to remedy the situation and lessen damage if negative sentiment is identified.
5.8.2. Chatbots
Using chatbots to enhance user experience is another option. NLP is what enables robots to comprehend customer issues or feedback when they are discussed with them by customers. Chatbots offer round-the-clock customer service, responding to common requests concerning purchase status, shipment details, refunds, and product inquiries. This lessens the workload for human workers and guarantees that clients get help when they need it. Chatbots enable customers to follow their orders in real-time [16]. The use of chatbots improves transparency and lowers consumer concern by retrieving and providing precise order status updates. Chatbots can assist customers in placing orders by assisting them in making product selections, adding goods to their shopping carts, and completing transactions. Chatbots can also help with order modifications, such as changing delivery addresses or removing an item. Chatbots can help supply chain participants like suppliers and manufacturers communicate more easily. They can track delivery timelines, automate order placing, and provide updates on material availability.
6. Machine learning algorithms
Due to their capacity to analyse vast volumes of data and optimize the supply chain process, machine learning (ML) algorithms have gained popularity in recent years [16]. A thorough analysis of the ML algorithms used in SCM and their applications is the goal of this study. Fig. 11 shows the different types of Machine Learning Algorithms.
SCM algorithms that leverage ML.
Fig. 11Machine learning algorithms
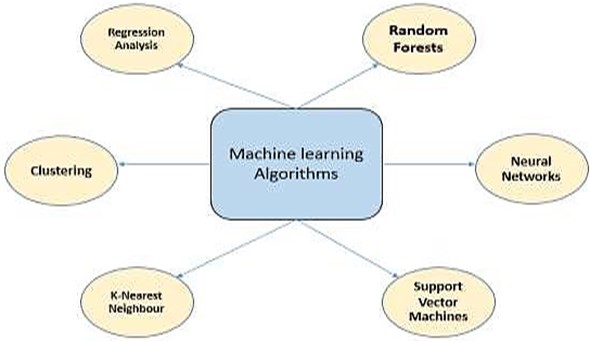
6.1. Regression analysis
Based on previous data, regression analysis is used to forecast future demand and sales volume. It aids in the discovery of trends and patterns as well as the forecasting of sales quantities, allowing for more precise demand planning. The relationship between demand and different factors, such as historical sales data, economic indicators, marketing efforts, and seasonality, can be modelled using regression analysis. Supply chain managers can enhance inventory management and decrease stock outs by analysing these factors to get more precise demand estimates. By taking factors like lead time, order frequency, demand variability, and order costs into account, regression analysis can assist in determining the ideal quantity of inventory. Supply chain managers can reduce holding costs while assuring product availability by understanding the links between these parameters [17]. The relationship between lead times and several variables, such as supplier location, order quantities, and transit modalities, can be modelled using regression analysis. This lowers planning uncertainty by enabling supply chain managers to forecast lead times more precisely. Regression analysis in the supply chain can be used successfully by gathering accurate and pertinent data for the relevant variables. Making educated decisions also requires selecting the right regression model (linear, multiple, logistic, etc.) and accurately interpreting the findings. Regression analysis is a powerful tool that supply chain managers may use to obtain insights, optimise different elements of their operations, and make datadriven decisions.
6.2. Random forests
A decision tree technique used in SCM for classification and regression issues is known as a random forest. It can be applied to forecast product demand, evaluate supplier performance, and spot fraudulent behaviour. By examining previous sales data combined with pertinent elements including sales, seasonality, economic indicators, and industry trends, Random Forests can be utilised to estimate demand [18]. Consequently, inventory planning and replenishment estimates made by supply chain managers are more accurate. Random Forests can help with inventory level optimisation by taking many factors into account, including as lead times, demand unpredictability, supplier performance, and carrying costs. As a result, inventory expenses and stock out risks are more evenly distributed. To assess the performance of suppliers, Random Forests can examine a variety of supplier-related criteria. Lead times, quality indicators, dependability of delivery, and cost are all included. The algorithm can offer perceptions into the choice of suppliers, bargaining, and relationship management. Data on product quality, production procedures, and defect incidence can be analysed using Random Forests to find patterns and connections.
6.3. Neural networks
A deep learning algorithm that can be used to forecast demand and optimize inventory levels is the neural network. They can be applied to data clustering, pattern recognition, and outcome prediction. In the supply chain, neural networks, a class of machine learning algorithms inspired by the organisation of the human brain, can be applied in a variety of ways to resolve difficult issues and enhance decision-making. To produce precise demand forecasts, neural networks can capture nonlinear correlations between past sales data, economic indicators, and other pertinent aspects. When dealing with complex demand patterns and relationships, this is especially helpful [19]. By examining traffic data, delivery times, and transportation costs, neural networks can optimise transportation modes, schedules, and routes. They assist in lowering transportation costs and enhancing delivery effectiveness. To quickly identify anomalies and flaws, neural networks may analyse data from sensors and quality control procedures. This helps ensure product quality and cut down on waste.
6.4. Support vector machines (SVMs)
Are an approach for supervised learning that may be applied to both classification and regression analysis. SVMs are employed in SCM to categorize goods according to demand, find suppliers, and improve logistics. By categorising items as defective or not defective based on attributes retrieved from sensor data or visual inspections, SVMs can assist in quality control. SVMs can identify fraudulent activity in supply chain finance by categorising transactions as genuine or suspect based on trends and anomalies. Based on previous order data, supplier performance, and outside variables, SVMs may forecast whether a given order will be filled on time. Based on their purchasing patterns, tastes, and demographics, SVMs can divide their clientele into many categories. This supports specialised supply chain strategies and targeted marketing campaigns.
6.5. K-Nearest neighbor
Non-parametric approach that can be used for regression and classification analysis. It is employed in SCM to assess supplier performance and forecast product demand. Based on elements like business model, market segment, and collaboration aims, KNN can find suppliers that are similar to one another. This promotes teamwork and information exchange. In order to find the k-nearest neighbours of a given data point, KNN measures the separations between data points in a feature space. In classification tasks, the class of the data point is determined by the majority class among these neighbours. KNN is adaptable for a variety of supply chain applications since it can handle both numerical and categorical data [20]. Preparing the data, picking the right distance measure, deciding on the value of k, and assessing the model's performance are all steps in the implementation of KNN in the supply chain. KNN is a practical tool for situations where data linkages are not very complex because of its clarity and interpretability.
6.6. Clustering
Using the unsupervised learning process of clustering, comparable data points can be grouped together. When employed in SCM, it can be utilised to determine consumer segmentation, optimise inventory levels, and pinpoint the most lucrative products [21]. Markets can be divided via clustering according to factors including competitiveness, consumer preferences, and purchasing power. Making informed choices about market entry and growth is aided by this. Using clustering, it is possible to group returned goods according to their cause, state, and intended use (repair, refurbishing, and disposal). Clustering is implemented by choosing the right algorithm (such K-Means, Hierarchical Clustering, or DBSCAN), figuring out how many clusters there will be, choosing the right characteristics, and assessing the outcomes. By adapting strategies to individual segments, clustering enables supply chain managers to make data-driven decisions, optimise operations, and improve customer satisfaction [22].
7. Examples of how AI and ML have been used in supply chain management
7.1. Amazon intelligent revenue and supply chain (IRAS) management: the intelligent revenue and
Supply Chain (IRAS) platform from Accenture integrates knowledge and results obtained from ML and AI models into its business and technological ecosystem. Its goal is to make the supply chain management system as a whole better. In order to ensure that the entire system is efficient and effective, IRAS also takes care of optimizing the forecasts and numerous other models [23].
7.2. On-road integrated optimisation and navigation system, or UPS ORIAN
UPS is a global provider of package transportation and supply chain management services. The average UPS driver makes roughly 100 deliveries every working day, despite the company boasting that it delivers thousands upon thousands of packages daily. UPS provides the best on-road integrated optimization and navigation (ORIAN) technology to ensure that items are delivered easily and on schedule. It makes sure that UPS drivers use the shortest, most efficient delivery routes in terms of time, distance, and fuel. The business claims that ORION uses extremely sophisticated algorithms to collect and analyze massive amounts of data in order to optimize routes for the drivers. This makes UPS’s delivery and pickup processes far more effective. The technology uses information from web maps to compute distance and travel time and create the shortest, most economical routes [24].
7.3. With AI, Rolls Royce reimagines freight transportation safety protocols
The venerable British automaker Rolls Royce collaborated with Intel to develop a clever AI system that may speed up and improve commercial shipping. They assert that this technology will be able to independently control communications, navigation, and obstacle detection, creating a new fleet of autonomous ships. This partnership can assist us in assisting ship owners with the automation of their navigation and operations, minimizing the possibility of human mistakes and allowing personnel to concentrate on more beneficial activities [25].
Fig. 12Use of AI globally in manufacturing and supply chains [26]
![Use of AI globally in manufacturing and supply chains [26]](https://static-01.extrica.com/articles/23349/23349-img12.jpg)
The supply chain AI adoption by worldwide businesses and industries is shown in the above graph. Grey indicates an infinite adoption of AI in businesses, orange indicates a plotting use case for AI, yellow indicates a widespread adoption of AI and ML, and blue with 11 % indicates that businesses view AI and ML are essential.
8. Result and discussion
The supply chain's efficiency, productivity, and cost savings have significantly increased with the usage of robotics and automation, AI, and ML. Worldwide e-commerce and online sales are increasing, and with that growth comes a need for products to flow through the supply chain more quickly. Customers’ rising expectations for quicker order fulfilment, fierce rivalry, and the emergence of newer business models have driven businesses, particularly ecommerce firms, to seek out cutting-edge technological solutions in order to serve their clientele. This causes a new generation of technological solutions to emerge, including mobile, intelligent, autonomous, and smart robots that can be valuable in supply chains and warehouses in particular. These solutions are enabling these businesses to quickly grow and meet the rising demand while ensuring lower operating costs, maintaining their competitiveness, and managing the labour shortages and efficiency further. Businesses can improve throughput, lower errors, and cut labour costs by automating repetitive and manual processes. Furthermore, the application of AI and ML has allowed businesses to forecast demand trends, optimize inventory levels, and identify potential supply chain disruptions in real-time.
Concerns over the displacement of human labour and potential job losses have also been highlighted by the use of robotics, automation, AI, and ML in the supply chain. However, proponents contend that these technologies can actually generate new employment prospects in fields like robot programming and maintenance. By aiding with physically taxing or dangerous jobs, collaborative robots, also known as cobots, can increase worker safety and morale generally. The up-front expenditures related to installing these technologies are another issue. However, many businesses find the investment to be justified due to the possible long-term cost savings and the boosted effectiveness and productivity. As the world becomes more interconnected and as the times change, there is more fierce competition and rivalry among organisations. Businesses are competing for growth and revenue generation while technology advances at an exponential rate. As we can observe, robotics are now being used in a variety of industries to complete challenging jobs. Automation is currently being used by businesses in every industry to combine human and machine labour.
However, AI will continue to advance and become more sophisticated than it already is. This development will intensify human and AI collaboration and possibly lead to ground-breaking innovations not only in the supply chain but also in other crucial industries. Furthermore, extensive data processing and analysis are needed in order to integrate AI and ML in supply chain management. Companies must therefore make sure they have the tools and knowledge needed to manage data properly. To ensure the successful application of these technologies, it could be necessary to spend more money on training or hiring data scientists. The application of robots, automation, AI, and ML in the supply chain is, all things considered, a positive development with great room for growth in the future. The supply chain industry is anticipated to improve in efficiency, cost-effectiveness, and safety over the next few years as businesses continue to invest in these technologies.
9. Conclusions
The supply chain has seen a considerable increase in efficiency, productivity, and cost savings through the use of robotics and automation, AI, and ML. The need for logistics robots is skyrocketing. According to a recent analysis by Tractica Research, warehousing and logistics robot sales will reach $22.4 billion globally by the end of 2021, with annual robot unit shipments expected to reach 620,000 units. More than 50 established and up-and-coming businesses are vying for clients in this market. The global warehouse robotics industry is anticipated to produce $10.34 billion in sales.
This demonstrates that robotics-based modern logistics will shape supply chains around the world in the coming years. The organisations may handle product movement considerably more effectively and efficiently while removing the chance of human error by automating the fundamental tasks of product movements in an assembly line, a manufacturing facility, or a warehouse. Mobile robotics solutions lessen the likelihood that a product would be erroneously received, stored, transferred, picked, packed, or shipped to the client. These robots reduce stress and make life and business operations safe.
At the end of the day, adding automation to the warehouses doesn't necessarily require a one-time upfront investment. It may be possible to upgrade the current warehouse system gradually with automation (Star ship, 2018) [27]. E-retailers can have a complete warehouse system that is fit for their business and can handle even complicated picking issues with the well-planned integration of the necessary equipment. Throughput has increased, mistakes have decreased, and labour expenses have been kept to a minimum as a result of automating repetitive and manual processes. Companies can now predict demand trends, optimize inventory levels, and spot possible supply chain problems in real-time thanks to the application of AI and ML.
Proponents contend that these technologies can generate new job opportunities and that long-term cost savings and improved efficiency make the investment worthwhile, despite concerns regarding the displacement of human labor and the upfront costs related to implementing them. The supply chain business is anticipated to get more productive, economical, and secure as technology advances. The supply chain's use of robotics, automation, AI, and ML has a bright future, with room for more technological improvements. In order to enhance supply chain operations and maintain market competitiveness, businesses need therefore continue to invest in these technologies.
Although there are many advantages to using robotics and automation, AI, and ML in the supply chain sector, there are also difficulties in putting them into practice. The expense of implementing these technologies, which can be substantial, is one of the biggest obstacles. The requirement for data infrastructure and knowledge to manage the huge amounts of data created by these technologies presents another difficulty. Concerns regarding job loss and the ethical ramifications of AI and ML have also been expressed.
10. Future technologies that can use in supply chain management
Supply chain management can benefit from the usage of block chain technology to improve transparency and traceability. Businesses may track the movement of items and make sure they are being moved and stored in line with industry standards and best practices by using a distributed ledger. Block chain can be used to monitor product authenticity and stop fraud.
The location and condition of commodities can be tracked throughout the supply chain using IoT devices like sensors and RFID tags. Companies may enhance inventory management, optimize logistics processes, and make sure that goods are being transported and stored in the proper conditions by gathering and analysing data from these devices. Workers in the warehouse or on the production floor can receive real-time information thanks to augmented reality technology. Workers can obtain instructions and suggestions on how to complete tasks by wearing AR headsets, decreasing errors and increasing efficiency.
Overall, a wide range of technologies is available for use in the supply chain sector, depending on the particular requirements and objectives of each company. Companies may enhance their supply chain operations, lower costs, and gain a competitive edge in the market by combining the proper technologies. In the field of SCM, AI's future looks bright. We recommend the following line of AI research topic areas that can improve SCM decision-making processes based on anticipated advances in AI research.
A new set of strategic SC issues, including SC integration and SC risk (disaster) management, can be addressed by multiple agent-based systems that are better able to manage complexity.
Reverse auctioning with SC partners and real-time pricing can both be done using intelligent agents.
Agent-based systems can include game theory to comprehend SC dynamics and create tactical SC alliances.
Using knowledge discovery approaches, profiles of ideal SC partners can be created, including suppliers and 3PL providers.
Rule-based expert systems can be created to aid with contract manufacturing or logistics outsourcing decisions.
It is possible to create expert systems that enhance airline revenue management.
Hybrid meta-heuristics can be created to handle combinatorial transportation network design challenges by combining the AI capabilities of GAs with those of ant colony optimisation.
The GA or ANN methodologies can be combined with the fuzzy logic approach to reduce overall logistical expenses.
The SC knowledge management framework can incorporate a rule-based expert system.
To handle the evaluation and selection concerns of overseas suppliers or 3PLs, rough set theory and/or machine learning can be further investigated.
Without impairing information flows between SC partners, AI may be integrated with the legacy systems already in place at multiple SC partners.
References
-
Fitzgerald J. and Quasney E., “Using autonomous robots to drive supply chain innovation,” Deloitte Perspectives, Vol. 1, No. 12, 2017.
-
Y. Yu, X. Wang, R. Y. Zhong, and G. Q. Huang, “E-commerce logistics in supply chain management: practice perspective,” Procedia CIRP, Vol. 52, pp. 179–185, Jan. 2016, https://doi.org/10.1016/j.procir.2016.08.002
-
J.-P. Rodrigue, The Geography of Transport Systems. Abingdon: Routledge, 2020.: Routledge, 2020, https://doi.org/10.4324/9780429346323
-
E. Puica, “How is it a benefit using robotic process automation in supply chain management?,” Journal of Supply Chain and Customer Relationship Management, pp. 1–11, Jan. 2022, https://doi.org/10.5171/2022.221327
-
N. Viswanadham, “The past, present, and future of supply-chain automation,” IEEE Robotics and Automation Magazine, Vol. 9, No. 2, pp. 48–56, Jun. 2002, https://doi.org/10.1109/mra.2002.1019490
-
“What is warehouse robotics?.” https://www.netsuite.com/portal/resource/articles/ecommerce/warehouse-robotics.shtml.
-
“Emerging warehouse automation and it’s benefits,” https://navata.com/cms/benefits-of-warehouse-automation/
-
https://www.masterautomation.in/warehouse-automation-asrs/
-
“AGV robots: unlocking your warehouse’s full potential,” https://www.howtorobot.com/expert-insight/agv-robots
-
“https://www.tyasuite.com/inventorymanagemen,” https://www.tyasuite.com/inventorymanagemen
-
“Robot market takes a hit from COVID-19,” https://www.designnews.com/automation/robot-market-takes-hit-covid-19
-
https://neptune.ai/blog/use-cases-algorithms-tools-and-example-implementations-of-machine-learning-insupply-
-
Pandian and Dr. A. Pasumpon, “Artificial intelligence application in smart warehousing environment for automated logistics,” December 2019, Vol. 2019, No. 2, pp. 63–72, Dec. 2019, https://doi.org/10.36548/jaicn.2019.2.002
-
https://www.pinterest.com/pin/634515034979360508/
-
Yuvaraja Devarajan, “A study of robotic process automation use cases today for tomorrow’s business,” International Journal of Computer Techniques, Vol. 5, No. 6, pp. 12–18, 2018.
-
H. Bui Trong and U. Bui Thi Kim, “Application of information and technology in supply chain management: case study of artificial intelligence – a mini review,” European Journal of Engineering Research and Science, Vol. 5, No. 12, pp. 19–23, Dec. 2020, https://doi.org/10.24018/ejers.2020.5.12.2254
-
https://www.vecteezy.com/vector-art/2094271-business-partnership-conceptual-design-businessman – handshake-together-on-top-mobile-phone-vector-isometric-illustration
-
C. Flechsig, F. Anslinger, and R. Lasch, “robotic process automation in purchasing and supply management: a multiple case study on potentials, barriers, and implementation,” Journal of Purchasing and Supply Management, Vol. 28, No. 1, p. 100718, Jan. 2022, https://doi.org/10.1016/j.pursup.2021.100718
-
K. L. Lee, A. Nurul Ain Najiha, J. R. Hanaysha, H. M. Alzoubi, and M. T. Alshurideh, “The effect of digital supply chain on organizational performance: An empirical study in Malaysia manufacturing industry,” Uncertain Supply Chain Management, Vol. 10, No. 2, pp. 495–5, 2022.
-
J. Thelander and V. Pettersson, “Implementation of procurement 4.0 technologies: a systematic content analysis on implementation factors,” Linnaeus University, Jun. 2021.
-
Rupa Dash, Mark Mcmurtrey, Carl Rebman, and Upendra K. Kar, “Application of artificial intelligence in automation of supply chain management,” Journal of Strategic Innovation and Sustainability, Vol. 14, No. 3, pp. 43–53, Jul. 2019, https://doi.org/10.33423/jsis.v14i3.2105
-
“Control software SWM LIne Supervisor,” https://www.directindustry.com/prod/smi/product-15003-1954659.html
-
S. Misra, C. Roy, and A. Mukherjee, Introduction to Industrial Internet of Things and Industry 4.0. CRC Press, 2021, https://doi.org/10.1201/9781003020905
-
R. Cole, M. Stevenson, and J. Aitken, “Blockchain technology: implications for operations and supply chain management,” Supply Chain Management: An International Journal, Vol. 24, No. 4, pp. 469–483, Jun. 2019, https://doi.org/10.1108/scm-09-2018-0309
-
M. Finger, I. Lapenkova, D. Kupfer, and M. Klumpp, “Regulation for artificial intelligence and robotics in transportation, logistics and supply chain management,” Network Industries Quarterly, Vol. 20, No. 2, pp. 3–7, 2018.
-
A. Chauhan, B. Brouwer, and E. Westra, “Robotics for a quality-driven post-harvest supply chain,” Current Robotics Reports, Vol. 3, No. 2, pp. 39–48, Apr. 2022, https://doi.org/10.1007/s43154-022-00075-8
About this article
The authors have not disclosed any funding.
The datasets generated during and/or analyzed during the current study are available from the corresponding author on reasonable request.
Om Banur – data research, writing of paper. Bhumeshwar Patle – formatting and editing. Sachin Pawar – formatting and editing.
The authors declare that they have no conflict of interest.