Abstract
In this study, unlike traditional design, innovative and exploratory design results that meet multiple criteria and reveal more than one option in the design of mechanical parts will be revealed. Artificial intelligence applications are now used in today’s technology in solving engineering problems in the design of mechanical parts and in design solutions that the human mind will never think of on its own. Thus, designers and engineers enter the design parameters (such as material, size, weight, power, manufacturing methods, and cost constraints) into the design software, and the software quickly creates hundreds or even thousands of design options to investigate and reveal all possible combinations of solutions. Thus, designers or engineers can choose the appropriate model by comparing CAD results that best meet their needs. In this article, unlike traditional design on an exemplary application, artificial intelligence-supported design outputs were explored. The results obtained, unlike traditional design, reveal many lighter and optimized design results compared to different materials and manufacturing processes.
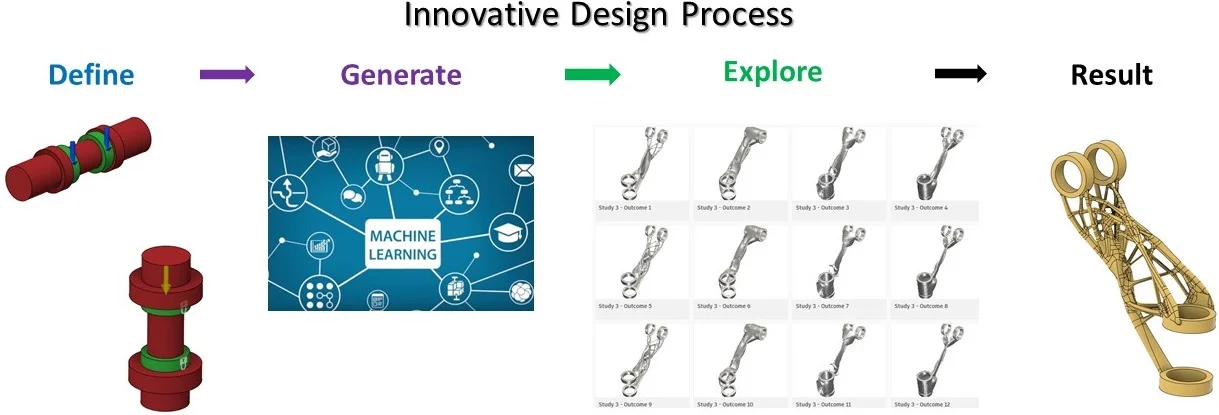
1. Introduction
Mechanical system design is an activity where different scientific, industrial and social activities are combined in a controlled hierarchy. Within this hierarchy, there is a process from mechanical part thought to production. The main starting point in the development of a particular design is to create a structure consisting of a regular combination of few or few physical parts that will perform a desired function or function under predetermined conditions. Today, the design of mechanical parts is provided by computer aided programs (CAD). In addition, when designing a part, parameters such as the type of material, mechanical strength and production patterns affect. For this reason, as shown in Fig. 1 in the design of traditional mechanical parts, the design put forward by the designer can change according to the desired material type, analysis results, production methods [1, 2]. For this reason, the design and production processes of mechanical parts can be challenging. Also, mechanical parts designed in CAD programs are limited depending on the ability of the designer and the program used.
Fig. 1The traditional approach process in the design of mechanical parts [3]
![The traditional approach process in the design of mechanical parts [3]](https://static-01.extrica.com/articles/21473/21473-img1.jpg)
![The traditional approach process in the design of mechanical parts [3]](https://static-01.extrica.com/articles/21473/21473-img2.jpg)
As you know, artificial intelligence (AI), one of today’s popular topics, is a field of computer science that emphasizes the creation of smart machines that think and react like people. Machine learning basically stems from artificial intelligence. In other words, it can be said that machine learning is the sub-branch of artificial intelligence. Machine learning includes an adaptive mechanism that allows you to learn computer experience, learn with examples, and these learning capabilities improve the intelligence system over time. Recently, intensive studies have been carried out in terms of technological developments in artificial intelligence and design of mechanical parts. In these studies, the innovative approach offered to the user with the support of artificial intelligence according to the desired criteria is called Generative design [4-6]. As a result of the necessary inputs to the designer computer, the modeling of the mechanical part under the desired conditions can be achieved with this technology. With this method, this idea is presented to the designer by presenting multiple design examples in a short time under the desired conditions. In this way, artificial intelligence applications can demonstrate many design examples according to the desired mechanical strength and production styles by trying different materials depending on the mechanical limitations and load conditions required for the design [7, 8].
Fig. 2The innovative approach process in the design of mechanical parts [3]
![The innovative approach process in the design of mechanical parts [3]](https://static-01.extrica.com/articles/21473/21473-img3.jpg)
![The innovative approach process in the design of mechanical parts [3]](https://static-01.extrica.com/articles/21473/21473-img4.jpg)
In the design of today’s industrial products, the products that come out as a result of artificial intelligence supported design may differ from classical designers. The resulting products are taught to the program through machine learning, the inputs and outputs previously used in the design process. In this way, the software creates the design process on different materials by calculating the production method by using topology optimization under the boundary conditions requested by the designer [9-11]. The design products that come out are different from the classical design results, but with the desired strength, light weight and many more designs, this idea is presented to the designer [12].
In this study, a sample piece and a machine learning supported design program will reveal the designs to solve the desired problem in the specified boundary conditions, and the examination and results of these design products will be revealed.
2. Material and methods
In our artificial intelligence-supported design, our product is the handlebar neck, which is a part of the bike that everyone uses a lot. This part is an intermediate part used to guide the bicycles by the user. This part is a spacer that transmits the movement on the steering wheel to the shaft attached to the front wheel at the request of the bicycle rider. As a result of the forces applied under the desired boundary conditions of this part, design examples with artificial intelligence supported, which provide the desired mechanical strength and production type with different materials will be introduced. It was realized with the Fusion 360 CAD program of Autodesk, which works as an artificial intelligence supported program. With this program, basic CAD data was entered and the desired part was designed with artificial intelligence support.
Fig. 3Handlebar neck in the parts of the bike
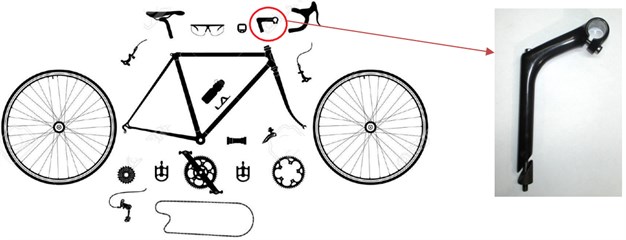
2.1. Modeling
The steering and front wheel main shaft part, to which the bicycle part of the handlebar neck will be attached, was drawn as a representation in the Fusion 360 program (Fig. 4(a)). Regions that should or should not be in the design that will emerge on this design were introduced. Thus, as shown in Fig. 4(b), the regions that should be in the design were modeled in green, and the regions that were not intended to be in the design were modeled as shown in red. Thus, while creating the program model, its limits are determined.
Fig. 4Modeling the design criteria of the bicycle part: a) region where the model will be created, b) desired and unwanted parts in the design
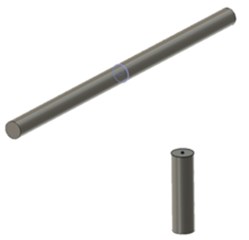
a)
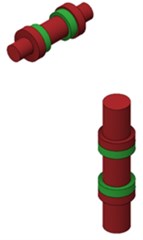
b)
2.2. Design conditions
In order to ensure that the model to be created within the design criteria is robust in terms of mechanical strength, mechanical limitations and forces have been defined on the model. The mechanical strength of the model, which will be created as a result of these restrictions, has been provided. As shown in Fig. 5, the regions fixed on the region that should be in the model and applied force and their values are shown. In this way, it is aimed to create the model according to the loads that the model should bear.
2.3. Design criteria
Some criteria can be proposed in the design of the model under the specified load and boundary conditions. The first one is to compare the model to be created with the specified materials according to minimum weight or maximum strength, while the other is the production method. In this study, the minimum weight of the model and the safety factor in terms of strength were asked to be at least 2. In addition, the model to be created was requested to be produced according to the parameters given in Fig. 6, to be produced in a 3D printer. In the creation of these models, he was asked to conduct research on cobalt chrome, titanium, aluminum and inconel materials.
Fig. 5Defining the boundary conditions and loads in the design of the bicycle part
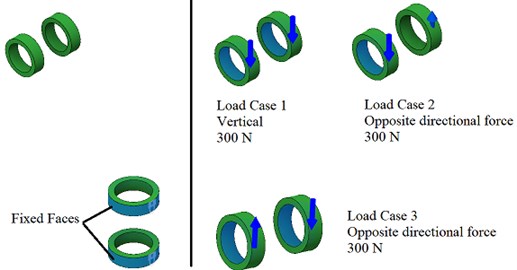
Fig. 6Determining the design criteria of the model
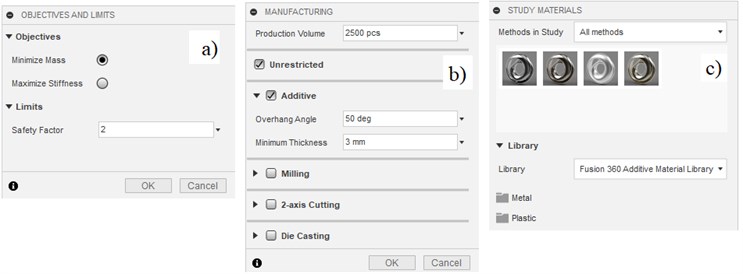
3. Result and discussion
Unlike traditional design, 16 different results were found in the design process with Generative design, which is artificial intelligence supported design. These results were found entirely by the program depending on the description of the geometry, loading and boundary conditions, different material types and production variety. These results mimic nature in the modeling of the classical designer. These results provide the designer with multiple models that he can explore.
Fig. 7Artificial Intelligence supported design models
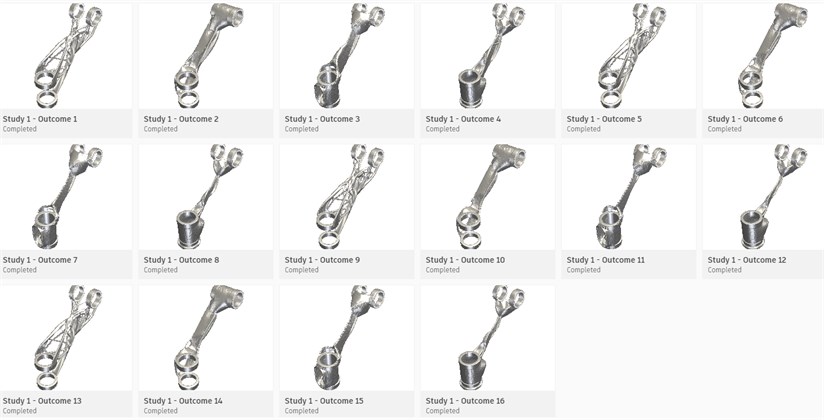
These created models are models prepared in accordance with the demands of the user. Through these models, the user is discovered the appropriate model by filtering and observation with the appropriate criteria. In this model, our biism criterion reveals our preference for being light and durable, and it is possible to conduct research on the Fusion 360 program by filtering suitable models. The models created in Fig. 8 were placed on the chart according to the safety factor and mass. In this graph, it is possible to compare the appropriate model by choosing according to the strength and lightness criteria it is looking for.
Fig. 8Graphing the designed models according to the appropriate criteria
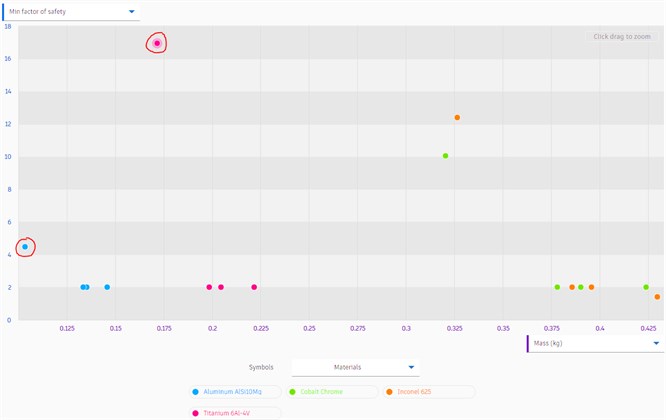
In the graphic of Fig. 8, ensuring that two design products are selected and compared in terms of high strength and lightness, enables the designer to make a more meaningful and easy decision. The designer ensures that these models are chosen visually, by discovering the most suitable model among these features offered by the program such as physical sizes, mechanical strength values and production costs. When the obtained results are examined, it is possible to be preferred by the designer because the maximum stress that can occur on the model created from aluminum material is 53.5 MPa and the safety factor is 4.49, and the model that occurs is 103 g with the lightest and accordingly the production cost is low.
Fig. 9Comparison of artificial intelligence models: a) Aluminum, b) Titanium material
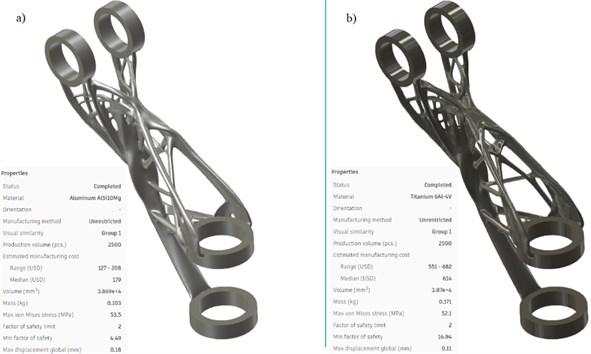
4. Conclusions
In this study, it was ensured that a piece used as bicycle girdon neck was modeled with artificial intelligence-supported design, generative design. With this model that emerged in this way, it was provided to create a model that provides the desired mechanical strength and lightness under the desired conditions (Fig. 10). This design uses machine learning to mimic nature's approach to design. Designers or engineers define design parameters (such as material, size, weight, power, manufacturing methods), and the software quickly searches for all possible combinations of solutions by creating hundreds or even thousands of design options. In this way, an innovative approach style for the designer to solve these complex engineering problems emerges.
Fig. 10Modeling of bicycle handlebar neck with generative design and assembly view
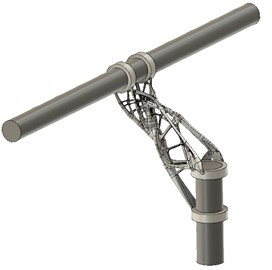
The development of modern engineering systems has brought increasing complexity and uncertainty over time. Sometimes it can take a lot of time to resolve these uncertainties with the classical approach. With the development of artificial intelligence technology, it evolves from the classical design process of the designers to the innovative design process, making use of machine learning to mimic nature’s approach to design. In this way, designers or engineers enter design parameters (such as material, size, weight, power, manufacturing methods, and cost constraints) into productive design software, and the software quickly creates hundreds or even thousands of design options to investigate all possible combinations of solutions. From there, designers or engineers can filter and select results to best meet their needs. In the near future, the items we use everyday, the vehicles we travel, the layout of our daily work environment and more can be created using generative design.
References
-
Wu Y., Zhou Y., Zhou Z., Tang J., Ouyang H. An advanced CAD/CAE integration method for the generative design of face gears. Advances in Engineering Software, Vol. 126, 2018, p. 90-99.
-
Zhang B., Goel A., Ghalsasi O., Anand S. CAD-based design and pre-processing tools for additive manufacturing. Journal of Manufacturing Systems, Vol. 52, 2019, p. 227-241.
-
Traditional Design. 16.05.2020, https://knowledge.autodesk.com/.
-
Giraud Moreau L., Lafon P. A comparison of evolutionary algorithms for mechanical design components. Engineering Optimization, Vol. 34, Issue 3, 2002, p. 307-322.
-
Krish S. A practical generative design method. Computer-Aided Design, Vol. 43, Issue 1, 2011, p. 88-100.
-
Toptaş E., Akkuş N. Damage detection of carbon fibers in filament winding machines using an electrical resistance method. The International Journal of Advanced Manufacturing Technology, Vol. 93, Issues 1-4, 2017, p. 671-679.
-
Oh S., Jung Y., Kim S., Lee I., Kang N. Deep generative design: Integration of topology optimization and generative models. Journal of Mechanical Design, Vol. 141, Issue 11, 2019, p. 111405.
-
Shea K., Aish R., Gourtovaia M. Towards integrated performance-driven generative design tools. Automation in Construction, Vol. 14, Issue 2, 2005, p. 253-264.
-
Akkuş N., Toptaş E., Topal O. Thermomechanical analysis of arc welded joint by finite element method. International Congress on Advances in Welding Science and Technology for Construction, Energy and Transportation Systems, 2011.
-
Lee L.-C.-J., Lin M.-H. Experimental research in applying generative design and 3D printers in user participating design. International Conference of Design, User Experience, and Usability, 2014.
-
Yildiz A. R., Abderazek H., Mirjalili S. A comparative study of recent non-traditional methods for mechanical design optimization. Archives of Computational Methods in Engineering, 2019, https://doi.org/10.1007/s11831-019-09343-x.
-
Özsoy K., Duman B., İçkale Gültekin D. Metal part production with additive manufacturing for aerospace and defense industry. International Journal of Technological Science, Vol. 11, Issue 3, 2019, p. 201-210.