Abstract
Percutaneous auricular vagus nerve stimulation (pVNS) is a novel approach of treating cardiovascular and inflammatory diseases, as well as pain and neurological conditions. The treatment can be optimized by using biosignals as objective measures and feedback-control. One suitable biofeedback could be the use of pulse rate and pulse rate variability (PRV) derived from optical pulse plethysmography (PPG) instead of heart rate and heart rate variability (HRV) derived from electrocardiogram (ECG). For this purpose, a single-lead ECG on the thorax and a PPG on the earlobe were measured simultaneously on 10 healthy subjects for 420 s during three different respiratory phases. The data was analyzed and compared with scatterplots, the Pearson correlation coefficient and a Bland-Altman analysis. The outcomes show a very high correlation of heart rates from PPG and ECG ( 0.9663) and SDNN values ( 0.9791). Comparison of RMSSD values showed a high positive correlation ( 0.7963) but a mean overestimation of 10 ms in RMSSD values measured with the PPG. The results presented suggest that PRV could be and alternative biofeedback used in pVNS.
1. Introduction
Percutaneous auricular vagus nerve stimulation (pVNS) is an innovative technique in recent clinical research [1]. As the predominant nerve of the parasympathetic nervous system, the vagus nerve represents an essential interface to the regulatory effects of the autonomous nervous system (ANS). The ANS ensures homeostasis by regulating the blood circulation (dilation and constriction of blood vessels), the anti-inflammatory response, the function of internal organs and controls the heart rate (HR) and heart rate variability (HRV) [2]. The electrical stimulation of auricular vagal afferents initiates an efferent modulation, resulting in physiological changes that can be used in the treatment of cardiovascular and inflammatory diseases [3-5], neurological conditions [6, 7] and pain [8]. Compared to surgical procedures and medicamentous treatment, pVNS is a minimally-invasive technique that comes with low risk factors and side-effects and a high overall treatment satisfaction [9].
Since pVNS is an innovative treatment, objective measures for stimulation efficacy in different indications still have to be established. One approach is the measurement of biosignals like HRV, optical pulse plethysmography (PPG) and blood perfusion index during the stimulation [10, 11]. Furthermore, feasible biosignals could be used in an individualized feedback-controlled stimulation to adjust stimulation characteristics for each patient. Therefore, a biosensor could be integrated into the stimulation device that is placed behind the auricle in order to measure the pulse rate variability (PRV). This paper discusses the level of agreement between the HRV measured on the thorax and the PRV measured on the earlobe to evaluate the feasibility of this approach.
2. Methods
The data presented was obtained at the Vienna University of Technology. For the measurement, ten healthy subjects were recruited. After obtaining a written informed consent, the test procedure was explained to the individual subjects and the demographic data was recorded (Table 1). The subjects were placed in an upright seated position and asked to follow the measurement protocol. The protocol was divided into three respiratory phases: normal respiration (300 s), deep respiration (30 s) and voluntary apnea (40-60 s depending on the subject).
The PPG was detected on the earlobe with a custom-made light-transmission sensor. A continuous single-lead ECG was measured on the thorax with three Ag/AgCl electrodes. Both signals were recorded simultaneously at a sampling rate of 1kHz using the BIOPAC MP36 Acquisition Unit (BIOPAC Systems Inc., Goleta, CA, USA). The ECG R-peaks and PPG maximum peaks were detected with a proprietary algorithm and manually cleared from occurring movement artefacts. HRV/PRV time domain parameters [12] SDNN (total variability) and RMSSD (parasympathetic influence on variability) were calculated in 60 s windows with an overlap of 80 %. The heart intervals (HI), pulse intervals (PI) and HRV/PRV parameters were analyzed with scatterplots, the Pearson correlation coefficient (PCC) and a Bland-Altman analysis (BAA). The limits of agreement were set to 1.96 standard deviation of mean differences, which corresponds to a 95 % confidence interval. All data processing was performed using MATLAB 2016b (The MathWorks Inc., Natick, MA, USA).
3. Results
The study population and duration of voluntary apnea are shown in Table 1. The subjects were aged 29± 4.5 years and had a mean BMI of 23.3±3.1 kg/m2. Of the ten subjects, two were female and two were smokers. None of the subjects had chronic health conditions.
Fig. 1(a) shows a scatterplot of ECG HIs and PPG PIs with the corresponding PCC of 0.9663. The linear regression and PCC indicate a very high positive correlation between HI and PI. The average of ECG and EFPG samples are in a range between 0.6 s (100 bpm) and 1.2 s (50 bpm). The differences of both samples as shown in Fig. 1(b) lie mainly within the limits of agreement between -0.074 s and +0.073 s. The mean difference between ECG and PPG values is 0.68 ms.
Table 1Demographic data and duration of voluntary apnea during measurement
Subject | Demographic data | ||||||
Sex | Age | Height (cm) | Weight (kg) | Smoker | Diseases | Duration of apnea (s) | |
P01 | m | 27 | 180 | 80 | n | n | 60 |
P02 | w | 27 | 172 | 65 | y | n | 40 |
P03 | m | 26 | 186 | 80 | n | n | 45 |
P04 | m | 40 | 174 | 70 | n | n | 45 |
P05 | m | 26 | 185 | 70 | n | n | 60 |
P06 | m | 31 | 176 | 95 | n | n | 60 |
P07 | m | 25 | 176 | 69 | n | n | 50 |
P08 | m | 28 | 187 | 83 | y | n | 60 |
P09 | w | 28 | 175 | 60 | n | n | 60 |
P10 | m | 32 | 193 | 85 | n | n | 60 |
Fig. 2(a) and (b) give the scatterplots of the HRV and PRV with the PCC values of 0.9791 and 0.7963, respectively. The PCC and linear regression in Fig. 2(a) indicate a very high positive correlation between SDNN values of ECG and PPG, whereas Fig. 2(b) shows a high positive correlation between the respective RMSSD values. The lower PCC is caused by an overestimation of RMSSD values measured with the PPG as shown in the scatterplot (Fig. 2(b)).
Fig. 1a) Scatterplot of ECG HIs and PPG PIs with PCC; b) Bland-Altman plot with average of ECG His and PPG PIs on the x-axis and differences between HI and PI on the y-axis (PIs were subtracted from HIs), limits of agreement (red lines) and mean average (blue line)
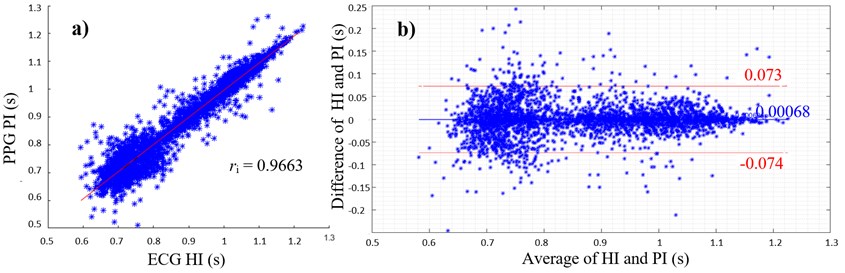
Fig. 2a) Scatterplot of ECG and PPG SDNN values with PCC; b) scatterplot of ECG and PPG RMSSD values with PCC; c) Bland-Altman plot with average of ECG and PPG SDNN values on the x-axis and differences between SDNN values on the y-axis (PPG SDNN values were subtracted from ECG SDNN values); d) Bland-Altman plot with average of ECG and PPG RMSSD values on the x-axis and differences between RMSSD values on the y-axis (PPG RMSSD values were subtracted from ECG RMSSD values), limits of agreement (red lines) and mean average (blue line)
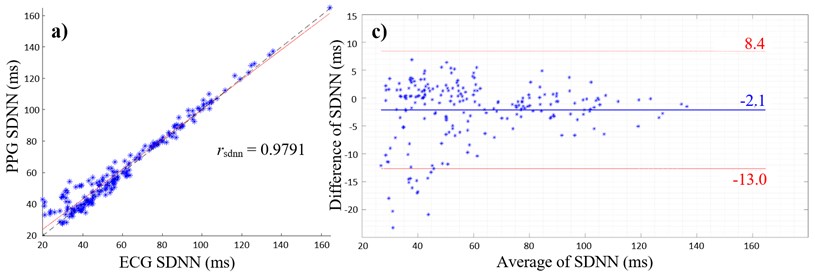
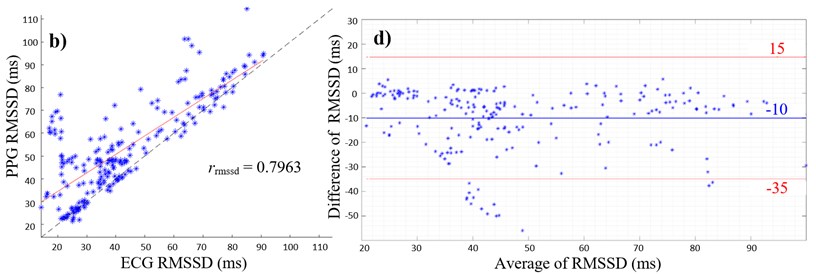
Furthermore, overestimation of HRV parameters are shown in Fig. 2(c) and (d), where the mean differences are both negative values with the mean of SDNN of –2.1 ms (Fig. 2(c)) and the mean of RMSSD of –10 ms (Fig. 2(d)). Values outside the 95 % confidence interval only occur below the lower limit of agreements (Fig. 2(c) and (d)).
4. Conclusions
The results presented in this paper indicate a strong correlation between the selected HRV and PRV parameters, as well as the heart and pulse intervals. These outcomes are consistent with previous studies. In 2011, C. S. Bulte et al. conducted a study to compare the level of agreement between HRV and PRV in healthy subjects [13]. The PRV was derived from continuous blood pressure measurements on the finger. The respective HRV parameters were all in the frequency domain (VLF, LF and HF). The outcomes showed a nearly perfect positive correlation (Spearman correlation coefficient 0.99) and a mean difference of 1 % for the very low and low frequency power and 14 % for the high frequency parameters. In a recent study, W. Shuai et al. compared the heart rate obtained from a standard ECG to the pulse rate obtained with an oscillometer blood pressure device [14]. The outcomes of this study also showed a high positive correlation ( 0.800) between heart and pulse rate.
Regarding RMSSD values, the correlation coefficient showed a high positive correlation, however, the BAA showed an overestimation of 10 ms in the PPG signal compared to the ECG signal. Small changes in PIs can result in a greater deviation of RMSSD values, since the measurement error is squared in the RMSSD calculation [12]. Changes in PI might occur because of small movement artefacts and because of the shape properties of the PPG signal. Artefacts can be reduced by measuring the PPG during sleep. The RMSSD corresponds to variance of R-R intervals and is a predictor for fast changes of the circulatory system that are evoked by vagal activity [15]. It is possible that fast changes in HRV – represented by the RMSSD – are better detected with an electrical biosignal like the ECG than with a mechanical signal like the PPG. It can be assumed that the HIs are more accurately detectable, since R-peaks are more prominent than the maximum peaks of a pulse wave. Experiments with an increased window size of 5 minutes are also expected to bring more accurate results [15].
Overall, the outcomes presented in this paper, in connection with previous studies, suggest that the PRV can be a suitable alternative to the HRV used as objective measure and biofeedback in pVNS. The auricular pVNS is a minimally-invasive approach of treating a vast range of patients and pathologies. The treatment may be individually adjusted and optimized when PRV biofeedback is integrated into the stimulation device.
References
-
Kaniusas E., Kampusch S., Tittgemeyer M., Panetsos F., Gines R. F., Papa M., et al. Current Directions in auricular vagus nerve stimulation II – an engineering perspective. Frontiers in Neuroscience, Vol. 13, 2019, p. 772.
-
Mccorry L. K. Physiology of the Autonomic Nervous System. The American Journal of Pharmaceutical Education, Vol. 71, Issue 4, 2007, p. 78-85.
-
Széles J. C., Kampusch S., Kaniusas E. Peripheral blood perfusion controlled by auricular vagus nerve stimulation. Proceedings of 17th International Conference on Biomedical Engineering, 2013, p. 73-77.
-
Hackl G., Prenner A., Jud P., Hafner F., Rief P., Seinost G., Pilger E., Brodmann M. Auricular vagal nerve stimulation in peripheral arterial disease patients. VASA, Vol. 46, Issue 6, 2017, p. 462-470.
-
Kampusch S., Thürk F., Kaniusas E., Szeles J. Autonomous nervous system modulation by percutaneous auricular vagus nerve stimulation: Multiparametric assessment and implications for clinical use in diabetic foot ulcerations. Proceedings of IEEE Sensors Applications Symposium, 2015, p. 79-84.
-
Bauer S., Baier H., Baumgartner C., Bohlmann K., Fauser S., Graf W., Hillenbrand B., Hirsch M. Transcutaneous vagus nerve stimulation (tVNS) for treatment of drug-resistant epilepsy: a randomized, double-blind clinical trial (cMPsE02). Brain Stimulation, Vol. 9, Issue 3, 2016, p. 356-363.
-
Kampusch S., Kaniusas E., Széles J. C. Modulation of muscle tone and sympathovagal balance in cervical dystonia using percutaneous stimulation of the auricular vagus nerve. Artificial Organs, Vol. 39, Issue 10, 2015, p. 202-212.
-
Sator-Katzenschlager S.-M., Michalek-Sauberer A. P-Stim auricular electroacupuncture stimulation device for pain relief. Expert Review of Medical Devices, Vol. 4, Issue 1, 2007, p. 23-32.
-
Kampusch S., Kaniusas E., Thürk F., Felten D., Hofmann I., Széles J. C. Device development guided by user satisfaction survey on auricular vagus nerve stimulation. Current Directions in Biomedical Engineering, Vol. 2, Issue 1, 2016, p. 593-597.
-
Kaniusas E., Szeles J. C., Materna T., Varoneckas G. Adaptive auricular electrical stimulation controlled by vital biosignals. Proceedings of the 2nd International Conference on Biomedical Electronics and Devices, 2009, p. 304-309.
-
Kaniusas E., Szeles J. C., Varoneckas G. Validation of auricular electrostimulation by heart rate variability and blood perfusion: possibilities and restrictions. Proceedings of the 26th International Conference on Microelectronics, 2008, p. 180-184.
-
Malik M. Heart rate variability – standards of measurement, physiological interpretation and clinical use. European Heart Journal, Vol. 17, 1996, p. 354-381.
-
Bulte C. S., Keet S. W., Boer C., Bouwman R. A. Level of agreement between heart rate variability and pulse rate variability in healthy individuals. European Journal of Anaesthesiology, Vol. 28, Issue 1, 2011, p. 34-38.
-
Shuai W., Wang X., Hong K., Peng Q., Li J. X., Li P., Cheng X. S., Su H. How to estimate heart rate from pulse rate reported by oscillometric method in atrial fibrillation: the value of pulse rate variation. International Journal of Cardiology, Vol. 222, 2016, p. 1022-1026.
-
Shaffer F., Ginsberg J. P. An overview of heart rate variability metrics and norms. Frontiers in Public Health, Vol. 5, 2017, p. 258.