Abstract
As an renewable and clean energy of the world, wind energy has gained more and more attention and its fault diagnosis becomes more and more important. The gearbox, as the kernel component of the wind turbine system, it’s robust conditions have a great influence on the whole wind turbines system. Wind turbine gearbox has complex structure, which is usually composed of solar planetary gearbox and cylindrical gearbox. In the process of operation, various kinds of faults easily occur, resulting in serious losses. Once the wind turbine gearbox is not functioning as smoothly as it could be, it may result in large economic losses for the company and owner. At the same time, the failure rate of wind turbine gearbox has always been high because of complicated mechanic structure and special motion. Therefore, the tasks of reducing the downtime and increasing the productivity of wind turbine gearbox are urgent. This paper reviewed some research results of faults diagnosis on wind turbines gearbox, such as time-frequency analysis method, vibration based methods, nondestructive testing methods, etc. Meanwhile, this paper finds out some key problems and the channel of the resolution of the issue in order to supply some information for the further research of wind turbines gearbox.
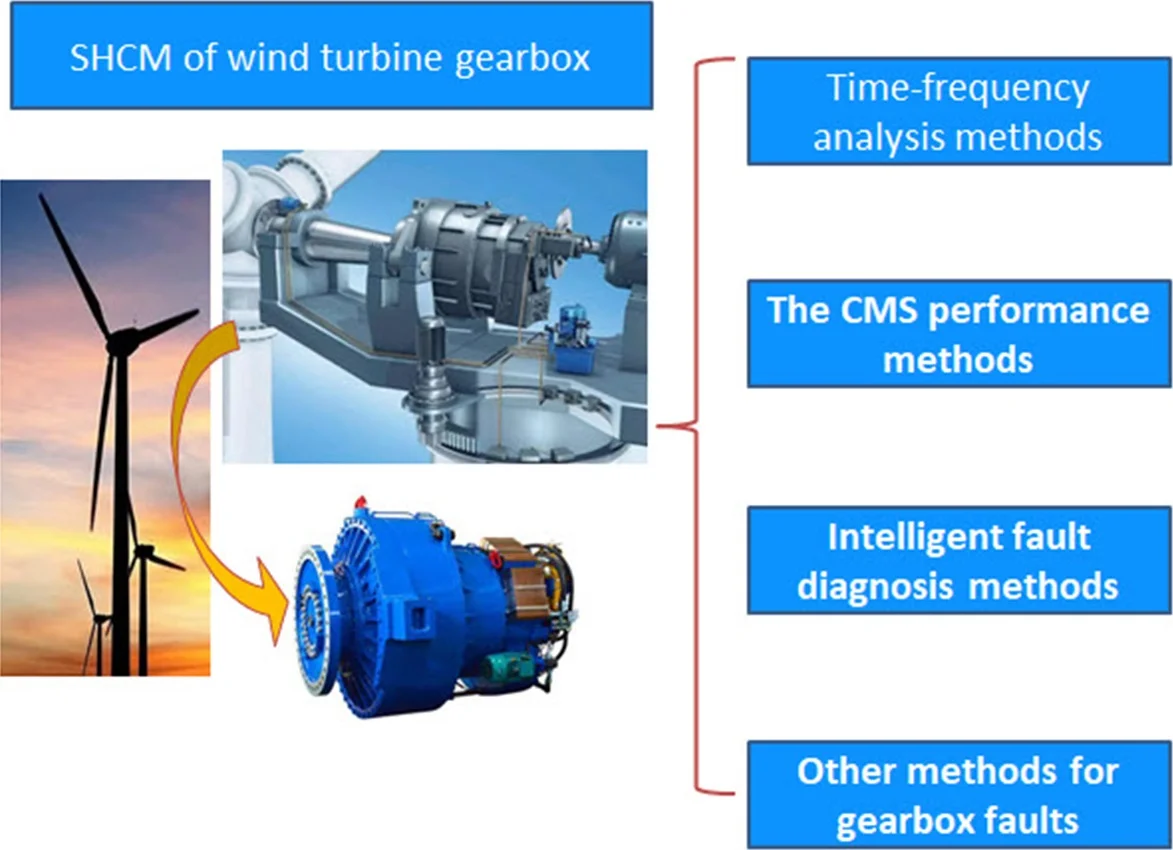
Highlights
- Wind turbine gearbox conditions have a great influence on the whole system.
- High speed shaft bearing failure plays an important role in the condition monitoring.
- Vibration-based methods on wind turbine gearbox are more common such as time-frequency analysis methods.
- Some new intelligent methods combining fault feature extraction technologies with fault recognition technology have high performance.
1. Introduction
Wind harvesting via wind turbines has been growing explosively in recent years [1], which plays an important role in optimizing energy structure. For investors, the main aim of wind turbines is creating the maximum economic benefits. Therefore, this need wind turbine can meet the requirements of more yield power and less downtime. That is to say, the research aims are increasing productivity and reducing operating costs. But, various failures of wind turbine become a powerful resistance factor to achieve the goal [2]. The most of wind turbines have been established in the remote areas, such as offshore and no man’s land, because these areas have abundant wind source. These workplaces of wind turbines don’t conducive to perform operational and maintenance works to some extent. It is estimated that the operational and maintenance costs of wind turbines account for about 25 %-30 % of costs or 75 %-90 % of the investment costs for a wind turbine of 20-years life cycle [3, 4]. At the other hand, as 2MW-type turbines appears in 2002, it reveals that the future trend is large-scale wind turbines. Compared with 750 KW machines, the operations and maintenance costs of 2 MW-type wind turbine might be decreased 12 % [2]. But, it remains challenging to keep normal running so as to minimize operational and maintenance costs and maximize productivity.
The typical faults in wind turbines generally fall into six categories: blade faults, gearbox faults, generator faults, bearing faults, mechanical braking system faults and pitch-controlled system faults. It is worth mentioning, because of running in poor working conditions in long time and complex structure, wind turbines gearbox fault diagnosis have been a significant challenge, especially decreasing fault frequency and downtime. More specifically, as the core component of wind turbine system, gearbox not only investment cost occupies large proportion in total component of wind turbine cost (about 18 %), but also it has been regarded as part with the highest failure rate based on statistical failure data of components. The detail failure rate and downtime as shown in Fig. 1 [5].
Fig. 1Wind turbines subassembly failure rates, downtime and failure types
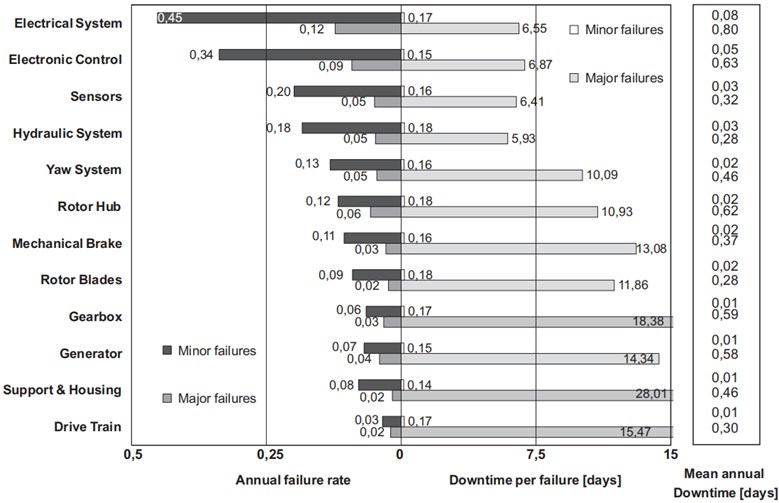
As can be seen form Fig. 1, the mean annual downtime of minor and major failures of gearbox up to 60 days, which seriously influences the productivity and efficiency of wind turbines. The picture also shows that maintenance works of wind turbines gearbox need 18.38 days to finish it which is in the second for per failure due to their size and robust link to other components making it harder to access, repair, or even replace [6]. Such long maintenance time and according another research [7-9] can fully illustrates above opinion that it is hard to repair the faults of wind turbine gearbox in the wind field. Moreover, the expected operation life of gearbox is usually publicized as 20 years from merchant. However, the true service life of gearbox can only reach six to eight years [5], which not only extra increases cost of wind turbines, but also decrease the production efficiency. In order to decrease produce and repair cost of wind turbines, direct-drive wind turbines are utilized to avoid the failures of gearbox so as to minimize maintenance cost [10]. This practice did not achieve the ideal results, the total failure rate of the electronic components in direct-drive wind turbines is 33 % higher than that of the gearboxes in indirect-drive wind turbines, especially the failure rate of large direct-driven wind turbines is up to double [11]. What’s more, the share of global wind turbines installations accounted for by gearbox-based wind turbines is 74 %, and it shows that gearbox-based wind turbines have enormous advantages compared with direct-drive wind turbines [12]. So, fault diagnosis of wind turbines gearbox is an essential part in the process of development of wind turbines in the further [13, 14]. The problem that provide the mature technology to largely reduces downtime and avoid catastrophic incidents need to be solved urgently [15]. The purpose of this paper is to analyze the different components of wind turbines gearbox in order to detect the faults that may happen, and then some present comparison methods can be used in diagnosing the faults when facing different faults that happen in gearbox.
The organization of the paper is as follows: Section 1 presents the main structure of some typical wind turbines gearbox. The second part mainly completes the main structure analysis of the gearbox of wind turbine, and what faults will happen to the gearbox of wind turbine, and what are the basic characteristics of these faults. Section 3 difficulties of fault diagnosis of wind turbine gearbox introduces. Section 4 analyzed the structure healthy condition monitoring (SHCM) methods according to turbine faults’ categories. Section 5 analyzed the advantages and disadvantages in the nowadays fault diagnosis (FD) methods. Some conclusions are given in the last section.
2. Typical faults in wind turbines gearbox
From the transmission principle of the wind turbine, it can be seen that the speed of the blade does not match the rated speed of the generator. Therefore, the transmission of wind power to the generator is used to achieve the corresponding input speed. At present, in many commercial wind turbine systems, fixed transmission ratio gearbox is used to connect turbine rotor and generator. A variable ratio gearbox (VRG) allows wind turbine, with a constant-speed generator, to discretely vary rotor speed and to achieve greater aerodynamic efficiency [16]. Generally, the gearbox is designed as sun and planetary gear with parallel gear structure to obtain optimum input speed and transmission ratio. The most common wind turbine gearboxes have one or two planetary gear stages and two or one parallel stage [17]. The structure of the 1.5MW-type wind turbine gearbox as shown in Fig. 2 [18].
Fig. 2The structure of the 1.5 MW-type wind turbine gearbox
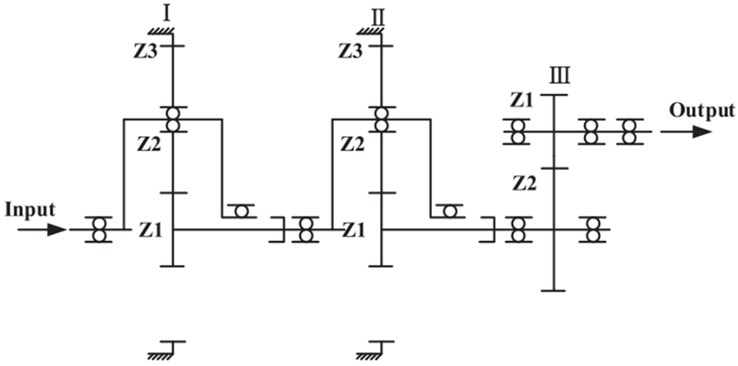
As can be seen from Fig. 2, the internal structure of the wind turbine gearbox is quite complex. Once the components fail, the whole system will not work properly because of chain reaction. In addition, wind turbines have long been under severe conditions such as heavy load, gust and dust corrosion, which makes the gearbox of wind turbines prone to failure. The faults in wind turbines gearbox can be classified into two categories: wear-out failures and temporary random faults [12]. One step further, the detail faults of wind turbines gearbox can be described as gear damage, bearing damage, broken shaft, leaking oil and high oil temperature, and poor lubrication, etc. [2, 19, 20].
2.1. Typical blade faults
For some reasons, wind turbines work directly in the wind, rain, snow and other harsh environments. The diameter of wind turbine blades becomes bigger and bigger in order to capture more wind energy. This makes it easy to lead to some faults directly on the blades, such as the rotor imbalance, blades and hub corrosion, crack, and serious aeroelastic deflections in large wind turbines [21].
2.2. Typical gearbox faults
The main function of gearbox is to transmit wind power into the generator to reach the corresponding input speed. Currently, most of the commercially available wind turbines have been operated using a fixed-gear ratio between the turbine rotor and electrical generator. A variable ratio gearbox (VRG) allows wind turbine, with a constant-speed generator, to discretely vary rotor speed and to achieve greater aerodynamic efficiency [22, 23]. In order to obtain higher input speed and transmission ratio, the gearbox is commonly designed as sun and planetary gear with parallel gear structure. Because of the longtime running in poor working conditions such as heavy load, wind gust or dust corrosion, failures of the wind turbine gearbox are various, such as shaft imbalance, shaft misalignment, shaft damage, bearing damage, gear damage, broken shaft, leaking oil, high oil temperature, and poor lubrication, etc.
2.3. Typical generator faults
Generator can transform mechanical energy into electricity energy directly. There are kinds of generator from the structure side, such as cage induction generator, brushless doubly-fed generator, Alternating Current (AC) excited generator, synchronous generator, etc. In these types, double-fed asynchronous motors are commonly used by most generator manufacturers. Generator’s long-term operation in the electromagnetic environment may cause some common failures as follows [24]: generator excessive vibration, generator overheating, bearing overheating, abnormal noises and insulation damage, etc.
2.4. Typical bearing faults
Bearings are essential components of wind turbines as in other rotation machines, especially in generators and gearboxes. Dynamic and unpredictable stress may cause premature wear in bearing, which will lead to the increase of maintenance cost, even lead to sudden expensive turbine breakdowns. Overheating is another typical fault in wind turbine bearings, and can impact the performance of running turbine bearings [2].
2.5. Mechanical braking system faults
The mechanical brake is generally applied after the blade furling and electromagnetic braking (where fifitted) have reduced the shaft speed. Otherwise, if lacking the mechanical brake, the component would rapidly be worn down if it is directly used to brake the turbine from full speed. In most wind turbine countries, wind turbines are required by law to obtain two independent fail safe brake mechanisms to stop the turbine promptly when required. On the other hand, failures within the braking process, for example when the speed exceeds the limit, can cause serious damages to the main structure, especially the mechanical units and blades. Failures within the hydraulic unit will also result in the brakes remaining on and increase the downtime [2].
2.6. Pitch-controlled system faults
The number of faults occurred in pitch-controlled system of wind turbine just follows the electrical system. It also account for a large proportions of the longest machine halt time in parts and single failure longest downtime. Moreover, due to complexity and ambiguity, it is difficult to describe relationships between mechanism and faults caused by mathematical models in the pitch controlled system [2].
The cost distribution over the different failure modes for a wind turbine gearbox population as shown to Fig. 3 [25]. To keep a better development of wind turbines, gearbox work w ell has thus become an increasingly important problem.
Fig. 3Cost distribution over the different failure modes for a wind turbine gearbox population
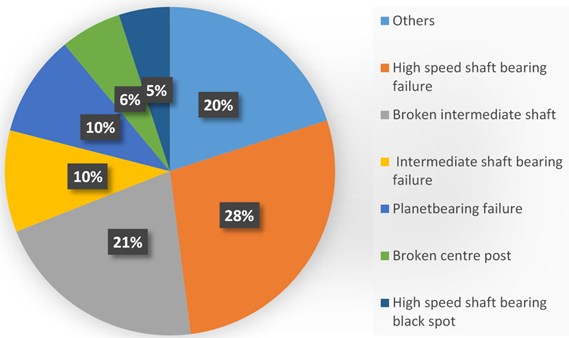
3. Difficulties of wind turbine gearbox fault diagnosis
The gearbox of wind turbine is generally considered to be composed of solar gears, planetary gears, gear rings and planetary frames. Compared with the fixed-axis rotating gearbox in structure, the gearbox of wind turbine is quite different. At the same time, the motion principle of planetary gearbox fixed axis rotating gearbox is determined by its structure in completely different ways. Usually, the ring gear is fixed, the sun gear revolves around its own central axis, and the planetary gear not only revolves around its own central axis, but also revolves around the central axis of the sun gear, meshing with the sun gear and the ring gear. Complex motion characteristics help to generate vibration signals, including characteristic frequencies of different components, vibration coupling elements of power input and load equipment, and noise interference elements. At the same time, the difficulties of fault diagnosis of wind turbine gearbox are as follows.
The arrangement of sensors is limited by the compact structure of wind turbine room. In the process of signal acquisition, many effective means are used to collect and transmit vibration signals. That is to say, different sensors are used according to the test target, such as low-speed accelerometer to test low-speed axis, and common accelerometer to test high-speed axis. In addition, the complex transmission path of the wind turbine gearbox can attenuate the vibration signal and weaken the vibration response of the fault.
The vibration signal of wind turbines gearbox has the frequency modulation characteristic when various frequency components are coupled [26]. So, vibration signal of wind turbines gearbox shows the nonlinear characteristic.
Generally speaking, when we collect vibration signals, the acceleration sensor is installed in the fixed position of the planetary gearbox of the wind turbine. The relative distance between accelerometers and the meshing position between solar gear and planetary gear, planetary gear and gear ring are time-varying, which causes the change of vibration transmission path. This phenomenon is called amplitude modulation. Therefore, the vibration signal of fan gearbox presents AM phenomenon, showing non-stationary characteristics, which increases the difficulty of fault diagnosis of fan gearbox [27]. Meanwhile, the varying loads of wind turbines contribute to intensify this characteristic, especially large-scale varying loads when the wind turbine instant start.
The vibration signals of transmission system of wind turbines disturbed by background noise is inevitably. Unfortunately, the vibration signal of wind turbines gearbox is polluted by low-frequency noise, which make difficult to extract fault feature.
As can be seen form above difficulties of fault diagnosis of wind turbine gearbox, which definitely hinders the development of fault diagnosis of wind turbines gearbox. So, it is important to find an effective method to solve the difficulties of fault diagnosis of wind turbine gearbox.
4. Wind turbine gearbox fault diagnosis methods
As mentioned above, the operation and maintenance cost of wind turbine gearbox occupies a large proportion in the whole wind turbine system, and the fault of wind turbine gearbox is difficult to diagnose. Therefore, in order to ensure the normal operation of wind turbines, it is necessary to establish fault diagnosis methods. In recent years, due to the attention paid by some scholars to the fault diagnosis of wind turbine gearbox, many solutions have been put forward and some results have been achieved. The results are as follows.
4.1. Time-frequency analysis methods
Vibration analysis is an effective method for fault diagnosis of transmission chain system of wind turbines and has been widely used. However, due to the above difficulties in fault diagnosis of wind turbine gearbox, it is difficult to accurately diagnose planetary gearbox faults.
Faced these difficulties, many scholars have conducted a series of studies in recent years. Wenyi Liu reviewed the noise mechanism and de-noising techniques in wind turbines of the recent years [28], he also put forward a multi-dimensional kernel domain spectrum (MD-KDS) method to conduct intelligent wind generator fault detection [29]. A time-frequency analysis based on Vold-Kalman filter and higher order energy separation for fault diagnosis of wind turbine planetary gearbox under nonstationary conditions was proposed by Zhipeng Feng [30]. Feng also introduced adaptive optimal kernel time- frequency analysis method to deal with wind turbine planetary gearbox fault under non-stationary conditions [31, 32]. In order to resolve time-varying gear fault features, Feng also conduct an Time-Frequency emodulation analysis via Vold-Kalman filter for wind turbine planetary gearbox fault diagnosis under nonstationary speeds and the proposed time-frequency analysis method is further extended to generate time-varying amplitude and frequency demodulated spectra [23]. A novel order tracking method based on discrete spectrum correction technique is proposed by Guolin He to analyze wind turbine gearbox vibration for the purposes of health monitoring and fault diagnosis [18]. Zhixiong Li used a supervised bounded component analysis method to detect gear cracks in a complex gearbox of wind turbines [33]. Bingchang Hou provided a novel tacholess order tracking method based on generalized demodulation (GD) for WT fault detection. A multi-channel sensor system is applied in the signal acquisition to solve issues relating to its installation position and direction of a single sensor. A new filtering algorithm based on the meshing resonance phenomenon is proposed by Tianyang Wang [34]. Aiming to extract the fault feature of planet gear effectively, Yun Kong propose a novel feature extraction method based on spectral kurtosis and time wavelet energy spectrum [35]. Jinjiang Wang presented a fault component separation method that integrates ensemble empirical mode decomposition with independent component analysis to address the problem of low signal-to-noise ratio [36]. Multi-fault detection has been a challengeable task for fault feature extraction, to address it, Wei Teng introduced a multi-fault detection and failure analysis method based on complex wavelet transform [37]. Zhaohui Du also presented sparse feature identification method based on union of redundant dictionary to deal with wind turbine gearbox multi-fault conditions [38]. In order to addressed the challenging issue that under-determined blind source separation (BSS) of nonlinear mixed signals in multiple-fault detection of wind turbine gearbox, Yang Qian presented a solution through a combination of empirical mode decomposition (EMD) and kernel independent component analysis (KICA) methods [39]. Aijun Hu proposed approach based on ensemble intrinsic time-scale decomposition and Wigner bi-spectrum entropy to extract the fault features and recognize the gearbox fault types when two or more fault features are close to each other [40]. Aimed at the planetary gearbox early failure detection, Liu Hong introduced Fourier series analysis is and robust feature extraction algorithms [41]. Ma Zhiyong also presented the multi-scale enveloping spectrogram method to detect weak faults [42]. Labar Hocine used wind turbine gearbox fault diagnosis method based on symmetrical components and frequency domain [43]. Yingning Qiu presented a first attempt to use Dempster-Shafer (D-S) evidence theory for the fault diagnosis of wind turbine (WT) on SCADA alarm data and proposed a procedure of multi-dimensional information fusion for WT fault diagnosis [44]. To solve the problem of the traditional fault detection methods which are based on constant operating speed assumption will be invalid in a complex situation, Bingchang Hou provided a novel tacholess order tracking method based on generalized demodulation (GD) for WT fault detection [45]. While using the novel modulation model to support the fault diagnosis of the planetary stage of a wind turbine gearbox, the fault feature of planetary stage with lower rotational speed is easily concealed by the meshing vibration energy of ordinary stages with higher speed, to resolve this problem ,Wei Teng submit it is effective to utilize empirical wavelet transform to adaptively find weak fault frequency in planetary stage as well as evident fault characteristics in other ordinary stages [46]. Wei Teng also proposed a tunable Q-factor wavelet transform based sparse representation method is proposed, which integrates the property of tunable Q wavelet transform, non-convex penalty and noise optimization into sparse decomposition [47]. Stephan Schmidt claimed a new method, referred to as the IFBIagram, which can be capable of identifying frequency bands that are rich with diagnostic information related to specific cyclic components. This allows the optimal frequency band to be determined for diagnosing the component-of-interest [48].
The basic idea of these methods is to process the signals collected by the vibration acceleration sensor, and the signal processing method is introduced. Therefore, the quality of signal processing methods directly affects the analysis results. Moreover, the field conditions of wind farms are complex, and some time-frequency analysis methods are not necessarily applicable to the actual situation of all wind farms. In another word, each method will achieve better results under its specific conditions, but it is not a panacea, in some environmental conditions change, these methods may not be significant or ineffective.
4.2. The CMS performance methods
The main function of condition monitoring system (CMS) is to predict some fault modes and apply it to the gearbox of wind turbine. It must also be pointed out that CMS cannot predict every potential failure mode, so costly error alerts may occur.
So far, researchers have made important contributions to the performance of CMS. Yang Wenxian summarized the technical methods of condition monitoring for wind turbine [49]. I. Antoniadou introduced a time-frequency analysis approach to deals with the condition monitoring of wind turbine gearboxes under varying operating conditions [50]. Joel Igba developed novel techniques for fault detection using the RMS and Extreme (peak) values of vibration signals [51]. Abdulwahed Salem adopted order analysis method to avoid any catastrophic failure to the gearbox and maintain system reliability [52]. Jong M. Ha propose autocorrelation-based time synchronous averaging (ATSA) to cope with the challenges associated with the current practice of time synchronous averaging (TSA) for planet gears in planetary gearboxes of wind turbine [53]. Huageng Luo presented approaches in vibration-based wind turbine gearbox condition monitoring. Detailed spectral analysis and acceleration enveloping techniques were used to effectively extract the gear and bearing damage features. Synchronous analysis was used to accurately detect specific damage features during constantly varying operational conditions [54]. Feng Yong-xin presented a practical CM technique based on vibration analysis for faulty gear and faulty bearing detection of wind turbine gearbox [55]. Shuangwen Sheng presented first-hand oil and wear debris analysis results obtained through tests that were based on full-scale wind turbine gearboxes [56]. Qian Peng presented a single hidden-layer feed forward neural network (SLFN), trained using an extreme learning machine (ELM) algorithm, for condition monitoring of wind turbines [57]. Salem introduced an improved technique to monitor the condition of the wind turbine gearbox based on gearbox vibration and shaft torque signatures analyses [58]. With the development of condition monitoring technology, the data collected by sensors are voluminous and much faster than before, Peng Qian proposed a novel wind turbine condition monitoring method based on cloud computing. This method is not only able to detect the faults effectively, but also consider data upload quantity reduction and data security, the case proved it is effective and can also enhance economic benefit and operating efficiency of the wind farm. However, it needs to transfer a lot of data which relies on the support of high-speed information transmission technology [59]. Aimed at the problem that the signal data of wind turbine faulty gearbox is difficult to obtain and the health condition is difficult to diagnose under variable working conditions, He Ren and Wenyi Liu came up with a fault diagnosis method based on variational mode decomposition (VMD) multi-scale permutation entropy (MPE) and feature based transfer learning (FTL) [60]. In practice of condition monitoring and fault diagnosis, the collected health condition signals are very similar and contain a lot of noise, which makes the fault diagnosis of WTs more challenging. In order to handle this problem, Xiaoyin Nie approached a model called denoising stacked feature enhanced autoencoder with dynamic feature enhanced factor (DSFEAE-DF) [61]. The basic idea of these methods is to synthetically consider the field analysis data of wind farms, and introduce the data processing method to analyze the results from the monitoring data including vibration data.
The main function of CMS system in supervisory control and data acquisition (SCADA) data is to predict the possible failures. The system is shown in Fig. 4 below. As shown in Fig. 4, the whole system is composed of training module, prediction module, anomaly detection module, fuzzy expert initialization module and fuzzy expert application module. At first the data collected are preprocessed according to the suitable methodology. Then in this system the normal behavior is trained before the diagnosis. Diagnosis and classification results are at last obtained after the testing processing. Based on the application of ANN for condition monitoring in wind turbines, Bangalore presented methods for filtering of data, which ensure that the ANN models are trained on the data representing the true normal operating conditions of the wind turbine [62]. Issa Al-Tubi investigated the probabilistic risk of gear tooth flank micro-pitting in wind turbine gearboxes and shows how relatively slow rate of supervisory control and data acquisition (SCADA) data, recorded during operation, can be used to analyze the onset of gear surface damage [63]. A monitoring the health method of wind turbine gearboxes based on the lubricant pressure data in the Supervisory Control and Data Acquisition (SCADA) system was investigated by Long Wang [64].
Fig. 4Overview of the CMS system
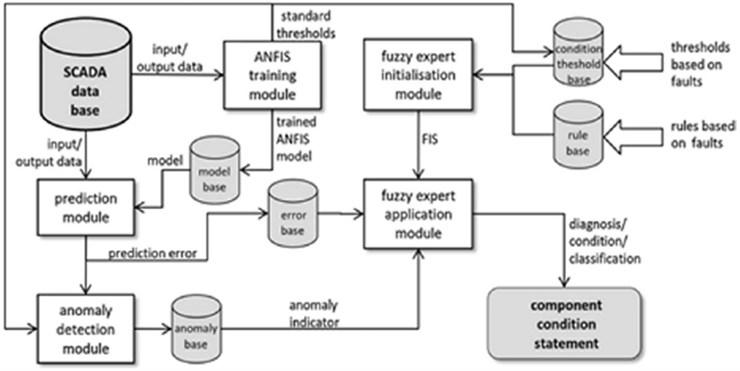
4.3. Intelligent fault diagnosis methods
Intelligent fault diagnosis is the combination of artificial intelligence and fault diagnosis, which is mainly reflected in the application of domain expert knowledge and artificial intelligence technology in the process of diagnosis. It is a system composed of people (especially experts in the field), hardware that can simulate brain function and its necessary external devices, physical devices and software supporting these hardware. At present, the theories and methods of intelligent diagnosis applied in wind turbine gearbox are mainly based on neural network, fuzzy logic, and information fusion. With the development of computer technology, more and more intelligent fault detection technologies are applied to the fault diagnosis of wind turbines gearbox.
In the last few year, many scholars have put forward some advanced and reliable methods. Silvio Simani and Cihan Turhan advanced a data driven approach that is based on neural networks and is used to implement the fault diagnosis block. This method relies on neural networks that are used to describe the strongly nonlinear relationships between measurement and faults. However, the verification and the validation of the proposed solutions by means of data acquired from real installations and for fault tolerant control applications are failed to conduct in-depth research [65]. Mingzhu Tang was devoted to the application of an adaptive LightGBM method for wind turbine fault detections. He developed an improved LightGBM fault detection approach, then a fault detection strategy for wind turbine gearboxes was investigated [66]. Given that the installed wind capacity grows, the volume of CM data increases, making manual interpretation of vibration signals challenging. Faced this, Sofifia Koukoura proposed an automated framework for gearbox incipient failure diagnosis. The framework utilises vibration signals and performs health estimation and fault isolation based on signal processing and artificial intelligence (AI) techniques. Studies have shown that this research can be used to optimise wind turbine maintenance actions [67]. As the traditional softmax loss of an LSTM network usually lacks the power of discrimination, Aijun Yin proposed a fault diagnosis method for wind turbine gearboxes based on optimized LSTM neural networks with cosine loss (Cos-LSTM). The loss can be converted from Euclid space to angular space by cosine loss, thus eliminating the effect of signal strength and improve the diagnosis accuracy. The effectiveness of the proposed method is verified with the fault vibration data collected on a gearbox fault diagnosis experimental platform [68]. When working in practical engineering applications, the operating states of wind turbine are various, accompanied by a large number of noise interference, which leads to the decline of the discrimination accuracy of intelligent diagnosis. In order to address this issue, Yuanhong Chang proposed a concurrent convolution neural network (CeCNN), the raw data is fed into the network without any prior knowledge, and the characteristics are learned directly and adaptively from the input [69]. To tackle the problem of directly applying the traditional algorithms which will cause significant degradation of performance by changing working loads of machines, Jianwen Guo presented a novel domain adaptation method, named generative transfer learning (GTL) [70]. Wenfeng Hu put forward a wind turbine intelligent fault diagnosis algorithm based on a wavelet packet transform (WPT) filter and a cross-validated particle swarm optimized (CPSO) kernel extreme learning machine (KELM) [71]. In fault detection studies, fuzzy inference can hardly detect early faults or measure fault severities due to the singleton input and the limited linguistic terms and rules. To work out this issue, Fuming Qu presented a WT fault detection method based on expanded linguistic terms and rules using non-singleton fuzzy logic. He used the generated fuzzy inputs, non-singleton fuzzy inference system (FIS) can be applied in WT fault detection. Then he proposed a mechanism of expanding linguistic terms and rules, so that the expanded terms and rules can provide more fault information and help to detect early faults [72]. Yonghao Miao introduced the encoder signal for the fault diagnosis of wind turbine gear and presented a novel adaptive filtering method, improved maximum correlated kurtosis deconvolution adjusted (IMCKDA), in order to eliminate the diverse noises in encoder signal. Additionally, to overcome the limitation from the sensibility of discontinuity point and filtered signal in traditional deconvolution methods (DMs), he also introduced convolution adjustment definition and correlated Gini index (CG) is originally designed to guide the selection of filter length [73]. The supervisory control and data acquisition (SCADA) system of a wind turbine provides valuable insights into turbine performance. In order to make full use of such valuable information, Yanting Li investigated fault diagnosis of wind turbines by using Gaussian process classifiers (GPC) to the operational data collected from the SCADA system and the comparison results show that the GPC method is able to provide more accurate fault diagnosis results than the SVM technique on average [74]. To addressed the voluntary abnormalities of various types and locations, Azzeddine Bakdi presented a data-driven algorithm for online detection of component and system faults in modern wind turbines at different operating zones [75]. The traditional time-frequency analysis technology is insufficient in the condition monitoring and fault diagnosis of wind turbines. Therefore, Zhe Wu proposed a new method of planetary gearbox fault diagnosis based on Compressive sensing, Two-dimensional variational mode decomposition (2D-VMD) and full-vector spectrum technology [76].
Intelligent fault diagnosis is one of the frontier disciplines in the field of fault diagnosis, which is developed on the basis of computer and artificial intelligence. These methods have promoted the development of wind turbine fault diagnosis technology to some extent. The advantages of neural network include parallel structure and parallel processing mode, high self-adaptability, strong self-learning ability, and strong fault tolerance. The merits of fuzzy logic are close to the human way of thinking and its results are practical. Data fusion improves the effectiveness and success rate of fault detection through information complementary, it can overcome the problems of uncertainty and low accuracy in fault recognition, and also overcome the complexity of environmental noise and external complicated excitation [76]. But at the same time, there are also some shortcoming, such as the disadvantages of neural network are as follows: its training time is not controlled, the dynamic system cannot be processed, and the reasoning explanation cannot be given by oneself. The drawbacks of fuzzy logic including difficulty to acquire fuzzy diagnostic knowledge, dependence on fuzzy knowledge base and the lack of learning ability. While The most serious problem of date fusion is the synchronous processing of massive monitoring data [76].
4.4. Other methods for gearbox faults
Time-frequency method, CMS method and intelligent fault diagnosis method provide an effective way to reduce downtime and operation and maintenance costs. However, due to the influence of variable speed and variable load operation conditions, the traditional methods still have some shortcomings in the control of wind turbines and there are also some limitations in the intelligent fault diagnosis.
With the development of fault diagnosis technology, more and more scholars have worked out other effective methods and applied them to the fault detection of wind turbine gearbox. Juan proposed an experimental study on the application of acoustic emission technology in the health diagnosis of wind turbine gearbox [77]. Fangzhou Cheng proposed a new generator stator current-based fault diagnostic method for the gearboxes in doubly-fed induction generator (DFIG)-based wind turbines under varying rotating speed conditions [78]. Lu Dingguo proposed for the fault detection of the gearbox loading conditions [79]. M. Grujicic demonstrated the use of the finite element analysis in modeling and elucidating the root cause of one of the gear failure modes (i.e. tooth bending fatigue) under a variety of normal operating and extreme wind‐loading conditions [80]. Bin Gao developed a physics-based multi-dimensional spatial-transient-stage tensor model to describe the thermo optical flow (TOF) pattern for evaluating contact fatigue damage [81]. To addressed the problems of spectral structure complexity and insensitive to the AM effect, Jae Yoon presented a method using a single piezoelectric strain sensor for wind turbines planetary gearboxes fault diagnosis [14]. Jae Yoon also presented a method using a single vibration sensor for wind turbines planetary gearboxes fault diagnosis using spectral averaging, which combines the techniques of enveloping, Welch’s spectral averaging, and data mining-based fault classifiers [82]. Joel Igba presented a systematic literature review of the current approaches of performance assessment, such as reliability and maintainability analysis of wind turbine gearboxes with a focus on the use of in-service data [13]. Jeremy Sheldon summarized algorithms and results and some conclusions and recommendations are provided that may help guide future tests and analysis efforts [83]. Yashwant Sinha discussed about industrial Wind Turbine Gearbox lubrication, its importance, applications, oil analysis method and lists constituents found in the oil [84]. F. Vanhollebeke used a combination of multi-body modelling and typical transfer path analysis (TPA) to investigate the impact of bearings on the total transfer path and the resulting vibration levels [85]. This method offered great assist on sensor. Xiang Dong analyzed the influence of these two parameters of mean wind speed and turbulence intensity on wind turbine gearbox’s fatigue life [86]. Aimed at the AE signals did not achieve ideal effect for complex structures, Wenxiu Lu used acoustic emission signals to detect planet gear fault localization for wind turbine gearbox [87]. In order to learn robust and discriminative fault feature representations through a deep network architecture for diagnosis accuracy improvement, Jiang Guoqian proposed a novel feature representation learning approach, named stacked multilevel-denoising autoencoders (SMLDAEs) [88]. To accurately detect the faults and analyze the fault signal transfer mechanism of planetary gearboxes, Huang Wentao proposed a novel method based on power flow finite element to study transfer paths [89]. Helsen Jan used experiment to investigate the bearing slip in a wind turbine gearbox during a transient grid loss event [90]. Considering both the meshing vibrations of the planet-ring and planet-sun gear pairs, Guolin He developed a mathematical model to analyse the planetary gear train's vibration response [91]. Aimed at the non-stationary and nonlinear characteristics of wind turbine vibration signals, Q. W. Gao presented a fault diagnosis method based on integral IELMD multiscale entropy and LSSVM [92].Vamsi Inturi proposed a method by using Empirical Mode Decomposition (EMD) which integrated vibration analysis and acoustic signal analysis to diagnose the gear faults [93]. The changes in the features of hardware sensor measurements, which are used in current SCADA systems, usually cannot provide reliable early alarms. In order to resolve this problem, Sikai Zhang a novel dynamic model sensor method is proposed for the SCADA data based wind turbine fault detection [94]. In order to eliminate the noise interference and extract the characteristics of the weak fault, Zhijian Wang provided a new method based on MED-LMD for the extraction of multiple faults and weak features in strong background noise [95]. Based on the application of high-power wind turbine gearbox, Le Zhang designed a three-coil induction abrasive particle sensor [96]. To detect gearbox fault early, Huanguo Chen proposed a method for an effective fault diagnosis by using improved ensemble empirical mode decomposition (EEMD) and Hilbert square demodulation (HSD) [97]. Hongsheng Su established a new stochastic differential equation model of gearbox state transition to maximize the utilization of gearboxes. This model divides the state of the gearbox into two parts: internal degradation and external random interference. Weibull distribution and polynomial approximation were used to construct the internal degradation model of the gearbox. The external random interference is simulated by Brownian motion. On the basis of the analysis of monitoring data, the parameters of the gearbox state model were solved using the Newton Raphson iterative method and entropy method [98]. Le Zhang designed a three-coil induction abrasive particle sensor based on the application of high-power wind turbine gearbox [96]. To improve SSC s performance, Chuang Sun developed a composite-graph based SSC method (CG-SSC) by introducing distance among the data points into SSC, where the L1 norm of the sparse coefficients is replaced by the product of the data distance and the sparse coefficients [99]. The complexity of many contemporary techniques is an inhibitor to autonomous fault detection and monitoring of non-stationary systems, to slove this issue, Stanley Fong presented a non-parametric, blind spectral pre-processing approach to simultaneously de-noise and extract harmonic content from nonstationary vibration signals [100].
Some of these monitoring methods start from the output voltage, current and power signals of the generator set, some from the mechanical structure, some from the fusion method, some from the vibration and acoustic signals, the main purpose is to complete the fault diagnosis and maintenance of the wind turbine gearbox.
5. Discussion
In view of the difficulties in fault diagnosis of wind turbine gearbox, this paper summarizes the fault diagnosis methods of wind turbine in recent three years, finds out the key problems, and puts forward solutions to solve the key problems. The advantages and disadvantages of this paper and the future development direction are summarized as follows.
5.1. Summary
These methods have achieved some results to a certain extent, especially the vibration analysis methods for multi-faults and early faults, which represent the direction of wind turbine gearbox fault diagnosis. Most of these methods enhance traditional methods through some innovations. Although these improved methods have achieved good diagnostic results, there are still some shortcomings in these improved methods, which need to be improved. At the same time, in order to achieve more accurate diagnosis, many methods from other academic fields are introduced, and abundant theoretical knowledge of fault diagnosis is accumulated. However, due to the characteristics of these methods, at the same time, there are some advantages and disadvantages, which affect the effectiveness of fault diagnosis. Therefore, it is still a challenge to adjust all faults quickly and accurately.
5.2. Prospect of some new methods
In view of the poor working conditions of the gearbox of wind turbine, some effective solutions are put forward. Therefore, the following research areas need to be further strengthened:
1) Sensor technology: Because of the compact structure of the fan, it is difficult to find a suitable position to place the sensor in the fan cabin. However, the main data comes from sensors. Therefore, according to the vibration mechanism of the gearbox of wind turbine, it is very important to select the sensitive point of the sensor.
2) Fault feature extraction technology: Fault feature extraction plays an important role in fault diagnosis of wind turbine gearbox. The vibration signal of wind turbine gearbox is not only non-stationary and non-linear, but also polluted by background noise. An effective algorithm should be established to solve the difficulties in fault diagnosis and fault diagnosis. That is to say, the main function of the algorithm is to remove the noise of wind turbine gearbox vibration signal. At the same time, the normal mode of wind turbine gearbox vibration signal is established, and the distance between the normal mode and noise elimination mode is found, and the improvement is made.
3) Fault identification: Many intelligent methods have been applied to recognize faults of wind turbines gearbox, such as neural network [101], expert system [102], or Support Vector Machine (SVM) [103]. Although these methods achieved some achievement in some extent, these methods all have their own drawbacks. New high-performance algorithm for wind turbines gearbox should be created and introduced.
6. Conclusions
This paper summarizes the SHCM of the gearbox of wind turbine and its fault diagnosis methods in the past few years. Aiming at the difficulties of fault diagnosis of wind turbine gearbox, the research results of time-frequency analysis, vibration analysis and non-destructive testing of wind turbine gearbox are analyzed. These documents are of great significance to the improvement of condition monitoring and prediction of wind turbine gearbox. The downtime and maintenance cost of the fan are reduced. At the same time, in order to improve the efficiency and accuracy of fault diagnosis, the advantages and disadvantages of these new application methods are compared in detail. Combining fault feature extraction technology with fault recognition technology, some new intelligent methods with high performance are proposed and introduced into fault diagnosis of wind turbine.
References
-
Lu X., Mcelroy M. B., Peng W., et al. Challenges faced by China compared with the US in developing wind power. Nature Energy, Vol. 1, Issue 6, 2016, p. 16061.
-
Liu W. Y., Tang B. P., Han J. G., et al. The structure healthy condition monitoring and fault diagnosis methods in wind turbines: a review. Renewable and Sustainable Energy Reviews, Vol. 44, 2015, p. 466-472.
-
Márquez F. P. G., Tobias A. M., Pérez J. M. P., et al. Condition monitoring of wind turbines: Techniques and methods. Renewable Energy, Vol. 46, Issue 5, 2012, p. 169-178.
-
Milborrow D. Operation and maintenance costs compared and revealed. Wind Stats, Vol. 19, Issue 3, 2006, p. 3.
-
Faulstich S., Hahn B., Tavner P. J. Wind turbine downtime and its importance for offshore deployment. Wind Energy, Vol. 14, Issue 3, 2011, p. 327-337.
-
Spinato F., Tavner P. J., Van Bussel G., Koutoulakos E. Reliability of wind turbine subassemblies, IET Renewable Power Generation, Vol. 3, Issue 4, 2009, p. 387-401.
-
Sheng S. Gearbox Typical Failure Modes Detection and Mitigation Methods. National Renewable Energy Laboratory, Golden, USA, 2014.
-
Jin X. H. Z., Cheng F., Peng Y. Y., Qiao W., Qu L. Y. A comparative study on vibration- and current-based approaches for drivetrain gearbox fault diagnosis. IEEE Industry Applications Society Annual Meeting, 2016.
-
Sanchez P., Mendizabal D., Gonzalez K., Zamarreño C. R., Hernaez M., Matias I. R., Arregui F. J. Wind turbines lubricant gearbox degradation detection by means of a lossy mode resonance based optical fiber refractometer. Microsystem Technologies, Vol. 22, 2016, p. 1619-1625.
-
Hossain M. M., Ali M. H. Future research directions for the wind turbine generator system. Renewable and Sustainable Energy Reviews, Vol. 49, 2015, p. 481-489.
-
Qiao W., Lu D. A survey on wind turbine condition monitoring and fault diagnosis – part I: components and subsystems. IEEE Transactions on Industrial Electronics, Vol. 62, Issue 10, 2015, p. 6536-6545.
-
Wind Turbine Gearbox and Direct-Drive Systems, 2014 Update – Global Market Size, Gearbox Refurbishments, Competitive Landscape and Key Country Analysis to 2020. Dublin, Ireland, Tech. Rep. ID: 2974385, 2014.
-
Igba J., Alemzadeh K., Durugbo C., et al. Performance assessment of wind turbine gearboxes using in-service data: Current approaches and future trends. Renewable and Sustainable Energy Reviews, Vol. 50, Issue 6, 2015, p. 144-159.
-
Yoon J., He D., Hecke B. V. On the use of a single piezoelectric strain sensor for wind turbine planetary gearbox fault diagnosis. IEEE Transactions on Industrial Electronics, Vol. 62, Issue 10, 2015, p. 6585-6593.
-
Blesa J., Jiménez P., Rotondo D., et al. An interval NLPV parity equations approach for fault detection and isolation of a wind farm. IEEE Transactions on Industrial Electronics, Vol. 62, Issue 6, 2015, p. 3794-3805.
-
Hall J. F., Chen D. Performance of a 100kW wind turbine with a variable ratio gearbox. Renewable Energy, Vol. 44, Issue 4, 2012, p. 261-266.
-
Fernandes C. M. C. G., Blazquez L., Sanesteban J., et al. Energy efficiency tests in a full scale wind turbine gearbox. Tribology International, Vol. 101, 2016, p. 375-382.
-
He G., Ding K., Li W., et al. A novel order tracking method for wind turbine planetary gearbox vibration analysis based on discrete spectrum correction technique. Renewable Energy, Vol. 87, 2016, p. 364-375.
-
Feng Y., Qiu Y., Crabtree C. J., et al. Monitoring wind turbine gearboxes. Wind Energy, Vol. 16, Issue 5, 2013, p. 728-740.
-
Cibulka J., Ebbesen M. K., Hovland G., et al. A review on approaches for condition based maintenance in applications with induction machines located offshore. Modeling Identification and Control, Vol. 33, Issue 2, 2012, p. 69-86.
-
Ma Yang, He Chengbing, Feng Xinxin Institutions function and failure statistic and analysis of wind turbine. Physics Procedia, Vol. 24, 2012, p. 25-30.
-
Igarashi T., Hamada H. Studies on the vibration and sound of defective roller bearings (First report: vibration of ball bearing with one defect). Bulletin of JSME, Vol. 25, Issue 204, 1982, p. 994-1001.
-
Feng Zhipeng, Zhu Wenying, Zhang Dong Time-frequency demodulation analysis via Vold-Kalman filter for wind turbine planetary gearbox fault diagnosis under nonstationary speeds. Mechanical Systems and Signal Processing, Vol. 128, 2019, p. 93-109.
-
Attya A. B., Hartkopf T. Penetration impact of wind farms equipped with frequency variations ride through algorithm on power system frequency response. Electrical Power and Energy Systems, Vol. 40, 2012, p. 94-103.
-
Horenbeek A. V., Ostaeyen J. V., Duflou J. R., et al. Quantifying the added value of an imperfectly performing condition monitoring system-Application to a wind turbine gearbox. Reliability Engineering and System Safety, Vol. 111, Issue 2, 2013, p. 45-57.
-
Cheng G., Chen X., Li H., et al. Study on planetary gear fault diagnosis based on entropy feature fusion of ensemble empirical mode decomposition. Measurement, Vol. 91, 2016, p. 140-154.
-
Chaari F., Fakhfakh T., Haddar M. Dynamic analysis of a planetary gear failure caused by tooth pitting and cracking. Journal of Failure Analysis and Prevention, Vol. 6, Issue 2, 2006, p. 73-78.
-
Liu W. Y. A review on wind turbine noise mechanism and de-noising techniques. Renewable Energy, Vol. 108, 2017, p. 311-320.
-
Liu Wenyi Intelligent fault diagnosis of wind turbines using multi-dimensional kernel domain spectrum technique. Measurement, Vol. 133, 2019, p. 303-309.
-
Feng Z., Qin S., Liang M. Time-frequency analysis based on Vold-Kalman filter and higher order energy separation for fault diagnosis of wind turbine planetary gearbox under nonstationary conditions. Renewable Energy, Vol. 85, 2016, p. 45-56.
-
Feng Z., Liang M. Complex signal analysis for wind turbine planetary gearbox fault diagnosis via iterative atomic decomposition thresholding. Journal of Sound and Vibration, Vol. 333, Issue 20, 2014, p. 5196-5211.
-
Feng Z., Chen X., Liang M. Iterative generalized synchrosqueezing transform for fault diagnosis of wind turbine planetary gearbox under nonstationary conditions. Mechanical Systems and Signal Processing, Vols. 52-53, Issues 1, 2015, p. 360-375.
-
Li Z., Yan X., Wang X., et al. Detection of gear cracks in a complex gearbox of wind turbines using supervised bounded component analysis of vibration signals collected from multi-channel sensors. Journal of Sound and Vibration, Vol. 371, 2016, p. 406-433.
-
Wang T., Chu F., Han Q. Fault diagnosis for wind turbine planetary ring gear via a meshing resonance based filtering algorithm. ISA Transactions, Vol. 67, 2017, p. 173-182.
-
Kong Y., Wang T., Li Z., et al. Fault feature extraction of planet gear in wind turbine gearbox based on spectral kurtosis and time wavelet energy spectrum. Frontiers of Mechanical Engineering, Vol. 12, 2017, p. 406-419.
-
Wang J., Gao R. X., Yan R. Integration of EEMD and ICA for wind turbine gearbox diagnosis. Wind Energy, Vol. 17, Issue 5, 2014, p. 757-773.
-
Teng W., Ding X., Zhang X., et al. Multi-fault detection and failure analysis of wind turbine gearbox using complex wavelet transform. Renewable Energy, Vol. 93, 2016, p. 591-598.
-
Du Z., Chen X., Zhang H., et al. Sparse Feature Identification Based on Union of Redundant Dictionary for Wind Turbine Gearbox Fault Diagnosis. IEEE Transactions on Industrial Electronics, Vol. 62, Issue 10, 2015, p. 6594-6605.
-
Yang Q., Yang Q., Huang M., et al. Particle swarm optimization-based empirical mode decomposition–kernel independent component analysis joint approach for diagnosing wind turbine gearbox with multiple faults. Transactions of the Institute of Measurement and Control, Vol. 40, Issue 6, 2017, p. 1836-1845.
-
Hu A., Xiang L., Gao N. Fault diagnosis for the gearbox of wind turbine combining ensemble intrinsic time-scale decomposition with Wigner bi-spectrum entropy. Journal of Vibroengineering, Vol. 19, Issue 3, 2017, p. 1759-1770.
-
Hong L., Dhupia J. S., Sheng S. An explanation of frequency features enabling detection of faults in equally spaced planetary gearbox. Mechanism and Machine Theory, Vol. 73, Issue 2, 2014, p. 169-183.
-
Ma Z., Teng W., Liu Y., et al. Application of the multi-scale enveloping spectrogram to detect weak faults in a wind turbine gearbox. IET Renewable Power Generation, Vol. 11, Issue 5, 2017, p. 578-584.
-
Hocine L., Nora Z., Samira K. M. Wind turbine gearbox fault diagnosis based on symmetrical components and frequency domain. Electrical Engineering, Vol. 97, Issue 4, 2015, p. 327-336.
-
Qiu Yingning, Feng Yanhui, Infifield David Fault diagnosis of wind turbine with SCADA alarms based multidimensional information processing method. Renewable Energy, Vol. 145, 2020, p. 1923-1931.
-
Hou Bingchang, Wang Yi, Tang Baoping, Qin Yi, Chen Yang, Chen Yuhang A tacholess order tracking method for wind turbine planetary gearbox fault detection. Measurement, Vol. 138, 2019, p. 266-277.
-
Teng Wei, Ding Xian, Cheng Hao, Han Chen, Liu Yibing, Mu Haihua Compound faults diagnosis and analysis for a wind turbine gearbox via a novel vibration model and empirical wavelet transform. Renewable Energy, Vol. 136, 2019, p. 393-402.
-
Teng Wei, Liu Yiming, Huang Yike, Song Lei, Liu Yibing, Ma Zhiyong Fault detection of planetary subassemblies in a wind turbine gearbox using TQWT based sparse representation. Journal of Sound and Vibration, Vol. 490, 2021, p. 115707.
-
Schmidt Stephan, Mauricio Alexandre, Heyns Stephan P., Gryllias Konstantinos C. A methodology for identifying information rich frequency bands for diagnostics of mechanical components-of-interest under time-varying operating conditions. Mechanical Systems and Signal Processing, Vol. 142, 2020, p. 106739.
-
Yang W., Tavner P. J., Crabtree C. J., et al. Wind turbine condition monitoring: technical and commercial challenges. Wind Energy, Vol. 17, Issue 5, 2014, p. 673-693.
-
Antoniadou I., Manson G., Staszewski W. J., et al. A time-frequency analysis approach for condition monitoring of a wind turbine gearbox under varying load conditions. Mechanical Systems and Signal Processing, 2015, p. 188-216.
-
Igba J., Alemzadeh K., Durugbo C., et al. Analysing RMS and peak values of vibration signals for condition monitoring of wind turbine gearboxes. Renewable Energy, Vol. 91, Issue 3, 2016, p. 90-106.
-
Salem A., Abu Siada A., Islam S. Application of order analysis to diagnose fatigue within wind turbine gearbox. Technology and Economics of Smart Grids and Sustainable Energy, Vol. 2, Issue 1, 2017, p. 3.
-
Ha J. M., Youn B. D., Oh H., et al. Autocorrelation-based time synchronous averaging for condition monitoring of planetary gearboxes in wind turbines. Mechanical Systems and Signal Processing, Vols. 70-71, 2016, p. 161-175.
-
Luo H., Hatch C., Kalb M., et al. Effective and accurate approaches for wind turbine gearbox condition monitoring. Wind Energy, Vol. 17, Issue 5, 2014, p. 715-728.
-
Feng Y. X., Ping Y., Ren J. S., et al. Investigation on vibration based CM technique for wind turbine gearbox. Power and Energy Engineering Conference, 2014.
-
Sheng S. Monitoring of wind turbine gearbox condition through oil and wear debris analysis: a full-scale testing perspective. Tribology Transactions, Vol. 59, Issue 1, 2016, p. 149-162.
-
Qian P., Ma X., Cross P. An integrated data-driven model-based approach to condition monitoring of the wind turbine gearbox. IET Renewable Power Generation, Vol. 11, Issue 9, 2017, p. 1177.
-
Salem A., Abu Siada A., Islam S. Improved condition monitoring technique for wind turbine gearbox and shaft stress detection. IET Science, Measurement and Technology, Vol. 11, Issues 4-7, 2017, p. 431-437.
-
Qian Peng, Zhang Dahai, Tian Xiange, Si Yulin, Li Liangbi A novel wind turbine condition monitoring method based on cloud computing. Renewable Energy, Vol. 135, 2019, p. 390-398.
-
Ren He, Liu Wenyi, Shan Mengchen, Wang Xin A new wind turbine health condition monitoring method based on VMD-MPE and feature-based transfer learning. Measurement, Vol. 148, 2019, p. 106906.
-
Nie Xiaoyin, Liu Shaoguang, Xie Gang A novel autoencoder with dynamic feature enhanced factor for fault diagnosis of wind turbine. Electronics, Vol. 9, 2020, p. 600.
-
Bangalore P., Letzgus S., Karlsson D., et al. An artificial neural network-based condition monitoring method for wind turbines, with application to the monitoring of the gearbox. Wind Energy, Vol. 20, Issue 8, 2017, p. 1421-1438.
-
Al-Tubi I., Long H., Tavner P., et al. Probabilistic analysis of gear flank micro-pitting risk in wind turbine gearbox using supervisory control and data acquisition data. Renewable Power Generation IET, Vol. 9, Issue 6, 2015, p. 610-617.
-
Wang L., Zhang Z., Long H., et al. Wind turbine gearbox failure identification with deep neural networks. IEEE Transactions on Industrial Informatics, Vol. 13, Issue 3, 2017, p. 1360-1368.
-
Simani Silvio, Turhan Cihan Fault diagnosis of wind turbine simulated model via neural networks. IFAC PapersOnLine, Vol. 51, Issue 24, 2018, p. 381-388.
-
Tang Mingzhu, Zhao Qi, Ding Steven X., Wu Huawei, Li Linlin, Long Wen, Huang Bin An improved light GBM algorithm for online fault detection of wind turbine gearboxes. Energies, Vol. 13, 2020, p. 807.
-
Koukoura Sofifia, Carroll James, Mcdonald Alasdair On the use of AI based vibration condition monitoring of wind turbine gearboxes. Journal of Physics: Conference Series, Wind Europe Conference and Exhibition, Bilbao, Spain, Vol. 1222, 2019.
-
Yin Aijun, Yan Yinghua, Zhang Zhiyu, Li Chuan, Sánchez René Vinicio Fault diagnosis of wind turbine gearbox based on the optimized LSTM neural network with cosine loss. Sensors, Vol. 20, 2020, p. 2339.
-
Chang Yuanhong, Chen Jinglong, Qu Cheng, Pan Tongyang Intelligent fault diagnosis of wind turbines via a deep learning network using parallel convolution layers with multi-scale kernels. Renewable Energy, Vol. 153, 2020, p. 205-213.
-
Guo Jianwen, Wu Jiapeng, Zhang Shaohui, Long Jianyu, Chen Weidong, Cabrera Diego, Li Chuan Generative transfer learning for intelligent fault diagnosis of the wind turbine gearbox. Sensors, Vol. 20, 2020, p. 1361.
-
Wenfeng Hu, Hong Chang, Xingsheng Gu A novel fault diagnosis technique for wind turbine gearbox. Applied Soft Computing Journal, Vol. 82, 2019, p. 105556.
-
Qub Fuming, Liua Jinhai, Zhub Hongfei, Zhoub Bowen Wind turbine fault detection based on expanded linguistic terms and rules using non-singleton fuzzy logic. Applied Energy, Vol. 262, 2020, p. 114469.
-
Miao Yonghao, Zhao Ming, Liang Kaixuan, Lin Jing Application of an improved MCKDA for fault detection of wind turbine gear based on encoder signal. Renewable Energy, Vol. 151, 2020, p. 192-203.
-
Li Yanting, Liu Shujun, Shu Lianjie Wind turbine fault diagnosis based on Gaussian process classifiers applied to operational data. Renewable Energy, Vol. 134, 2019, p. 357-366.
-
Azzeddine Bakdia, Abdelmalek Kouadrib, Saad Mekhilef A data-driven algorithm for online detection of component and system faults in modern wind turbines at different operating zones. Renewable and Sustainable Energy Reviews, Vol. 103, 2019, p. 546-555.
-
Wu Zhe, Zhang Qiang, Cheng Lifeng, Tan Shengyue A new method of two-stage planetary gearbox fault detection based on multi-sensor information fusion. Applied Sciences, Vol. 9, 2019, p. 5443.
-
Chacon J. L. F., Andicoberry E. A., Kappatos V., et al. An experimental study on the applicability of acoustic emission for wind turbine gearbox health diagnosis. Journal of Low Frequency Noise Vibration and Active Control, Vol. 35, Issue 1, 2016, p. 64-76.
-
Cheng F., Peng Y., Qu L., et al. Current-based fault detection and identification for wind turbine drivetrain gearboxes. IEEE Transactions on Industry Applications, Vol. 53, Issue 2, 2017, p. 878-887.
-
Lu D., Qiao W., Gong X. Current-based gear fault detection for wind turbine gearboxes. IEEE Transactions on Sustainable Energy, Vol. 8, Issue 4, 2017, p. 1453-1462.
-
Grujicic M., Galgalikar R., Ramaswami S., et al. Finite-element analysis of horizontal-axis wind-turbine gearbox failure via tooth-bending fatigue. Science and Engineering Publishing, Vol. 3, Issue 1, 2014, p. 6-15.
-
Gao B., He Y., Woo W. L., et al. Multi-dimensional tensor-based inductive thermography with multiple physical fields for offshore wind turbine gear inspection. IEEE Transactions on Industrial Electronics, Vol. 63, Issue 10, 2016, p. 6305-6315.
-
Yoon J., He D., Van Hecke B., et al. Vibration-based wind turbine planetary gearbox fault diagnosis using spectral averaging. Wind Energy, Vol. 19, Issue 9, 2016, p. 1733-1747.
-
Sheldon J., Mott G., Lee H., et al. Robust wind turbine gearbox fault detection. Wind Energy, Vol. 17, Issue 5, 2014, p. 745-755.
-
Sinha Y., Steel J. A., Andrawus J. A., et al. Significance of effective lubrication in mitigating system failures - a wind turbine gearbox case study. Wind Engineering, Vol. 38, Issue 4, 2015, p. 441-450.
-
Vanhollebeke F., Peeters J., Vandepitte D., et al. Using transfer path analysis to assess the influence of bearings on structural vibrations of a wind turbine gearbox. Wind Energy, Vol. 18, Issue 5, 2015, p. 797-810.
-
Xiang D., Jiang L., You M., et al. Influence of quasi-steady wind loads on the fatigue damage of wind turbine gearboxes. Strojniški vestnik-Journal of Mechanical Engineering, Vol. 63, Issue 5, 2017, p. 300-313.
-
Zhang Y., Lu W., Chu F. Planet gear fault localization for wind turbine gearbox using acoustic emission signals. Renewable Energy, Vol. 109, 2017, p. 449-460.
-
Jiang G., He H., Xie P., et al. Stacked multilevel-denoising autoencoders: a new representation learning approach for wind turbine gearbox fault diagnosis. IEEE Transactions on Instrumentation and Measurement, Vol. 66, Issue 9, 2017, p. 2391-2402.
-
Huang W., Sun H., Dou H., et al. Study of transfer path of wind turbine gearbox fault vibration signal based on power flow finite element method. Advances in Mechanical Engineering, Vol. 9, Issue 5, 2017, p. 16-20.
-
Helsen J., Guo Y., Keller J., et al. Experimental investigation of bearing slip in a wind turbine gearbox during a transient grid loss event. Wind Energy, Vol. 19, Issue 12, 2016, p. 2255-2269.
-
He G., Ding K., Li W., et al. Frequency response model and mechanism for wind turbine planetary gear train vibration analysis. IET Renewable Power Generation, Vol. 11, Issue 4, 2017, p. 425-432.
-
Gao Q. W., Liu W. Y., Tang B. P., Li G. J. A novel wind turbine fault diagnosis method based on integral extension load mean decomposition multiscale entropy and least squares support vector machine. Renewable Energy, Vol. 116, 2018, p. 169-175.
-
Vamsi Inturi, Sabareesh G. R., Vaibhav Sharma Integrated vibro-acoustic analysis and empirical mode decomposition for fault diagnosis of gears in a wind turbine. Procedia Structural Integrity, Vol. 14, 2019, p. 937-944.
-
Zhang Sikai, Lang Zi-Qiang SCADA-data-based wind turbine fault detection: a dynamic model sensor method. Control Engineering Practice, Vol. 102, 2020, p. 104546.
-
Wang Zhijian, Wang Junyuan, Kou Yanfei, Zhang Jiping, Ning Shaohui, Zhao Zhifang Weak fault diagnosis of wind turbine gearboxes based on MED-D. Entropy, Vol. 19, 2017, p. 277.
-
Zhang Le, Yang Qiang Investigation of the design and fault prediction method for an abrasive particle sensor used in wind turbine, gearbox. Energies, Vol. 13, 2020, p. 365.
-
Chen Huanguo, Chen Pei, Chen Wenhua, Wu Chuanyu, Li Jianmin, Wu Jianwei Wind turbine gearbox fault diagnosis based on improved EEMD and Hilbert Square demodulation. Applied Sciences, Vol. 7, 2017, p. 128.
-
Su Hongsheng, Wang Dantong, Duan Xuping Condition maintenance decision of wind turbine gearbox based on stochastic differential equation. Energies, Vol. 13, 2020, p. 4480.
-
Sun Chuang, Chen Xuefeng Composite graph-based sparse subspace clustering for machine fault diagnosis. IEEE Transactions on Instrumentation and Measurement, Vol. 69, Issue 5, 2020, p. 1850-1859.
-
Fong Stanley, Harmouche Jinane, Narasimhan Sriram, Antoni Jerome Mean shift clustering-based analysis of non-stationary vibration signals for machinery diagnostics. IEEE Transactions on Instrumentation and Measurement, Vol. 69, Issue 7, 2020, p. 4056-4066.
-
Tayarani-Bathaie S.-S., Khorasani K. Fault detection and isolation of gas turbine engines using a bank of neural networks. Journal of Process Control, Vol. 36, Issue 1, 2015, p. 22-41.
-
Nor N. M., Steeneveld W., Mourits M. C. M., et al. Study on Fault Diagnosis Expert System for the Engine of a Helicopter Based on Rule. Journal of Dairy Science, Vol. 98, Issue 2, 2015, p. 861-871.
-
Li Z., Outbib R., Giurgea S., et al. Online implementation of SVM based fault diagnosis strategy for PEMFC systems. Applied Energy, Vol. 164, Issue 2, 2016, p. 284-293.
Cited by
About this article
This research was supported by the National Natural Science Foundation of China (Grant No. 51505202), the Natural Science Foundation of Jiangsu Province of China (No. BK20201463), the 333 Project of Jiangsu Province of China (2016-III-2808), the Qing-Lan Project of Jiangsu Province of China (QL2016013). Dr. Wenyi Liu is the corresponding author.