Abstract
In the paper a multichannel vibration data processing method is presented in the context of local damage detection in gearboxes. The purpose of the approach is to obtain more reliable information about local damage when using several channels in comparison to results obtained for single channel vibration. The method is a combination of time-frequency representation and Independent Component Analysis (ICA) but applied not to raw time series but to each slice (along to time) from spectrogram. Finally we create new time-frequency map, that after aggregation clearly indicates presence of damage. In the paper we will present details of the method and benefits of using our procedure. We will refer to autocorrelation function of mentioned aggregated new time frequency map (1D signal) or simple spectrum (that might be somehow linked to classical envelope analysis). We believe that results are very convincing – detection of cyclic impulses associated to local damage are clearly identifiable. To validate our method we use real vibration data from heavy duty gearbox used in mining industry.
1. Introduction
A problem of fault diagnosis in rotating machines attracts attention of researchers for many years. In the literature one can find several comprehensive reviews on damage detection in gears and bearings [1-4]. Classical methods incorporate high-order statistics [5], empirical mode decomposition [6], wavelet transform [7], time-frequency domain analysis [8-10], bi-frequency analysis [1, 11]. Vibration signal from a machinery system is often a mixture of several source signals. For instance, a signal acquired on a bearing operating in a belt conveyor driving station might be contaminated with vibrations of a gearbox located nearby [12-21], vibration signal from a hummer crusher bearing consist of both a signal related to bearing and an impulsive noise related to the crushing process [22]. Another example of a multi-source signal is a gearbox with two faults, each of different nature [17, 23]. In [24] authors analyze a signal from a gas compressor and provide a diagnostic method in case of bearing vibration signal in presence of impulsive noise. In this paper we incorporate independent component analysis (ICA) for local damage detection in a two-stage gearbox operating in a belt conveyor driving station. The vibration data obtained during the experiment represents vibration acceleration of a single gearbox, measured at 3 different locations. ICA is performed on time-frequency representations of the signals. The method proved that integration of vibration signals from several channels provide a more clear damage indication than a single signal does.
The paper is structured as follows. In section 2 a methodology based on both ICA and time-frequency representation is described. Section 3 contains description of the considered machine and results obtained from real data analysis. The last section contains conclusions.
2. Methodology
In this section we present the methodology which we use to real vibration signal from heavy duty gearbox of belt conveyor used in mining industry. The technique consists of few main steps. As it was mentioned, we analyze the multichannel (three dimensional) vibration data. First, the decomposition of input channels into time-frequency maps (spectrograms) is performed. The spectrogram is an absolute value of the short-time Fourier transform (STFT) defined as follows:
where is the shifted window and is the input signal. In the next step of our procedure we divide the time-frequency maps into narrow-band slices corresponding to given frequencies. As a result of this step we obtain three dimensional sub-signals for each frequency band. Then for each of three-dimensional sub-signal we apply the Independent Component Analysis (ICA) which returns three independent features. In signal processing, the ICA is a computational method for separating a multivariate signal into additive subcomponents (features). This is done by assuming that the subcomponents are non-Gaussian signals and that they are statistically independent from each other. ICA is a special case of blind source separation [25-33].
Fig. 1The scheme of the introduced technique of local damage detection
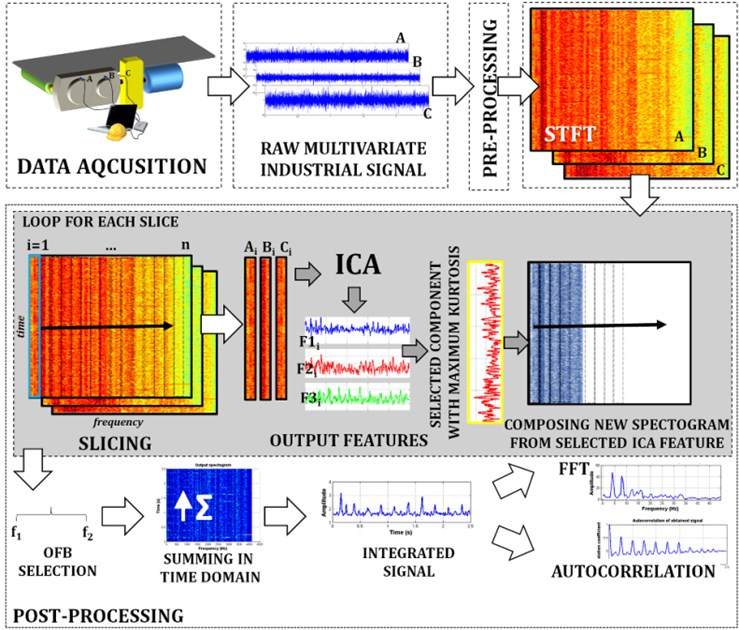
In the next step of our analysis for each three-dimensional sub-signal we select one feature, mainly this with the greatest kurtosis. As a result we obtain the new time-frequency map which contains selected features corresponding to given frequencies. On the basis of this map we can indicate the informative frequency band. At the end we aggregate the new time-frequency map in time domain to extract one-dimensional time series which may contain cyclic components resulting from damage. In order to enhance this cyclic behavior we calculate spectrum of the new time series and/or its autocorrelation function.
The scheme of the presented methodology is shown in Fig. 1.
3. Application to industrial data
3.1. Machine and experiment description
The machine considered here is a two-stage gearbox used in drive system for belt conveyor, see Fig. 2(a). Data used for validation of the method has been measured with using 3 accelerometers mounted on the gearbox housing (Fig. 2(b)) and the Brüel&Kjær Pulse system. Due to high level of noise and presence of high amplitude components related to mesh frequencies informative part of the signal representing damage cannot be seen in the acquired signals. It is known that impulsive signal related to damage in gear-wheel on the middle shaft should be associated to the fault frequency equals to 4.1 Hz.
Fig. 2a) Scheme of the investigated machine, b) location of sensors on gearbox housing
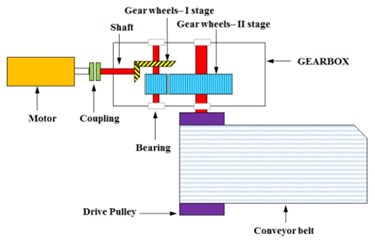
a)
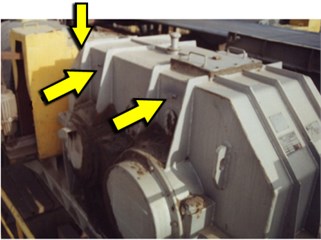
b)
3.2. Diagnostic data – raw multichannel signal
Raw multivariate (3D) signal considered here consists of three 2.5 s time-series representing gearbox vibration under “normal” operation i.e. during transportation of bulk material. Frequency sampling is 8192 Hz. Raw multichannel signal and its spectrogram representation is shown in Figs. 3 and 4, respectively. Both time series and their spectrograms allow to notice some weak impulsive/wideband components but it is impossible to distinguished unambiguously.
Fig. 3Multichannel input signal
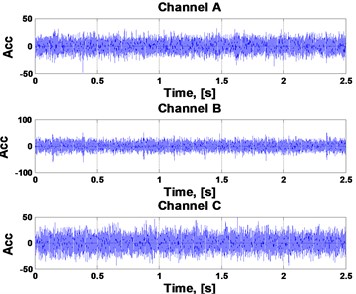
Fig. 4Spectrograms of the multichannel signal
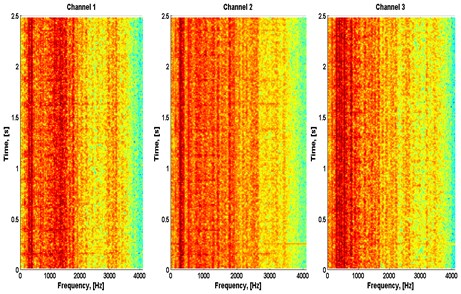
3.3. Evaluation of algorithm performance with industrial data
In this section we present the obtained results i.e. performance of the algorithm provided with real industrial data. Fig. 5(a) presents output, i.e. new time-frequency map which consists of the features extracted by applying ICA to the sub-signals from the initial spectrograms presented in Fig. 4. As we observe, the impulses (wideband excitations) are visible much clearly than in the spectrograms of multichannel raw signal. Moreover, simple aggregation of 2D map into 1D vector by integration of energy for each time instance shows impulsive nature of energy flow. Using autocorrelation or simple spectrum one might identify period/frequency of impulse repetition that corresponds to fault frequency, see Fig. 5(b).
Fig. 5a) Output spectrogram composed from selected ICA features, b) time series extracted from output spectrogram, spectrum of integrated time series and autocorrelation function of the integrated time series
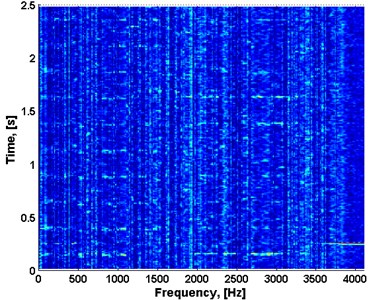
a)
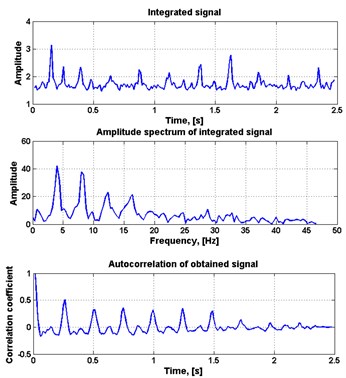
b)
4. Conclusions
In this paper we have introduced a new method of local damage detection applied to the real vibration signal from heavy duty gearbox used in mining industry. This methodology is based on the analysis of multichannel time series and its representation in time-frequency domain (spectrogram). In order to extract information about the damage we analyze the features obtained by applying the ICA to three-dimensional sub-signals corresponding to given frequencies on the spectrogram. The introduced technique applied to real signal gives much better results than the classical methods based on the analysis of one-dimensional vibration signal. By using the methodology we can easier identify the informative frequency band. Moreover, the cyclic impulses are more visible and distinguishable. We should mention, the proposed algorithm is automatic and can be applied to other vibration signals for which the classical methods do not give the desired results.
References
-
Randall R. B., Antoni J. Rolling element bearing diagnostics – a tutorial. Mechanical Systems and Signal Processing, Vol. 25, Issue 2, 2011, p. 485-520.
-
Samuel P. D., Pines D. J. A review of vibration-based techniques for helicopter transmission diagnostics. Journal of Sound and Vibration, Vol. 282, Issues 1-2, 2005, p. 475-508.
-
Yaguo L., Jing L., Zhengjia H., Ming J., Zuo A. Review on empirical mode decomposition in fault diagnosis of rotating machinery. Mechanical Systems and Signal Processing, Vol. 35, Issues 1-2, 2013, p. 108-126.
-
Feng Z., Liang M., Chu F. Recent advances in time-frequency analysis methods for machinery fault diagnosis: a review with application examples. Mechanical Systems and Signal Processing, Vol. 38, Issue 1, 2013, p. 165-205.
-
Antoni J., Randall R. The spectral kurtosis: application to the vibratory surveillance and diagnostics of rotating machines. Mechanical Systems and Signal Processing, Vol. 20, Issue 2, 2006, p. 308-331.
-
Dybała J., Zimroz R. Rolling bearing diagnosing method based on Empirical Mode Decomposition of machine vibration signal. Applied Acoustics, Vol. 77, 2014, p. 195-203.
-
Lin J., Zuo M. Gearbox fault diagnosis using adaptive wavelet filter. Mechanical Systems and Signal Processing, Vol. 17, Issue 6, 2003, p. 1259-1269.
-
Burdzik R., Konieczny L., Folęga P. Structural health monitoring of rotating machines in manufacturing processes by vibration methods. Advanced Materials Research, Vol. 1036, 2014, p. 642-647.
-
Lazarz B., Wojnar G., Czech P. Early fault detection of toothed gear in exploitation conditions. Eksploatacja i Niezawodnosc – Maintenance and Reliability, Vol. 49, Issue 1, 2011, p. 68-77.
-
Combet F., Gelman L. Optimal filtering of gear signals for early damage detection based on the spectral kurtosis. Mechanical Systems and Signal Processing, Vol. 23, Issue 3, 2009, p. 652-668.
-
Borghesani P., Pennacchi P., Chatterton S. The relationship between kurtosis- and envelope-based indexes for the diagnostic of rolling element bearings. Mechanical Systems and Signal Processing, Vol. 43, Issues 1-2, 2014, p. 25-43.
-
Obuchowski J., Wylomanska A., Zimroz R. Stochastic modeling of time series with application to local damage detection in rotating machinery. Key Engineering Materials, Vols. 569-570, 2013, p. 441-448.
-
Zak G., Obuchowski J., Wylomańska A., Zimroz R. Application of ARMA modelling and alpha-stable distribution for local damage detection in bearings. Diagnostyka, Vol. 15, Issue 3, 2014, p. 3-10.
-
Obuchowski J., Wylomańska A., Zimroz R. Recent developments in vibration based diagnostics of gear and bearings used in belt conveyors. Applied Mechanics and Materials, Vol. 683, 2014, p. 171-176.
-
Obuchowski J., Wyłomańska A., Zimroz R. The local maxima method for enhancement of time-frequency map and its application to local damage detection in rotating machines. Mechanical Systems and Signal Processing, Vol. 46, Issue 2, 2014, p. 389-405.
-
Obuchowski J., Wyłomańska A., Zimroz R. Selection of informative frequency band in local damage detection in rotating machinery. Mechanical Systems and Signal Processing, Vol. 48, Issues 1-2, 2014, p. 138-152.
-
Obuchowski J., Wylomanska A., Zimroz R. Two-stage data driven filtering for local damage detection in presence of time varying signal to noise ratio. Mechanisms and Machine Science, Vol. 23, 2015, p. 401-410.
-
Obuchowski J., Zimroz R., Wylomanska A. Identification of cyclic components in presence of non-Gaussian noise application to crusher bearings damage detection. Journal of Vibroengineering, Vol. 17, 2015, p. 1242-1252.
-
Zak G., Obuchowski J., Wyłomańska A., Zimroz R. Novel 2D representation of vibration for local damage detection. Mining Science, Vol. 21, 2014, p. 105-113.
-
Zak G., Wylomanska A., Zimroz R. Application of alpha-stable distribution approach for local damage detection in rotating machines. Journal of Vibroengineering, Vol. 16, Issue 6, 2015, p. 2987-3002.
-
Barszcz T., Jabłoński A. A novel method for the optimal band selection for vibration signal demodulation and comparison with the Kurtogram. Mechanical Systems and Signal Processing, Vol. 25, Issue 1, 2011, p. 431-451.
-
Obuchowski J., Zimroz R., Wylomanska A. Identification of cyclic components in presence of non-Gaussian noise application to crusher bearings damage detection. Journal of Vibroengineering, Vol. 17, 2015, p. 1242-1252.
-
Burdzik R. Research on the influence of engine rotational speed to the vibration penetration into the driver via feet – multidimensional analysis. Journal of Vibroengineering, Vol. 15, Issue 4, 2013, p. 2114-2123.
-
Barszcz T., Zimroz R., Urbanek J., Jabłoński A., Bartelmus W. Bearings fault detection in gas compressor in presence of high level of non-Gaussian impulsive noise. Key Engineering Materials, Vol. 569, Issue 570, 2013, p. 473-480.
-
Hyvarnen A., Oja E. A fast fixed-point algorithm for independent component analysis. Neural Computation, Vol. 9, 1997, p. 1483-1492.
-
Hyvarnen A., Oja E. Independent component analysis: algorithms and applications. Neural Networks, Vol. 13, 2000, p. 411-430.
-
Li L., Qu L. Machine diagnosis with independent component analysis and envelope analysis. IEEE ICIT, 2002, p. 1360-1364.
-
He Q., Feng Z., Kong F. Detection of signal transients using independent component analysis and its application in gearbox condition monitoring. Mechanical Systems and Signal Processing, Vol. 21, Issue 5, 2007, p. 2056-2071.
-
Zuo M. J., Lin J., Fan X. Feature separation using ICA for a one-dimensional time series and its application in fault detection. Journal of Sound and Vibration, Vol. 287, Issue 3, 2005, p. 614-624.
-
Roan M. J., Erling J. G., Sibul L. H. A new, non-linear, adaptive, blind source separation approach to gear tooth failure detection and analysis. Mechanical Systems and Signal Processing, Vol. 16, Issue 5, 2002, p. 719-740.
-
Lin J., Zhang A. Fault feature separation using wavelet-ICA filter. NDT and E International, Vol. 38, Issue 6, 2005, p. 421-427.
-
Wang J., Gao R. X., Yan R. Integration of EEMD and ICA for wind turbine gearbox diagnosis. Wind Energy, Vol. 17, Issue 5, 2014, p. 757-773.
-
Wang H., Li R., Tang G., Yuan H., Zhao Q., Cao X. A Compound fault diagnosis for rolling bearings method based on blind source separation and ensemble empirical mode decomposition. PLoS ONE, Vol. 9, Issue 10, 2014, p. e109166.
About this article
This work is partially supported by the Statutory Grant No. S40128 (R. Zimroz), No. S50112 (G. Zak), No. B50120 (J. Obuchowski), No. B40037 (P. Stefaniak), No. B50137 (P. Stefaniak).