Abstract
Optimizing engineering processes for effective time and cost considerations especially in the field of geotechnical engineering which involves time-consuming laboratory processes and procedures. This study aims at developing predictive models for computing the mechanical property of the soil. In this study, the property of the soil of keen interest was the California Bearing Ratio (CBR) for soaked condition. The CBR of the natural soil was computed and found to be 70.14 %. The optimization technique was carried out effectively using Scheffe’s second-degree polynomials and the optimal mix ratio was observed to be 1:0.75:0.17 for soil, rice husk and water with an optimal Soaked CBR value of 80 % corresponding to the Y3 response space. The model generated for predicting the CBR of the soil treated with rice husk ash is given as; CBR(soaked)=75K1+77K2+80K3-24K1K2-2K1K3-2K2K3. Validation using the F-test method gave the F-stat value as 4.34 with a corresponding F-critical value of 5.05. The result from the F-test was found adequate at a confidence level of 95 %. The validation results further showed that in all, there was no significant difference between the model results and the experimental results. Therefore, the model is adequate and the null hypothesis was adopted.
1. Introduction
In most situations, the soil to be used as a foundation bed for a structure or road pavement is seen to possess very low engineering properties as required to support the intended load from the structure or pavement. The advent of soil stabilization has been a relief for tackling soil related problems. In a quest to substantially provide a reduction in the cost of construction process, other seemingly cheaper and readily available materials for soil stabilization are greatly considered. This study essentially considers an agro-allied waste material such as rice husk ash, for the stabilization of soil. These agro-waste materials are by-products of agricultural processes, which are also a significant source of environmental pollution [1].
Researchers have found that agro-waste materials are “often burned outdoors, dumped in landfills and littered around the environment, leading to environmental pollution of all sort” [2], [3-6]. The soil considered for the study is lateritic soil, which are predominantly existent in tropical and subtropical regions such as Nigeria. Soils of this nature are usually characterized by low strength, high plasticity due to the presence of clay minerals, susceptibility to erosion, moisture variation leading to excessive shrinkage and swelling potentials. Soil stabilization techniques are crucial to enhance the Engineering properties of soil for sustainable infrastructural development. Among these techniques, the use of rice husk ash, RHA as a stabilization agent further integrated with the Scheffe’s method for optimization has gained significant attention in recent research.
Models generated in a study for soil sampled from Enugu, Enugu State, for optimizing the CBR of the soil that has been treated with variations of Rice Husk Ash, were concluded as accurate in predicting the CBR of the soil. The results showed that the optimal mix proportion was 1:1.70:0.25 with a resulting CBR value of 30 %. Further steps were employed which involved subjecting the model to validation using the F-test and T-test statistical method. The model was found adequate at a confidence level of 95 %, which informed the adoption of the null hypothesis (H0) [7], [8].
Furthermore, in a study undertaken in Ebonyi state, the researcher employed the Scheffe’s approach as an optimization method to model the California bearing ratio properties of weak lateritic soil stabilized with constituents of kaolin for pavement construction purposes. The lateritic soil and kaolin used in the research were both sampled from Ebonyi state. The application of the Scheffe second-degree polynomial in deriving the models for predicting the CBR behavior of the kaolin treated laterite soil was carefully carried out. The highest CBR, which had a value of 76.6 %, was recorded for the stabilized soil sample. The adequacy of the model was scrutinized by the student T-test and analysis of variance (ANOVA) test, and the model was found to be satisfactory at a confidence level of 95 % [9], [10].
2. Methodology
2.1. Study area
The study area is situated in the south-eastern part of Nigeria in Agbani town, Enugu State. The soil was collected from the environs of the Enugu State University of Science and Technology (ESUT). The area is characterized by soil with considerable stiffness as observed by the difficulty posed during the excavation of the trial pit.
2.2. Sampling of materials
Trial pit for soil sampling was excavated, reaching a depth of 1.3 m with the use of shovels and pickaxe, the soil obtained were disturbed soil. Corresponding coordinates for the area was obtained using the Google Earth application. The Global positioning system (GPS) coordinate for the location is given as 6.304458° for latitude and 7.541142° for longitude respectively. The rice husk was sourced from a local rice miller located in Ugbawka, Nkanu East Local Government Area, Enugu State. Impurities present in the husks were sorted out; the husks were placed in bags and further transported to the laboratory where it was incinerated into ash.
2.3. Soil characterization method
The results from the laboratory testing of the soil informs its properties and aids its delineation based on those properties. The soil classification systems employed for the classification were the American Association of State Highway and Transport Officials (AASHTO) system and the Unified Soil Classification System. The soil in this study was classified based on these soil classification systems relative to the properties exhibited by the soil as observed from the laboratory test conducted [11-13].
2.4. Methods for testing of soil
The soil testing was carried out in harmony with the methods stipulated in BS 1377 (methods for soil testing). Therefore, to determine the index properties of the soil, the following tests that includes; Sieve analysis, Atterberg Limits, Compaction, Moisture Content, Specific Gravity and California Bearing Ratio were conducted. The standardized laboratory test results for the natural soil are highlighted in this paper. Methods of computation for some of the tests carried out are listed as Eq. (1) and Eq. (2):
where w1 is the weight of dry soil and can, w2 is the weight of wet soil and can, w3 is the weight of can.
where LL is the liquid limit, PL is the plastic limit.
However, the property of the stabilized soil of keen interest in this study was the CBR property of the soil. The CBR test procedures is further discussed below.
2.4.1. California bearing ratio
This test was conducted to evaluate soil strength under soaked conditions. The soil was mixed in three layers and 61 (sixty-one) blows were given to each layer by a rammer as a means of compaction. The soil is placed under a plunger which penetrates the soil. The load readings by the plunger is obtained from the dial gauge at 1.25 mm per minute intervals. The dial gauge reading is multiplied by the proving ring factor of 0.019 KN, which is the applied force by the plunger. Penetration values at 2.5 mm and 5 mm are calculated as follows [13]:
2.5. Scheffe’s method of optimization
Henry Scheffe devised a method to optimize the behavior of stabilized soils, where the property to be improved depends on the percentages or proportions by weight of the constituents, not on their quantities in the combination. The responses known as “q, n-polynomials” are modelled using a second-degree polynomial regression. The method represents the inputs as a simplex, which is a polygon or factor space with a straight line as its basic simplex [14].
Straight lines signifies a one-dimensional factor spaces, solids are factor spaces that are three-dimensional such as; prisms, cones, tetrahedrons, spheres, cylinders, cubes, and cuboids, among other shapes [14].
In addition, based on the number of mix constituents that make up the factor space, the number of responses or observations to be expected is derived using Eq. (5):
Such that, is the number of observations required, is the Number of mixture components, is the Degree of the polynomials.
This research work involved a three-component mixture (Soil, RHA and Water) hence; the second-degree (3, 2) simplex model is a two-dimensional factor space represented as an Equilateral Triangle. Employing Eq. (5), to determine the number of responses in the experiment and control points. Hence:
Therefore, the mix design required six number of responses. In addition, six other responses were obtained for the control points used for validating the model. Furthermore, the response of the mixture is assumed a real value function on a simplex, to which an appropriate form of polynomial regression model is introduced.
The polynomial function of degree in variable has coefficients. If a mixture has a total of components and is the proportion of the th component in the mixture such that 0 ( 1, 2, …, ), then the summation of the component proportion is equal to one, i.e. [7-10].
Further written as:
One mix component appears at a single vertex of the simplex triangle. The simplex triangle’s vertices only contain stand-alone components of a mixture; blend components are limited to existing along the line that joins two vertices; and no more than two components of a mixture are present along a single line that joins two vertices concurrently.
2.5.1. Development of the model using Scheffe’s method
Scheffe’s design mix, which facilitated the formulation of the model, consists of two fundamental elements, which are the Pseudo and Real mix components, designated as and , respectively. Scheffe states that the relationship between and is given as:
where is the real mix ratio matrix, is the coefficient of the matrix, is the pseudo mix ratio matrix
The polynomial equation below is used to determine the system's response, which is represented by
Considering a three component mix of two degrees, the response is of the form:
Generally, the polynomial equation for a ternary system is given as:
Multiplying Eq. (6) by :
Furthermore, multiplying Eq. (6) by , and successively and re-arranging gives:
Substituting Eq. (11) and Eq. (12) into Eq. (10) gives:
Let:
Re-writing Eq. (13) to arrive at a reduced second-degree polynomial with three variables:
Fig. 1Simplex triangle (2-dimensional factor space), where K1 is the Stand-alone of lateritic soil, K2 is the stand-alone of rice husk ash, K3 is the stand-alone of water, K12 is the blend of soil and rice husk ash, K13 is the blend of lateritic soil and water, K23 is the blend of rice husk ash and water
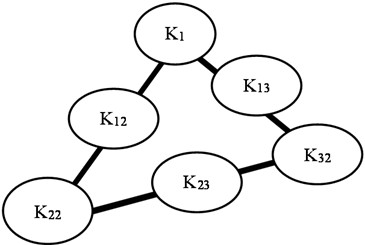
Therefore, Eq. (16) is the model as per Scheffe’s method of simplex lattice design used in this study for predicting the CBR values of the soil at various mix proportions. The coefficient of the second-degree polynomial is further derived from the vertex of the simplex triangle. As shown in Fig. 1, only stand-alone components are present at the vertex of the simplex triangle while intersections have blends of components. Hence, , and have coordinates as follows: ; ; .
2.5.2. Determination of the coefficients of the model
Substituting the above lattice coordinate into Eq. (16) gives the coefficient of the second-degree polynomial as:
In addition:
Therefore, Eq. (17) and Eq. (18) represents the coefficients of the second-degree polynomial.
2.6. Statistical validation technique for the model
The validity of the model was determined using a statistical test method to test the adequacy of the model and also enable for the acceptance of the study hypothesis.
2.6.1. Fisher’s test (F-test)
In this study, the model developed was subjected to the F-test for validation and adequacy check. Value for the F-statistics is then obtained; the F-statistics is given as the ratio of variance between the models (predicted values) and the empirical values, with the greater value of either variance being the numerator. In addition, the models developed was declared adequate and the null hypothesis accepted if the calculated F-statistics is less than the F-critical at a confidence level of 95 %. Conversely, the alternate hypothesis is adopted and the predictive model is considered inadequate. The F-test was ran on the 2016 version of Microsoft Excel application.
3. Results and analysis
Analysis of the laboratory test results as carried out in the laboratory are made explicit and are presented. The index properties of the natural soil are tabulated and are shown in Table 1. Conversely, data for the optimization technique using Scheffe’s method is also presented and analyzed. The matrix formation technique by Scheffe’s second-degree polynomial is shown and the results outlined. Other underlining issues are further discussed.
3.1. Design mix matrix
A matrix is typically used to express the design mix's constituent. Additionally, as shown in Eq. (16), the optimization model is expressed in pseudo mix ratios, and Eq. (7) illustrates the link between the pseudo and real components.
By weighing the dry lateritic soil, the amount of rice husk ash was calculated, and the associated water content was determined. The mix ratios for the vertices where pure blends of the mix are to be present are as follows:
Table 1Properties of the soil
S/N | Properties | Standards | Results |
1 | Colour | – | Reddish-Brown |
2 | Percentage passing sieve No 200 | BS 1377 | 29 |
3 | Liquid limit (%) | BS 1377 | 34.10 |
4 | Plastic limit (%) | BS 1377 | 25 |
5 | Plasticity index | BS 1377 | 9.10 |
6 | Specific gravity | BS 1377 | 2.54 |
7 | Natural moisture content (%) | BS 1377 | 7.73 |
8 | AASHTO classification | AASHTO | A-2-4 |
9 | Unified classification system | USCS | SW |
10 | Maximum dry density (kg/m3) | BS 1377 | 2150 |
11 | Optimum moisture content (%) | BS 1377 | 6 |
12 | CBR (soaked) (%) | BS 1377 | 70.14 |
13 | CBR (un-soaked) (%) | BS 1377 | 85.17 |
The related pseudo mix ratios have the shape of an identity matrix as follows:
Furthermore, Eq. (7) can be expressed as follows:
Substituting values for the matrix into Eq. (19) for the first run, the matrix becomes:
Eq. (20) gives the following coefficients for matrix :
Considering the second run, the matrix is of the form:
Eq. (21) gives the following coefficients for matrix :
For the third run, the matrix is of the form:
Eq. (22) gives the following coefficients for matrix :
The coefficients derived from Eq. (20) to Eq. (22) put together, further yields the following coefficient matrix :
3.1.1. Derivation for real components
The values of the real components () of the mixture are obtained through the multiplication of the values from matrix and values from matrix, .
For , substituting the values of yields:
For , substituting the values of yields:
For , substituting the values of yields:
Table 2Mix proportion for trial mix
Trial | Actual components | Responses | Pseudo components | ||||
Soil | RHA | Water | Soil | RHA | Water | ||
1 | 1 | 0.25 | 0.15 | 1 | 0 | 0 | |
2 | 1 | 0.50 | 0.16 | 0 | 1 | 0 | |
3 | 1 | 0.75 | 0.17 | 0 | 0 | 1 | |
4 | 1 | 0.38 | 0.16 | 0.5 | 0.5 | 0 | |
5 | 1 | 0.50 | 0.16 | 0.5 | 0 | 0.5 | |
6 | 1 | 0.63 | 0.17 | 0 | 0.5 | 0.5 |
3.1.2. Derivation for control points
The control points are represented as , , , , , and .
For control, point :
For control point :
For control point :
For control point :
For control point :
For control point :
Table 3Mix proportion for control points
Trial | Actual components | Responses | Pseudo components | ||||
Soil | RHA | Water | Soil | RHA | Water | ||
1 | 1 | 0.48 | 0.16 | 0.4 | 0.3 | 0.3 | |
2 | 1 | 0.5 | 0.16 | 0.3 | 0.4 | 0.3 | |
3 | 1 | 0.53 | 0.16 | 0.3 | 0.3 | 0.4 | |
4 | 1 | 0.40 | 0.16 | 0.6 | 0.2 | 0.2 | |
5 | 1 | 0.50 | 0.16 | 0.2 | 0.6 | 0.2 | |
6 | 1 | 0.60 | 0.16 | 0.2 | 0.2 | 0.6 |
3.2. Experimental results from the optimization
Sequel to the formulation of the design matrix using the Scheffe’s optimization technique and obtaining values for the mix proportion, the soil mix was subjected to California bearing ratio test for soaked condition, which was conducted on 12 (twelve) soil samples having distinct mix proportions. Six of which are trial mixes and the other six are control point mixes.
The results of the Soaked CBR for the treated soil samples are shown in the Tables 4 and 5, as well as the corresponding CBR plot for all responses are displayed in Fig. 3 and 4.
Table 4Soaked CBR experimental results for trial mix
Actual components | Responses | CBR (%) | Pseudo components | ||||
Soil | RHA | Water | Soil | RHA | Water | ||
1 | 0.25 | 0.15 | 75 | 1 | 0 | 0 | |
1 | 0.50 | 0.16 | 77 | 0 | 1 | 0 | |
1 | 0.75 | 0.17 | 80 | 0 | 0 | 1 | |
1 | 0.38 | 0.16 | 70 | 0.5 | 0.5 | 0 | |
1 | 0.50 | 0.16 | 77 | 0.5 | 0 | 0.5 | |
1 | 0.63 | 0.1.7 | 78 | 0 | 0.5 | 0.5 |
Table 5Soaked CBR experimental results for control point
Actual components | Responses | CBR (%) | Pseudo components | ||||
Soil | RHA | Water | Soil | RHA | Water | ||
1 | 0.48 | 0.14 | 70 | 0.4 | 0.3 | 0.3 | |
1 | 0.5 | 0.16 | 77 | 0.3 | 0.4 | 0.3 | |
1 | 0.53 | 0.16 | 76 | 0.3 | 0.3 | 0.4 | |
1 | 0.40 | 0.16 | 73 | 0.6 | 0.2 | 0.2 | |
1 | 0.50 | 0.16 | 77 | 0.2 | 0.6 | 0.2 | |
1 | 0.60 | 0.16 | 76 | 0.2 | 0.2 | 0.6 |
Fig. 2Graphical description of soaked CBR values
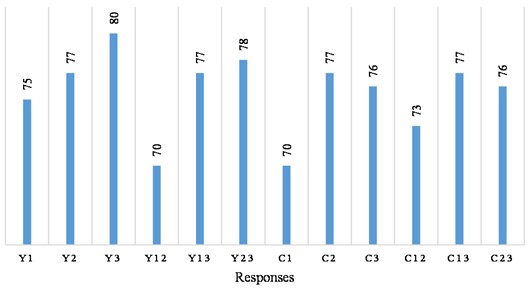
Fig. 3Experimental soaked CBR graph for trial mix responses
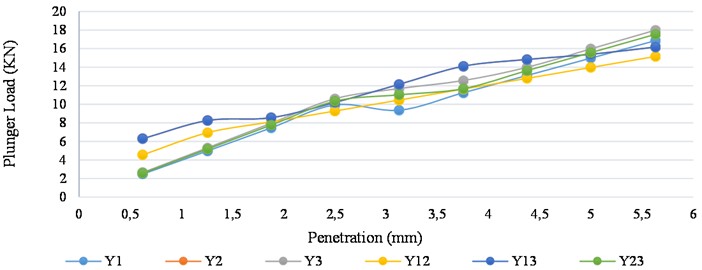
The CBR experimental values derived for each responses shows an increase in the experimental CBR values for the treated soil in comparison with the natural soil, which had a soaked CBR value of 70.14 %. The graphical representation from Fig. 2 shows that the mix design derived from the implementation of the Scheffe’s method gives a maximum soaked CBR value for the trial mix as 80 %, corresponding to the response space and a maximum CBR value for the control point as 77 %, corresponding to the and response spaces.
Fig. 4Experimental soaked CBR graph for control mix responses
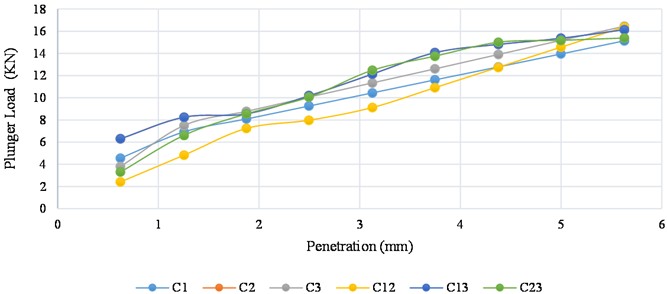
3.3. Model results
The computation of the results from the model formulated using Scheffe’s method was derived by substituting the values for the model coefficients, Pseudo components and further simplifying. The model equation stated in Eq. (16) as; . According to Scheffe, the values for , , and corresponds to, or are equal to the response values, , and respectively. Other model coefficients; , , and are derived using Eq. (18). Therefore, re-calling Eq. (17) and Eq. (18), the model coefficients are computed. The values computed are presented in Table 6.
Table 6Model coefficients for soaked CBR
Response space | Response values | Coefficients | Coefficient computation | Coefficient values |
75 | 75 | |||
77 | 77 | |||
80 | 80 | |||
70 | –24 | |||
77 | –2 | |||
78 | –2 |
Fig. 5Model and experimental results comparison
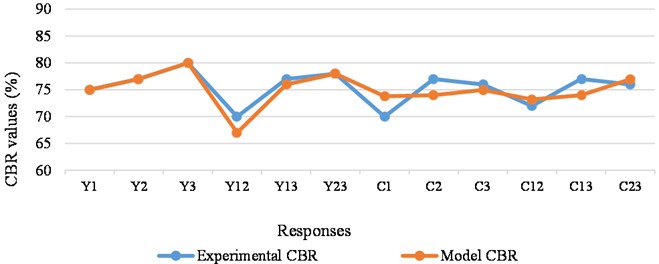
The values of the coefficients and corresponding pseudo component values are substituted into Eq. (16) to obtain the model equation for predicting the CBR values of the soil treated with RHA for soaked condition. The predictive model equation is derived as:
The predicted soaked CBR value is computed for each trial mix and control point using Eq. (33). The computation process is displayed in Table 7 and values are outlined for clarity in Table 8.
Fig. 5 displays a comparison between the experimental CBR values and the predicted values by the model. As seen in the Fig. 5, variations exist between both values as indicated by the displacements of the trend at specified responses. For responses , and , the experimental and predicted values are exact as depicted on the graph. The extent of variance between the both values (experiment and model) is analyzed in the model validation process using statistical methods and insights is drawn on the predictive adequacy of the model formulated.
Table 7Predicted soaked CBR for trial mix and control point
Responses | Calculation | Predicted CBR values |
75×1 + 77×0 + 80×0 – 24×1×0 – 2×1×0 – 2×0×0 | 75.00 | |
75×0 + 77×1 + 80×0 – 24×0×1 – 2×0×0 – 2×1×0 | 77.00 | |
75×0 + 77×0 + 80×1 – 24×0×1 – 2×0×0 – 2×1×0 | 80.00 | |
75×0.5 + 77×0.5 + 80×0 – 24×0.5×0.5 – 2×0.5×0 – 2×0.5×0 | 67.00 | |
75×0.5 + 77×0 + 80×0.5– 24×0.5×0 – 2×0.5×0.5 – 2×0×0.5 | 76.00 | |
75×0 + 77×0.5 + 80×0.5 – 24×0×0.5 – 2×0×0.5 – 2×0.5 ×0.5 | 78.00 | |
75×0.4 + 77×0.3 + 80×0.3 – 24×0.4×0.3 – 2×0.4×0.3 – 2×0.3×0.3 | 73.80 | |
75×0.3 + 77×0.4 + 80×0.3 – 24×0.3×0.4 – 2×0.3×0.3 – 2×0.4×0.3 | 74.00 | |
75×0.3 + 77×0.3 + 80×0.4 – 24×0.3×0.3 – 2×0.3×0.4 – 2×0.3×0.4 | 74.96 | |
75×0.6 + 77×0.2 + 80×0.2 – 24×0.6×0.2 – 2×0.6×0.2 – 2×0.2×0.2 | 73.20 | |
75×0.2 + 77×0.6 + 80×0.2 – 24×0.2×0.6 – 2×0.2×0.2 – 2×0.6×0.2 | 74.00 | |
75×0.2 + 77×0.2 + 80×0.6 – 24×0.2×0.2 – 2×0.2×0.6 – 2×0.2×0.6 | 76.96 |
Table 8Experimental results and model result
Responses | Soaked CBR experimental result (%) | Predicted CBR values (%) |
75 | 75.00 | |
77 | 77.00 | |
80 | 80.00 | |
70 | 67.00 | |
77 | 76.00 | |
78 | 78.00 | |
70 | 73.80 | |
77 | 74.00 | |
76 | 74.96 | |
73 | 73.20 | |
77 | 74.00 | |
76 | 76.96 |
3.4. Model validation and analysis
The Null hypothesis, which states that; “there is no significant difference between the experimental CBR values and the model values”, as well as the Alternate hypothesis that states that; “there is a significant difference between the results of the experimental CBR and the model results”, were tested. The model validation was conducted using the 2016 version of the Microsoft Excel application software. The data analysis tool pack on the software was activated and used to run the F-test analysis. The results are shown in Table 9. Manual analysis of the F-test is further outlined in Appendix.
Results from the F-test gave the F-stat value for soaked CBR 4.34 with a corresponding F-critical value as 5.05. The F-test was conducted at a confidence level of 95 %. Therefore, with the value of the F-stat being less than the F-critical value, the result is indicative that the variance between the experimental CBR values and the Predicted values is insignificant.
In other words, there is no significant difference between the results of experimental CBR and the model predicted results. Hence, the null hypothesis is accepted and the model is adequate.
Table 9Fisher’s test result (f-test) for soaked CBR
Description | Experiment | Model |
Mean | 74.83333 | 74.48667 |
Variance | 7.766667 | 1.788427 |
Observations | 6 | 6 |
Degree of freedom (Df) | 5 | 5 |
F-statistics | 4.342737 | |
P () one-tail | 0.066442 | |
F critical one-tail | 5.050329 |
4. Conclusions
The following technical insights were drawn from all experimental procedures, test results, validation technique as well as reviews.
The natural soil was classified as per AASHTO as an A-2-4 soil, having low plasticity, as seen in its low plasticity index.
The RHA used for the stabilization improved the mechanical property of the soil as seen in an increase in its CBR value.
Models constants using Scheffe’s method were formulated for the prediction of the CBR of the soil stabilized with RHA.
The optimal mix ratio for the CBR of the soil treated with RHA was 1:0.75:0.17, corresponding to Soil, RHA and Water respectively, having a Soaked CBR value of 80 %.
The model equation formulated for obtaining the CBR value of the RHA treated soil for soaked condition was obtained as; .
The models demonstrated adequacy and reliability in predicting the CBR value of the soil treated with RHA.
The F-test validation technique gave the F-stat value for soaked CBR as 4.34 with a corresponding F-critical value as 5.05, conducted at a confidence level of 95 %.
The F-stat value of 4.34 being less than the F-critical value of 5.05 is indicative that there is no significant difference between the experimental CBR values and the predicted CBR values for soaked condition.
The CBR value of the treated soil was observed to decrease at some response point.
References
-
C. A. Diugwu, “Modelling and optimization of the effect of rice husk ash on the California bearing ratio of Agbani soil,” Enugu State University of Science and Technology, Agbani, Enugu State, 2024.
-
G. Gupta, “Soil stabilization by using lime rice husk,” International Journal of Modern Developments in Engineering and Science, Vol. 1, No. 8, pp. 54–59, 2022.
-
G. U. Alaneme et al., “Mechanical properties optimization and simulation of soil-saw dust ash blend using extreme vertex design (EVD) method,” International Journal of Pavement Research and Technology, Vol. 17, No. 4, pp. 827–853, Jan. 2023, https://doi.org/10.1007/s42947-023-00272-4
-
C. Nwaobakata and K. E. Ohwerhi, “Optimization model development for California bearing ratio prediction of metakaolin stabilized lateritic soil,” Uniport Journal of Engineering and Scientific Research (UJESR), Vol. 5, pp. 41–50, 2020.
-
O. A. Oguaghamba, F. O. Okafor, and V. C. Anokwute, “Application of Scheffe’s model for stabilization of Amuro-okigwe subgrade using male inflorescence of oil palm ash,” Nigerian Journal of Technology, Vol. 38, No. 1, pp. 60–65, Jan. 2019, https://doi.org/10.4314/njt.v38i1.9
-
U. Okonkwo, “The optimization of bagasse ash content in cement stabilized lateritic soi,” University of Nigeria Nsukka, Nigeria, 2015.
-
U. E. Ike, “Optimization of the California bearing ratio of lateritic soil stabilized with rice husk ash using Scheffe’S. method,” Enugu state University of science and technology, 2022.
-
I. U. Elvis and I. C. Chinwuba, “Optimization of the California bearing ratio of lateritic soil stabilized with rice husk ash using Scheffe’s method,” Jurnal Kejuruteraan, Vol. 35, No. 3, pp. 705–715, May 2023, https://doi.org/10.17576/jkukm-2023-35(3)-19
-
C. C. Ikpa, “Scheffe optimization of the California bearing ratio of a kaolin blended lateritic soil for pavement construction,” Enugu University of Science and Technology, 2022.
-
C. C. Ikpa, C.C. Ike and G. U. Alaneme, “Scheffe optimization of the California bearing ratio of a kaolin blended lateritic soil for pavement construction,” Journal of Silicate Based and Composite Materials, Vol. 75, No. 2, pp. 58-66, 2023, https://doi.org/10.14382/epitoanyag-jsbcm.2023.9
-
“Standard Specifications for transportation, material and method of sampling and testing,” American Association of State Highway and Transportation Official, 1986.
-
“Standard practice for classification of soils for engineering purposes (unified soil classification system),” American Society for Testing and Materials, 2006.
-
“Methods of testing soils for civil engineering purposes,” British Standard 1377, 1990.
-
H. Scheffe, “Experiments with mixtures,” Journal of the Royal Statistical Society, Ser. B, Vol. 20, pp. 344–360, 1963.
About this article
The authors have not disclosed any funding.
The datasets generated during and/or analyzed during the current study are available from the corresponding author on reasonable request.
Diugwu Chijioke Ambrose: methodology, laboratory testing, model development, data analysis and writing of the paper. Ike Charles Chinwuba: ideology and formulation of the research, supervision, review and editing
The authors declare that they have no conflict of interest.