Abstract
Non-linearity is considered to be an essential property of complex systems. The associated high sensitivity of the result on the constraints leads to fundamental problems of a system description based on variables selected in the reductionist tradition. The attempt to compensate the problems by averaging data leads to the neglect of the individual and the moment. However, both is of enormous importance for effective therapy, training, and learning. The theory of differential learning suggests an alternative approach to dealing with these problems. With constantly changing complex whole-body movements, extensive decisions are demanded from the learner, which lead to brain states through an overstraining of the working memory, as it were, as they are also known after mindfulness meditation.
1. Introduction
There is no doubt about that human movements can be considered as one of the most complex systems in science. The same we would agree on motor learning. However, not all models on movements or learning approaches in movement science are complex. When complex systems are studied, it seems helpful first to distinguish the terms complex and complicated, since both are often applied synonymously in everyday language. Something can be complex without being complicated or complex because it is complicated. With a more differentiated reflection the term complicated is associated with being difficult and often depends on the number of elements or variables to be controlled, whereas the term complex relates to being entangled or inscrutable due to the kind and amount of the variable’s interactions. Therefor systems that are only defined by means of many elements, like a car, are complicated and their behavior fortunately is predictable. In contrast when the system contains internal feedback loops, memory, is nested, or the boundaries of the systems and subsystems are difficult to determine then the system becomes complex and increasingly unpredictable [1]. The limited predictability of complex systems is closely related to their non-linearity, which implies a high sensitivity of the system on the initial boundary conditions. Although the specification of all constraints is considered to be a basic prerequisite for a scientific approach at least since the Hempel-Oppenheim scheme [2], the fundamental undertaking to describe complex systems by means of a reductionistic specification of selected constraints, in order to predict future behavior, becomes obvious. Nevertheless, although human movements can be understood as complex systems, they seem to be predictable to a certain degree. For this purpose, hierarchical and heterarchical models of control have been suggested [3]. The first is mainly based on the assumption of a single, central institution that organizes the other elements in a ranked order, whereas the later favors a system organization without ranking. System dynamics provided a model for the emergence of the heterarchical control. The model of self-organization [4, 5] no longer required a complete description and control of each single element but stimulates the whole system by supplying diffuse energy and lets it find its own order. In self-organization processes a phenomenon is the omnipresence of fluctuations and their increase before the switch from one stable state towards another happens. By increasing the fluctuations, different combinations of the system elements are tested for an optimal mode. During such a transition, a system becomes instable and takes advantage of a mechanical principle according to which instability can be characterized by the fact that only little effort is needed to change the existing state. This abstract principle inspired the rethinking of models about motor learning.
A first period of describing and provoking these phenomena extensively and exclusively in various cyclic movements [6] was followed by a period in which similar phenomena were discovered in some gross motor movements and coarsely described with the terminology of coordination dynamics [7, 8]. Thereby the observed fluctuations became reinterpreted as being functional but still were considered as indirect effects of adaptation processes and practical implications were rather speculative. Increasingly detailed biomechanical analysis of movement patterns shifted the focus more on variability in performance. First the reinterpretation of fluctuations found in biomechanically described learning processes of high performance athletes [9] as a major characteristic for the identification of individuals on the basis of their movement patterns [10, 11] together with the exploitation of fluctuations by increasing them as a training intervention [14] allowed the transfer of the system dynamic principles not only to ballistic gross motor movements. The rethinking about motor learning processes that have been criticized for a while [12] became intensified [13]. Thereby the actively increased fluctuations during learning processes in form of stochastic perturbations were understood as potential sources for learning that fulfill the criterion of an instability and should be increased in order to make the system more instable for more effective and self-organized learning [14]. Destabilizing the learner by means of increasing fluctuations as a kind of diffuse energy supply, not only allows the learner to find his or her own optimal organization for the actual moment but also supports to find his or her “self”. By introducing not only the individuality of learning processes but also their situatedness an widening and tolerance for alternative approaches was suggested. The classical discussions about right and wrong techniques in sports or learning approaches became strongly relativized.
By increasing the number of fluctuations in general, in detail the differences between two successive movements are increased as well, thus giving access to an additional source of information for the learner. Because the information about the comparison of two subsequent movements was assumed to have an influence on the learning progress that is dependent on the individual as well as on the situation, the differencial learning approach was embedded in a theory of simulated annealing (SA) and stochastic resonance (SR) [15, 16]. The first part (SA) maps an earlier phase of learning, in which the variations are more extensive and move to the second part of the model for a more specific range, in which smaller variations are applied in the form of SR. The term SR comprises two indications of possible underlying mechanisms for optimal learning. The term resonance reflects the relative tuning of two signals. One signal was associated with “noise” that was caused by the intended fluctuations of the boundary conditions of the executed exercise and the other signal reflected the individual and its situation that can be dependent on emotions, music [17], fatigue [18] or time [19, 20]. Quantitatively, the boundary conditions of the executed exercise can be specified by the structure of the changes of the biomechanical variables, whereas the individual potential can be specified by the psycho-physiological state of the learner e.g. by means of brain-[21], heart-[22], etc. signals. Both signals were assigned stochastic character that could be associated with different colors of noise or the associated various frequency distributions.
A problem was and still is the usage of the term “noise”, because in science it is rarely applied in the sense of nuisance but often is associated in the narrow sense with “white noise” that requires equi-distant sampling rates. These are rarely given in everyday learning processes. In consequence, because of the individuality and situatedness of movements and learning the optimum learning noise of the SR curve can be at different “noise” of the executed exercises. This means for someone repetition could be the optimum “noise” in exercises and for someone variable training could be best, for another learner sometimes “methodical rows of exercise” could be best for optimum learning rates and sometimes “contextual interference” approach could lead to optimal learning strategy (Fig. 1). With the model of SR the theory of differencial learning suggested to unify all common motor learning approaches considering them as only differing in the amount or structure of noise. The first published example of DL in the narrow sense (DL i.n.s.) was just a practical derivation and consequence of the underlying DL theory. With this, DL theory assumes that even in the repetitive approach learning mainly happened because of the little deviations and for most learners these deviations are too small for optimal learning progress. Derived from biomechanical analysis of learning processes variations for a broad spectrum of variables have been suggested for the realization. These variations were biomechanically related to all joints, and because all the human joints contain sensors that can be assigned to joint angles, joint angular velocity, joint angular acceleration and rhythm, variations of them were included. These variables were also chosen because all associated sensors transmit signals to the central nervous system that were already simulated in the beginning with the help of artificial neural networks.
Fig. 1Differencial learning in the context of stochastic resonance
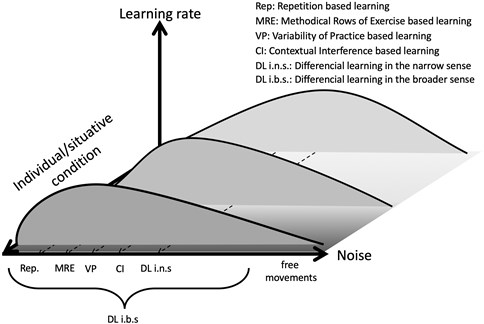
A sports pedagogical transcription of the idea of learning on the basis of increased noise is provided by the ‘non-linear pedagogy’ approach [23] with numerous practical examples how to cause movement noise in the acquisition process mainly by means of varying equipment or changing exercise conditions that cause the corresponding joint variations. An additional shift of focus of the structure of interventions from biomechanical variables towards an earlier suggested model [24] resulted in the ‘constraints led approach’ [25] which corresponds to a mix of the guided discovery and divergent discovery teaching style [48]. Accordingly, the interventions that cause corresponding noise were classified into three groups that are related to environment, tasks, and subject. However, by guiding (`led`) athletes through a series of supposedly correct exercises the idea of self-organisation is largely abandoned and the danger of focusing on athletes with a specific learning style increases.
Meanwhile ample evidence for a constructive influence of increased motor noise is provided from various areas of research (birds [26], eye-hand coordination tasks [27-29] artificial laboratory tasks [30]). Beside applications in different fields of sports techniques (shot put [31], hurdles [32], high jump [33], tennis [34]; football [35], basketball [36]) and sport tactics [37, 38] the DL theory was successfully applied in non-sports related areas such as music learning [39], handwriting skill acquisition [40], focal dystonia [41]; stroke rehabilitation [42] and even to office environments [43].
On the search for neurophysiological conditions of DL EEG studies provided evidence not only for clearly different brain activations post training in comparison to most common motor learning approaches but also for higher alpha and theta power in the prefrontal areas that are frequently assumed to support learning processes in general and which were seen previously mainly during sleeping and meditation [21, 44]. Similar phenomena had been observed after practicing endurance sports like running or cycling for a longer time and resulted in the hypo-frontality hypothesis [45]. In a more detailed analysis of brain physiology, one of the causes was suspected to be in the reticular activating system and there in especially the locus coeruleus (LC), which projects to the prefrontal cortex. Especially the phasic LC activity occurs in response to novel, stressful, and rewarding events and exerts a potent modulatory influence on executive functions such as inhibiting the processing of irrelevant stimuli or keeping task-relevant information “online” in working memory [46]. The low-frequency bands of the alpha and theta frequencies in the prefrontal cortex are associated, among other things, with the integration of information from different areas of the brain [47]. Similar to other areas in nature, e.g. the communication of elephants or whales, it seems that the lower frequencies are used in order to bridge bigger distances.
While fine motor movements seem to bring the prefrontal working memory to its capacity limits mainly by the length of a e.g. keystroke task or by the number of different task sequences to be stored, differencial learning i.n.s. with the constantly changing whole body movements probably forces the prefrontal lobe much faster to change its strategy in order to find additional resources for the solution of the problem by accessing additional areas of the brain. Whole body movements seem to be connected with more decisions in parallel in comparison to classical dual tasks. It is speculated, that differencial learning i.n.s. is a kind of overloading the prefrontal control unit with too many decisions in order to get access to an even bigger working memory. Because the most studies on working memory were conducted with fine motor movements more work is demanded on the connection of working memory in whole body movements. Regardless of the models of information processing, in practice DL seems to enable a capability that is often associated with a goal intended by mindfulness meditation: learning to be in the moment. By constantly changing the movements without consciously judging the previous movement, because after several task changes it will be impossible to memorize one of the previous events in detail, the learner seems to learn to be in the moment. If this is pursued, additional less memory capacity seems to be required and more working memory is available for the actual movement.
2. Conclusions
Departing from the nonlinearity of complex systems and their high sensitivity on the constraints we arrived at a more abstract model for heterarchical control of human movements and learning by means of self-organization that is realized by the differencial learning approach. DL in the narrow sense has been applied successfully in numerous complex sports movements by adding stochastic perturbations to single and multiple elements of the whole movement system. Numerous decisions during this learning procedure had to be made by the learner to get the continuously changing boundary conditions somehow under better control. Surprisingly, the overstraining of the central nervous control by too many decisions seems to alter the brain states towards a more distributed activation that is comparable to meditative states and seems to be advantageous for learning. How much overstraining is necessary for each individual in the specific situation may open a new research program. Finding principles in this process will be even more challenging.
References
-
Randall A. Risc and Precaution. University Press, Cambridge, 2011.
-
Hempel C. G., Oppenheim P. Studies in the logic of explanation. Philosophy of Science, Vol. 15, 1948, p. 135-175.
-
Mcculloch W. A heterarchy of values determined by the topology of nervous nets. Bulletin of Mathematical Biophysics, Vol. 7, Issue 2, 1945, p. 89-93.
-
Foerster von H., Zof Jr G. V. Principles of Self-Organization. Pergamon Press, Oxford, 1962.
-
Haken H. Cooperative Phenomena. Springer, 1973.
-
Schöner G., Haken, Kelso J. A. A stochastic theory of phase transitions in human hand movement. Biological Cybernetics, Vol. 53, Issue 4, 1987, p. 247-257.
-
Newell K. M., Corcos D. M. Variability and Motor Control. Champaign, Human Kinetics, 1993.
-
Handford C., Davids K., Bennet S., Button C. Skill acquisition in sport: some applications of an evolving practice ecology. Journal of Sports Sciences, Vol. 15, Issue 6, 1997, p. 621-40.
-
Bauer H. U., Schöllhorn W. I. Self-organizing maps for the analysis of complex movement patterns. Neural Processing Letters, Vol. 5, 1997, p. 193-199.
-
Schöllhorn W. I., Bauer H. U. Identifying individual movement styles in high performance sports by means of self-organizing Kohonen maps. 16th International Symposium on Biomechanics in Sports, 1998, p. 574-577.
-
Schöllhorn W. I., Nigg B. M., Stefanyshyn D., Liu, W. Identification of individual walking patterns using time discrete and time continuous data sets. Gait and Posture, Vol. 15, Issue 2, 2002, p. 180-186.
-
Newell K. M. Skill Learning. Human Skills, Chichester, England, Wiley, 1981, p. 203-226.
-
Davids K., Newell K. M., Bennett S. Movement System Variability. Champaign, Human Kinetics, 2005.
-
Schöllhorn W. I. Individuality – a neglected parameter? Leistungssport, Vol. 29, Issue 2, 1999, p. 7-11.
-
Schöllhorn W. Differential teaching and learning of movement: through changed assumptions on new consequences. Czwalina, Hamburg, 2005, p. 125-135, (in Germany).
-
Schöllhorn W. I., Mayer Kress G., Newell K. M., Michelbrink M. Time scales of adaptive behavior and motor learning in the presence of stochastic perturbations. Human Movement Science, Vol. 28, Issue 3, 2009, p. 319-333.
-
Janssen D., Schöllhorn W. I., Lubienetzki J., Fölling K., Kokenge H., Davids K. Recognition of emotions in gait patterns by means of artificial neural nets. Journal of Nonverbal Behavior, Vol. 32, Issue 2, 2008, p. 79-92.
-
Janssen D., Schöllhorn W. I., Newell K. M., Jäger J. M., Rost F., Vehof K. Diagnosing fatigue in gait patterns by support vector machines and self-organising maps. Human Movement Science, Vol. 30, Issue 5, 2011, p. 966-975.
-
Horst F., Eekhoff A., Newell K. M., Schöllhorn W. I. Intra-individual gait patterns across different time-scales as revealed by means of a supervised learning model using kernel-based discriminant regression. PLoS ONE, Vol. 12, Issue 6, 2017, p. e0179738.
-
Horst F., Kramer F., Schäfer B., Eekhoff A., Hegen P., Nigg B. M., Schöllhorn W. I. Daily changes of individual gait patterns identified by means of support vector machines. Gait and Posture, Vol. 49, 2016, p. 309-314.
-
Henz D., Schöllhorn W. Differential training facilitates early consolidation in motor learning. Frontiers in Behavioral Neuroscience, Vol. 10, 2017, p. 199.
-
John A., Schöllhorn W. I. Acute effects of instructed and self-created variable rope skipping on EEG brain activity and heart rate variability. Frontier in Behavioral Neuroscience, Vol. 12, 2018, p. 311.
-
Chow J. Y., Davids K., Button C., Shuttleworth R., Renshaw I., Araujo D. Nonlinear pedagogy: a constraints-led framework for understanding emergence of game play and movement skills. Review of Educational Research, Vol. 77, Issue 3, 2007, p. 251-278.
-
Newell K. M. Constraints on the development of coordination. Motor development in children: Aspects of coordination and control. Martinus Nijhoff, Dordecht, Netherlands, 1986, p. 341-360.
-
Davids, Button K., Bennett C. S. Dynamics of Skill Acquisition – a Constraints Led Approach. Human Kinetics, Champaign, 2008.
-
Tumer E. C., Brainard M. S. Performance variability enables adaptive plasticity of ‘crystallized’ adult birdsong. Nature, Vol. 450, 2007, p. 1240-1244.
-
Braun D. A., Mehring C., Wolpert D. M. Structure learning in action. Behavioral Brain Research, Vol. 206, Issue 2, 2010, p. 157-165.
-
Herzfeld D. J., Shadmehr R. Motor variability is not noise, but grist for the learning mill. Nature Neuroscience, Vol. 17, 2014, p. 149-150.
-
Wu H. G., Miyamoto Y. R., Gonzalez Castro L. N., Ölveczky Smith B. P. M. A. Temporal structure of motor variability is dynamically regulated and predicts motor learning ability. Nature Neuroscience, Vol. 17, Issue 2, 2014, p. 312-321.
-
Hasson D. J., Zhang Z., Abe M. O., Sternad D. Neuromotor noise is malleable by amplifying perceived errors. Plosone Computational Biology, Vol. 12, Issue 8, 2016, p. e1005044.
-
Beckmann H., Schöllhorn W. I. Differencial learning in shot put. 1st European Workshop on Movement Science, 2003, p. 68.
-
Schöllhorn W. I., Beckmann H., Janssen, Drepper J. Stochastic Perturbations in Athletics Field Events Enhances Skill Acquisition. Motor Learning in Practice. A Constraints-Led Approach. Routledge, London, 2010, p. 69-82.
-
Schöllhorn W. I., Michelbrink M., Welminsiki D., Davids K. Increasing Stochastic Perturbations Enhances Acquisition and Learning of Complex Sport Movements. Perspectives on Cognition and Action in Sport. Nova Science Publishers, New York, 2009, p. 59-73.
-
Schöllhorn W. I., Oelenberg M., Michelbrink M. Can mental training enhance the learning effect after differencial training? A tennis serve task. 12th European Congress of Sport Psychology- Sport and Exercise: Bridges Between Disciplines and Cultures, 2007.
-
Schöllhorn W. I., Michelbrink M., Beckmann H., Trockel M., Sechelmann M., Davids K. Does noise provide a basis for the unification of motor learning theories? International Journal of Sport Psychology, Vol. 37, Issues 2-3, 2006, p. 34-42.
-
Schönherr T., Schöllhorn W. I. Differencial learning in basketball. 1st European Workshop on Movement Science, 2003, p. 58-59.
-
Santos S., Coutinho D., Gonçalves B., Schöllhorn W. I., Sampaio J., Leite N. Differential learning as a key training approach to improve creative and tactical behavior in soccer. Research Quarterly for Exercise and Sport, Vol. 89, Issue 1, 2018, p. 11-24.
-
Coutinho D., Gonçalves B., Wong D. P., Travassos, Coutts A. J., Sampaio J. Exploring the effects of mental and muscular fatigue in soccer players’ performance. Human Movement Science, Vol. 58, 2018, p. 287-296.
-
Widmaier M. On the System Dynamics of Practice. Frankfurt, Schott, 2016.
-
Vehof K., Janssen D., Schöllhorn W. I. Writing Skill Acquisition in First Graders by Means of Differential Learning. Czwalina, Hamburg, 2009, p. 290.
-
Rosset Llobet J., Fàbregas Molas S. Rehabilitation and Plasticity of Task-Specific Focal Hand Dystonia. Treatment of Dystonia. Cambridge University Press, Cambridge, 2018, p. 256-260.
-
Repšaitė V., Vainoras A., Berškienė K., Baltaduonienė D., Daunoravičienė A., Sendžikaitė E. The effect of differential training-based occupational therapy on hand and arm function in patients after stroke: Results of the pilot study. Neurology and Neurosurgery, Vol. 49, Issue 3, 2015, p. 150-155.
-
Henz D., Schöllhorn W. I. Dynamic office environments improve brain activity and attentional performance mediated by increased motor activity. Frontiers in Human Neuroscience, Vol. 13, 2018, p. 121.
-
Henz D., John A., Merz C., Schöllhorn W. I. Post-task effects on EEG brain activity differ for various differential learning and contextual interference protocols. Frontiers in Human Neuroscience, Vol. 12, 2018, p. 19.
-
Dietrich A. Functional neuroanatomy of altered states of consciousness: the transient hypofrontality hypothesis. Consciousness and Cognition, Vol. 12, 2003, p. 231-256.
-
Audiffren M. The Reticular-Activating Hypofrontality (RAH) Model of Acute Exercise: Current Data and Future Perspectives. Exercise-Cognition Interaction, Elsevier, 2016, p. 147-166.
-
Cavanagh J. F., Frank M. J. Frontal theta as a mechanism for cognitive control. Trends in Cognitive Sciences, Vol. 18, Issue 8, 2014, p. 414-421.
-
Mosston M. Teaching in physical education. Merrill Publishing, Columbus, Ohio, 1981.