Abstract
Engineering asset management (EAM) has received a lot of attention in the last few decades. Despite this, industries struggle to identify the best strategies for maintaining assets. The decision-making around selecting a relevant maintenance strategy generally considers factors like risk, performance and cost. Risk management is, usually, largely subjective and industries consequently make investments in a subjective manner, making the allocation of budget unstructured and arbitrary. Generally, industries focus only on either overt risks or basic performance of assets, thus creating uncertainties in the decision-making process. Recently, however, maintenance decision-making has evolved from a subjective assessment, chiefly dependent on expert opinions, to utilizing live-data-sensor technology. The attitude towards component failures and how to address them has changed drastically with the evolution of maintenance strategies. Additionally, the emergence and use of several tools and models have assisted the drafting and implementation of effective maintenance strategies. These advancements, however, have only considered discrete parameters while modelling, instead of using an integrated approach. One of the primary factors which can address this shortfall and make the decision-making process more robust is the economic element. To enable an effective decision-making process, it is imperative to consider quantifiable determinants and include economic parameters while drafting maintenance policies. This paper reviews maintenance decision-making strategies in EAM and also highlights its relevance through an economic lens.
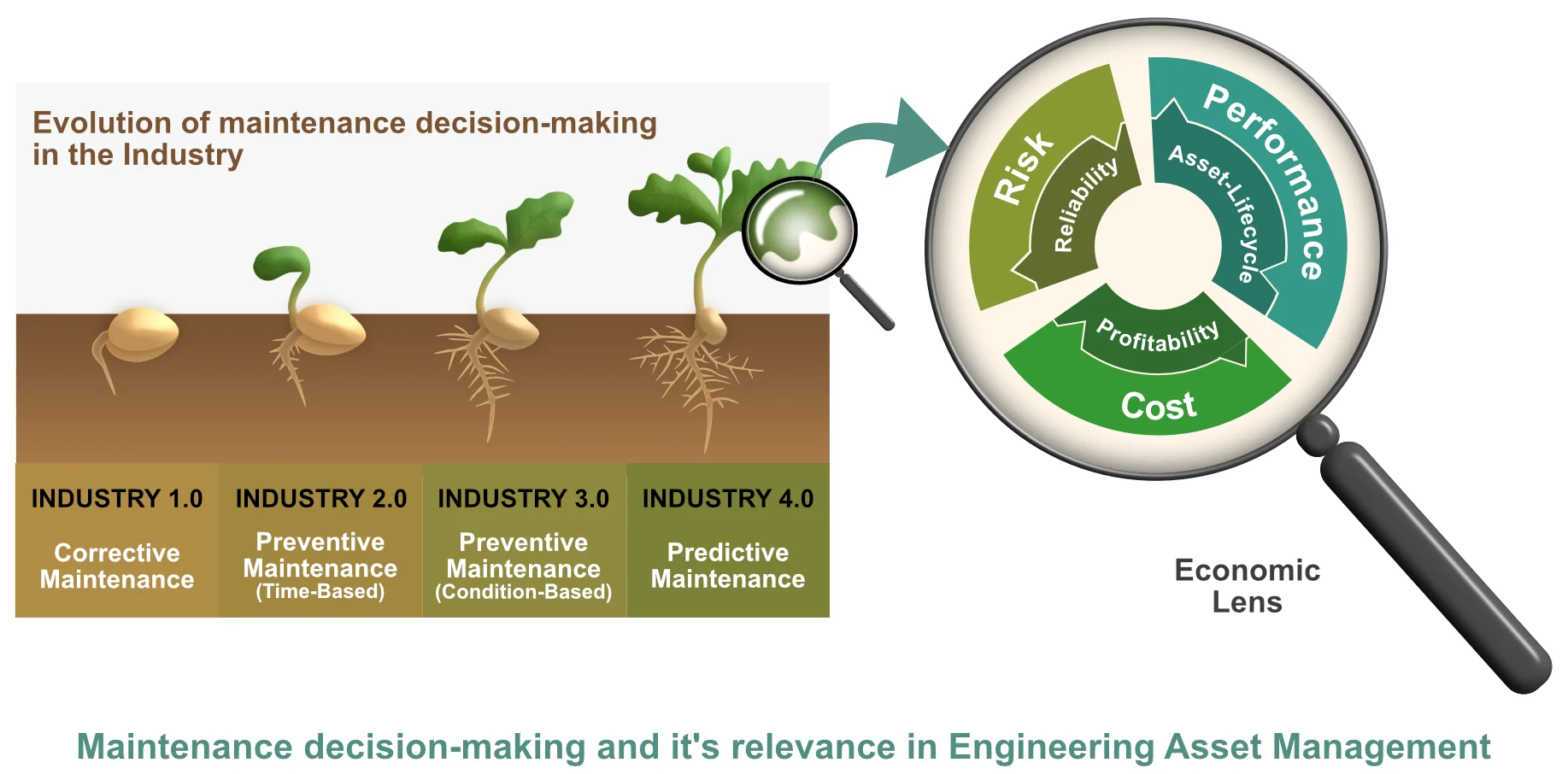
Highlights
- The criticality should be assessed at the granular level by analysing the failure modes of the sub-systems.
- The risk assessment should be well supported by quantitative parameters to remove the uncertainties from the maintenance decision-making process.
- The maintenance decision-making should be filtered through an economic lens by balancing the risk, cost and performance variables.
1. Introduction
The fourth industrial revolution has given a new dimension to decision-making in optimising the operational efficiency of industries. This phase was driven by technological revolutions and by focusing on the implications of reforming decisions in all domains of an industry as opposed to only a few areas of the industry [1]. One of the domains which isn’t given its due importance is asset management (AM). Today, industries are transforming to meet the competitive needs of the market with the help of industrial equipment and machinery. Ensuring that these assets perform at an optimal level and establishing processes to extract maximum value from them is essential to a firm’s growth and can impact profit-making to a huge degree. Firms, however, have floundered to understand the influence that good AM exercises over company performance. AM is usually relegated to the background in company policies, and firms fail to comprehend the impact good AM can make in not only generating substantial financial yields but also in deflecting certain risks, increasing the rate of output, and aiding overall company sustainability.
AM has established its importance in various types of industries. Process industries like oil and gas refineries, chemical plants, nuclear plants, mining industries, etc., are in the continuous production process, and here a reliable AM approach is critical to controlling business risk. The mining industry is a pivotal example of an industrial sector were establishing and implementing appropriate AM activities are imperative to efficient and productive functioning. It is prone to many uncertain and natural events, thus making its associated brand of issues and challenges complex and multidimensional. The mining industry operates in complex environments characterised by significant intrinsic uncertainties [2]. History has shown that an implemented solution at one mining site does not yield the same result at another. The reasons for this can often be linked to local conditions, culture and available resources which can substantially vary from site to site [3]. Consequently, the intensity of AM in this industry changes with respect to the mentality and approach towards risk management. Additionally, predicting future events is difficult due to knowledge gaps, system complexities and human fallibilities [4]. On account of the complexity and multi-dimensionality of the problems, the mining industry’s decision-making is generally based on certain integrated factors, for instance, risk analysis which is based on criteria like detectability, severity and occurrence of a failure mode. These criteria further help in understanding the availability, reliability, and maintainability of the systems. In the mining industry, selecting a maintenance strategy is a more challenging task in the decision-making process, because the stoppage of equipment leads to the stoppage of the entire manufacturing line. In such cases, the decision methods should focus on the system’s availability. Currently, in the mining industry decision-making processes for asset investment are largely qualitative, with assets being monitored and managed via qualitative FMEA tools or other risk-based methods.
Assets can be classified in 5 categories – human, information, financial, intangible, and physical, which must be managed holistically to achieve the organisational strategic plan. [5]. The Asset Management Council in Australia defines AM as the management of physical assets’ life cycle to achieve specific outputs and goals in an enterprise [6]. In the context of Engineering Asset Management (EAM), Davis [7] defines AM as the “continuous process improvement strategy for improving the availability, safety, reliability and longevity of plant assets, i.e., systems, facilities, equipment and processes”. EAM focuses on optimising assets through every stage of their life cycle including planning, acquisition, operation and eventual disposal as shown in Fig. 1. Implementing good AM strategies through the different stages of an asset’s life cycle can extend its output and life and ensures that maximum value is extracted from it [8].
Fig. 1Asset lifecycle management
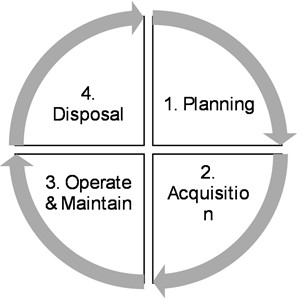
AM is not a new concept, as assets were always considered a hallmark of human activities. However, despite references from earlier times, organisations still struggle with the execution of AM [9]. Taking into consideration the importance of AM in the organisation management system, the first edition of Publicly Available Specification (PAS 55) was drafted and published in 2004 [10]. PAS 55, subsequently revised in 2008, was accepted by around 50 organisations from 15 different industry sectors in 10 countries [11]. Parallel to this, ISO project committee 251 (PC251) published the first international Am standard, ISO 55000, in 2014 [12]. The standards were based on the foundation of PAS 55-2008. AM is a reasonably comprehensive and challenging discipline to be consistently implemented in industries [13]. The American organisation, ISA (Instrumentation, Systems and Automation Society), has also drafted standards for AM, 2 of which - ISA-88 and ISA-95, have become international standards of significant importance in the AM sector [14]. The introduction of these standards provided industries with an internationally recognised terminology and framework for AM [15].
AM, until now, was considered only a mundane discipline. Perhaps it is time to start viewing it as a philosophy that needs to be transferred across different chains of hierarchy in an organisation. This helps create awareness across the organisation in understanding the need to optimise the performance of engineering assets, thus synchronising the organisation’s goals with AM goals. AM aims to enable an organisation to realise value from its assets as it pursues its objectives while balancing financial, environmental and social costs, risk, level and quality of service, and asset performance [9]. A sense of maturity is developing around the importance and significance of AM, which ensures reliability around the performance of the asset and takes us one step closer to the organisational targets. It plays a vital role in the business management of many industries, primarily to meet the ever-changing market conditions, maintain asset health, revise production targets and influence many other variables which can generate financial uncertainty and increase business risk. Understanding these business risks and how industries can use them to drive sound decision-making practices, minimise downtime, enable risk control, improvise operational efficiency, enable defect elimination, and increase overall market competitiveness has become strategically important [16]. The AM system plans and controls asset-related activities and their relationships to ensure that asset performance meets the intended competitive strategy of the organisation [17]. It is essential to align the AM system with organisational strategies and planning since AM works within an integrated framework of multi-disciplinary collaboration where the organisation, operation planning and performance are integrated and evaluated for continuous improvement [15].
The challenges in AM in the current industrial era generally include organisational challenges which entail the integration of all stakeholders across the organisation hierarchy for successful implementation and improvement of AM practice. Organisational challenges arise because of the organisation structural changes, which further give rise to decision-making challenges related to assets. Effective decision-making is necessary for predicting possible issues in asset life cycle and taking pre-emptive measures to combat the same, tailoring AM to industry specific issues, increasing asset lifetime to extract maximum value and aligning AM to support new emerging business models. For effective decision-making, metrics like information sharing, financial understanding, broader perspectives towards the organisational goals, risk assessment, etc. are imperative and which, if not tackled properly, can create challenges in the AM practice [18]. In financial considerations, cost challenges can change the decision-making dimensions and therefore it is necessary to invest the available budget wisely, which is again a part of effective decision-making. Thus, the focus of this paper will be on discussing the different strategies, tools and methods used for decision-making in AM.
The maintenance decision-making process in AM plays a crucial role in achieving organisational targets and objectives. To achieve maximum operational efficiency, the industry deals with issues by using various models as decision-making tools. Approaching maintenance management strategically and systematically has become essential for making the right choices [5]. However, it is critical to select relevant maintenance strategies, given that every strategy has its own strength and weakness, and that one-third of all maintenance costs are wasted as the result of unnecessary or unsuitable maintenance activities [19].
This paper reviews existing literature to summarise the existing strategies considered in making decisions in AM, highlights lacunae in the same and posits new strategies which can aid in efficient AM. Section 2 outlines the development and evolution of maintenance strategies in AM. Section 3 elaborates the primary characteristics of decision making in industrial maintenance. Section 4 further illustrates the different tools and methods involved in the maintenance decision-making process and identifies the critical factors associated with it. Finally, Section 5 critically reviews literature which highlights the significance of using an economic perspective in maintenance decision-making methods of EAM.
2. Evolution of maintenance strategies
According to Moubray [20], prior to 1950, maintenance strategy was not well defined, poorly executed and was largely reactive (implemented only in response to an event), leading to increased equipment downtime. The rapid change in manufacturing demands made the evolution of asset maintenance strategies critical to optimise the asset’s life cycle.
Fig. 2 depicts the evolution of maintenance strategies. The initial approach to maintenance before 1950 was known as “run to failure” or corrective maintenance (CM) because of its reactive approach to any failure. According to the European Standard EN 13306:2010, CM is defined as “maintenance carried out after fault recognition and intended to put an item into a state in which it can perform a required function” [21]. CM strategies have limitations such as, increasing downtime hours, and no possibility to optimise the operational performance with respect to cost and risk. Although most industries revised this strategy and gravitated to other maintenance strategies, this particular maintenance approach is still applied to low-risk engineering systems due to its low implementation cost [22].
Fig. 2Evolution of maintenance strategies [13]
![Evolution of maintenance strategies [13]](https://static-01.extrica.com/articles/23687/23687-img2.jpg)
In an attempt to minimise avoidable CM costs, preventative maintenance (PM) was employed with EN 13306:2010 defining it as “maintenance carried out at predetermined intervals or according to prescribed criteria and intended to reduce the probability of failure or the degradation of the functioning of an item” [21]. PM encouraged maintenance practices before an actual failure event, thus preventing the need for corrective measures and ensuring optimal performance with respect to cost and risk, making it a better alternative to CM. This approach employs planned strategies implemented at specific intervals of time to ensure continuous operational performance in the case of high-risk engineering systems. PM is the most common strategy applied in the industry and is further classified as time-based PM and condition-based PM [22].
Time-based maintenance dominated the second generation of the evolution of maintenance strategies. It entails administering maintenance of assets at regular intervals, irrespective of their current state [22]. Certain types of complex systems are difficult to monitor, and methods that consider only the condition of assets require substantive data, which might not be available or difficult to procure [22]. In such cases, a regular time-based preventive approach can be more beneficial. Additionally, specific components reflect defective symptoms prior to the failure event, for example, when a component is inspected for the defect and replaced to prevent the failure. In such cases, time-based inspections also benefit the overall maintenance system, reducing costs and decreasing risks [22]. At the same time, as mentioned earlier in this section, preventive maintenance occurring at regular fixed intervals like time-based inspections or maintenance leads to planned downtime [23].
The third generation of the evolution focused on condition-based maintenance (CBM). CBM is defined as “preventive maintenance which includes a combination of condition monitoring and inspection and/or testing, analysis and the ensuing maintenance actions” [21]. This maintenance policy works on a predictive approach. CBM provides options to perform maintenance activities before the occurrence of system/component failure, thus reducing the uncertainty of maintenance activities. The primary focus of CBM is fault detection, diagnostics, degradation monitoring and failure prediction [22].
The fourth generation in the evolution of maintenance strategies addressed risk management and reliability of the systems. Predictive Maintenance (PredM) dominates this fourth generation and entails risk-based and reliability-centred maintenance (RCM) strategies. RCM keeps reliability of the asset at centre and thus leads to the formal definition of reliability-centred maintenance by Moubray [20] as “a process used to determine the maintenance requirements of any physical asset in its operating context”. The results of applying RCM has been highlighted in a case study by Afefy, et al. [24] based on data of a Fayoum Sugar Works Company in Egypt, which produces Sugar. The results show that on applying corrective and preventive maintenance the downtime decreased by 55.77 % and 52.17 %, respectively, which further lead to a saving in the total maintenance cost by 52.17 %. It was shown that the proposed RCM saved about 6.19×106 L. E (Egyptian Pound) in total maintenance cost. Moreover, the results revealed that the availability increase from 57.1 % to 90.74 % and reliability increased from 99.73 % to 99.88 % [24].
Maintenance strategies are optimised with the help of different tools such as Machine Learning, Artificial Intelligence, and many others to improve condition monitoring. However, PredM can prove costly as it encompasses hiring and training technical experts and staff, setting up and integrating comprehensive software packages into the overall process and is economically viable to be used only for high-risk systems. Implementation of PredM can be justified by the value added to the overall process. Although PredM is an expensive tool to employ, it is advantageous to implement it in combination with other maintenance strategies to extract maximum benefit for the industry [22].
Maintenance data analytics is another perspective of maintenance strategic planning and can be categorised in 4 phases as shown in Fig.3. Descriptive analytics answers the question ‘What happened?’ by providing information about previous maintenance operations. Diagnostic data analysis can respond to ‘Why it happened?’ by identifying causes. Predictive analytics estimates future events (what will happen, when?) by learning from historical maintenance data (possibly in real-time). Prescriptive analytics can respond to ‘What should be done?’ by providing actionable recommendations for decision making and improving and/or optimizing forthcoming maintenance processes. Both descriptive and diagnostic analytics methods are reactive while predictive and prescriptive analytics approaches are proactive.
Fig. 3Maintenance analytics phases
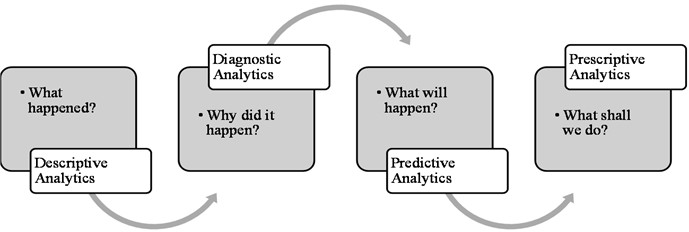
In summary, devising and choosing maintenance strategies can involve multiple dimensions and perspectives. However, most industries refer to a basic classification system. Fig. 4 explains the general classification of maintenance strategy types.
3. Elements involved in industrial maintenance decision-making
The decision-making process in AM focuses on optimising, reducing and enhancing cost, risk and performance, respectively. All three variables are equally important and critical to manage. Finding the right balance among these variables requires planning. Optimising cost helps in managing the budget, reducing risk helps in increasing the life cycle of an asset and enhancing performance helps in meeting the ever-increasing demands of the competitive market. Even in this era of transformation, some companies operate in a survival mode and still approach the subject of AM reactively. The industry lacks the urge for continuous improvement, which is one of the essential elements for the successful implementation of the AM system. Its focus predominantly lies around cost saving, thus ignoring the investment in relevant maintenance strategies. This is very generic as every organisation is bound by an annual maintenance budget that must be utilised wisely. To use the available budget effectively, organisations opt for scheduling maintenance strategies without analysing the current state of the assets. This decision-making approach leads to more expenses. Scheduled maintenance reduces the risk and enhances performance, but the cost gets affected, thus disturbing the balance between these variables. A potential risk, if detected in time, can save a lot of downtime and maintenance cost and the definition of this potential risk is very subjective. This gives rise to uncertainties in the decision-making process thus affecting the performance. Thus, prioritising and attending the risk with respect to criticality can not only enhance the performance but can also help in cost saving and, at the same time, help in balancing these variables.
Fig. 4General classification of maintenance strategy types
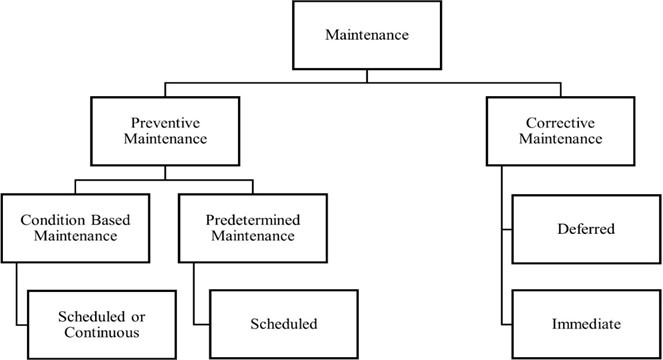
Generally, the decision-making in a maintenance management process is divided into two parts: the definition of the strategy and the strategy implementation. The critical element is to align the initial part, i.e., defining the strategy, with the overall business strategy, which aids the success of the maintenance activities in an organisation. Maintenance objectives derived from the business plan act as an input in defining the maintenance strategy process. The second part of the process focuses on implementing the selected strategy. It reflects an organisation’s ability to deal with maintenance strategy implementation problems and minimising the direct maintenance cost [25]. Table 1 highlights some significant work carried out in the field of maintenance decision-making.
The senior management plays a vital role in defining the above discussed maintenance activities. The maintenance management process can be streamlined more with effective decision-making. The organisation’s senior management strategies should be transparent at every level of the hierarchy, thus aiming for an efficient implementation of the planned strategy. The decision-making process depends on various critical factors, which differ with the industry type and stakeholder needs, as highlighted in Fig. 5.
Table 1Significant work carried out in the field of maintenance decision-making
Author | Contribution | Research gaps |
Maintenance planning | ||
Wan, et al. [26] | Developed a collaborative platform between the production and maintenance departments for ease of communication and planning maintenance activities | It is challenging to implement such a collaborative platform for sharing information between different stakeholders of the system in an industry, as there might be loss of informative data due to lack of training, negligence by operators, feeding of wrong information, and other human error |
Terkaj, et al. [27] | Developed an ontology-based virtual platform that consisted of a multi-layered integration of the information related to hardware, software, human resources, etc | |
Selecting a maintenance strategy | ||
Jamshidi and Esfahani [28] | Developed a bi-objective mathematical model for preventive repair and replacement schedules to minimise the total cost and maximise the reliability of the entire system | The model assumes the repair and replacement time as negligible whereas repairing and replacement activities are generally time consuming thus making the preventive and corrective maintenance inefficient from cost optimisation |
Ding, et al. [29] | Generated a maintenance policy selection model with three phases including identification of the critical system, further failure modes that are identified by Failure Mode Effect and Analysis (FMEA) and Multi-Criteria Decision-Making (MCDM) to identify an optimal maintenance policy that can minimise the failures | The limitation of the model lies within the expert’s judgement and the accuracy of the information provided by them |
Maintenance management | ||
Bousdekis, et al. [30] | Focus on real-time sensor data for monitoring machine conditions to improve proactive maintenance strategy. The monitoring and data analysis framework method was also used to estimate predictions of failure | Although their paper focused on proactive decision-making to support condition-based maintenance and embed the e-maintenance concept, only the optimisation of the time factor is justified. Other elements of decision-making like spare parts inventory, logistics, production targets, etc. Along with the cost associated with the same need to be considered |
Martón, et al. [31] | Propose a new approach to Ageing Probabilistic Safety Assessment (APSA) modelling by incorporating effectiveness of maintenance, efficiency test and ageing factors as inputs. It is intended to support risk-informed decision making and their research focuses on the critical equipment of Nuclear Power Plants within the framework of the Risk-Informed Decision-Making | The work focuses only on one critical component, not the entire system or plant |
Verma, et al. [32] | Focus on Condition-based predictive maintenance (CBPM) and works with the Fuzzy Inference System in a multi-objective optimisation framework. It applies the genetic algorithm by using failure rate; preventive repair rate; corrective repair rate; costs of detectability, prognostic, logistics and failure etc. As inputs for providing several options for maintenance decision maker and suitable Condition Monitoring System | Estimates of detectability (d) and prognostic abilities (p) are based on expert elicitation which are further incorporated in the optimisation model. Due to this subjective approach, the risk of uncertainty arises in the decision-making process |
Fig. 5Maintenance decision-making critical factors
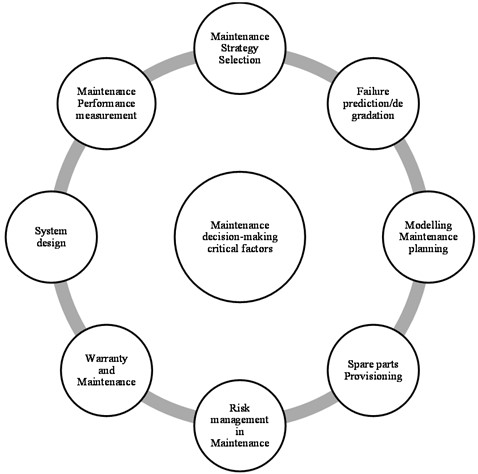
Along with the above critical factors of decision-making, Almeida and Bohoris [33] considered the following eight elements as the basic ingredients in a decision-making process: a) Elements like conditions and basic laws which define the natural calamities over which the decision maker has no control; b) Set of possible actions are derived after identifying the critical factor or analysing the risk which provides the decision maker with certain set of possible maintenance actions; c) Consequences are analysed by risk assessment framework either qualitatively or quantitatively to give an overview of the outcome of the decision; d) Function of loss and utility quantifies each outcome related to the derived set of actions; e) Multi Attribute utility theory is related to the multi criteria decision-making; f) Elicitation and consistency is based on how robust the decision-making model selected by the user is based on the consequences; g) Optimisation is the ultimate goal of the decision making process; h) Sensitivity Analysis helps in building a robust decision-making model and removing any uncertain factors from the process.
4. Tools and methods used in maintenance decision-making
Industries can utilise several tools and methods to assist maintenance decision making. Multi-Criteria Decision-Making (MCDM) aims to determine the best solution by using more than one criterion in the decision-making process. The implementation of MCDM has experienced a significant rise over the last several decades. It has helped develop new methods and improve old ones in different domains. The evolution of technology has created an awareness for developing more complex decision analysis methods. Velasquez and Hester [34] focused on developing a new approach to decision analysis by combining MCDM methods. Considering the same, Triantaphyllou, et al. [35] proposed the use of a decision method, the Analytic Hierarchy Process (AHP), considering four maintenance criteria: cost, reparability, reliability and availability. The methodology focuses on sensitivity analysis, which further investigates the robustness of the selected utility function by varying them [35]. AHP is used to derive relative priorities on absolute scales (invariant under the identity transformation) from both discrete and continuous paired comparisons in multilevel hierarchic structures. As many decision problems cannot be structured hierarchically, due to the interaction and dependence of higher-level elements with the lower level, a more general form of AHP known as analytic network process (ANP) method is suggested for the decision-making process which structures the decision-problem in a network [36]. ANP is a practical approach for determining the interdependency of maintenance strategies [37]. Although ANP has shown extensive strengths in allowing dependency and feedback in hierarchy and simplifying complex problems, it has its own limitations like relying heavily on experts’ judgements and experiences and multiple factors leading to unmanageable models [38].
The efficiency and the effects of interdependency of the different industry AM decision making systems can be analysed with the domino effect analysis. Domino effect analysis developed by Khan and Abbasi [39] includes two levels of study. The first level is a detailed analysis to identify units that may be considered as targets, and the second level is a detailed analysis made to verify the existence of the domino effect, using the potential damages of the primary event and the characteristics of the secondary unit. Khan [40] also developed the MCAS (Maximum Credible Accident Scenarios) methodology to evaluate all credible accident scenarios in an industrial unit. Scientists within industrial engineering have also investigated frameworks for quantitative risk assessment linked to domino effects. The risk assessment and evolution modelling of domino effects are divided into three categories: Analytical method, Graphical method, and Simulation method [41]. Many authors have developed comparative analytical methodologies for domino effect analysis. Cozzani, et al. [42] devised a systematic procedure for quantitative risk assessment caused by the domino effect. Antonioni, et al. [43] developed an analytic methodology based on historical data for the quantitative risk assessment of accidents triggered by seismic events. The main objective of this methodology is to identify the scenario, evaluate the credibility and assess the consequential event that may follow a seismic event. Renni, et al. [44] developed a procedure for the quantitative assessment of industrial risk caused by lighting in the form of a flowchart, and the main aspects of this methodology are the frequency and severity of an external event, the identification of target equipment, damage states, reference scenarios, estimation of damage probability, consequences calculation for the events, each combination of events, frequency/probability calculation for each combination and calculation of risk/hazard indices.
Graphical models provide a novel analytical framework for the joint evolution of domino effects, tackling complex domino scenarios and higher-order propagations [41]. Various graphical methods are used for the evolution of domino effects and for better representation of the scenarios. Types like graph/network models, graph metrics, dynamic graphs, Bayesian network, dynamic Bayesian network, petri-net models, etc. have been used in industries [41]. De Montis, et al. [45] used a weighted network representation where the vertices represent the towns and the edges represent the actual commuting flows among those towns. The advantage of using a network approach is that it allows one to uncover the rich structure in the flow pattern that could otherwise be difficult to extract and analyse. The limitation of the study is that it does not consider the uncertainties in the network approach leading to misguiding of the commuters. Graph theory has been applied to model causal frameworks inherent in the complex phenomenon of various science disciplines including infectious disease epidemiology, network traffic and neuroscience [46]. Graph metrics like the clustering coefficient and the characteristic path length are useful measures of global organisation of large-scale networks [47]. The clustering coefficient is a measure of local network connectivity. A network with a high average clustering coefficient is characterised by densely connected local clusters. The characteristic path length is a measure of how well connected a network is. A network with a low characteristic path length is characterised by short distances between any two nodes [48]. In a graphical representation of a brain network, a node corresponds to a brain region while an edge corresponds to the functional interaction between two brain regions. There are several statistical limitations to the study, for example there are no corrections applied for multiple testing and covariates like age are not adjusted in the non-parametric Kruskal-Wallis [48]. Abdolhamidzadeh, et al. [49] developed a methodology based on Monte Carlo Simulation and overcame some of these limitations. This new method, known as the FREEDOM algorithm, assessed the domino effect in the chemical process industry and was based on Monte Carlo simulation by conducting several hypothetical experiments to simulate the actual behaviour of a multi-unit system. Many of these methodologies were inherently limited in their ability to handle the uncertainty and complexity associated with domino effect phenomena. After analysing the available information on domino effects in the process industry and in some natural events, where the domino effects analysis is well supported by quantitative risk assessment framework, vulnerability models and graphical methods, the existing literature does not consider availability of the system as the criteria to analyse the domino effects. Any one model is not sufficient to arrive at an ideal decision-making process. These tools need to be exercised through an economic perspective to be able to extract the best possible value from them. Table 2 summarises the advantages and disadvantages of some of the above significant decision-making tools and methods.
Table 2Summary of advantages and disadvantages of some significant decision-making tools and methods
Tools and methods | Advantages | Disadvantages |
AHP | It allows structuring the decision-making problem into a hierarchy tree and facilitates understanding of the problem | Scoring and ranking in AHP depends on the alternatives considered for evaluation |
ANP | It allows dependency and feedback in hierarchy and simplifying complex problems by understanding the importance level that an attribute could take based on its correlation with other attributes | It relies heavily on experts’ judgements and experiences and the multiple factors leading to an unmanageable model |
Domino theory | It provides a very quick and simple investigation to determine reasons for the accident | It does not allow for effective analysis of the environmental factors – the factors external to the unsafe act |
Quantitative risk assessment | It helps in quantifying the risks and remove uncertainties from the process | It is a costly and time-consuming process |
Graphical models | It is simple and helps to understand and analyse the individual variables and their dependency on other variables | It is an overfitting and lack of robustness |
Simulation theory | It helps in detailed and risk-free analysis. | It lacks flexibility and accuracy |
Maintenance decision-making in industry is well supported by advanced tools and different models, as described above, but still lacks an integrated and objective methodology which can give the industry an overview of the impact that equipment failure events have on key business objectives. The major issues which might affect the decision-making process are the uncertainties which develop due to limitations of the implemented methods, and which affect the final solution. In most cases, the ultimate solution is constrained either by budget or a general unwillingness to adapt maintenance strategies in accordance with equipment condition. The subjective approach disintegrates the different parameters, instead of integrating them. The model generated in this manner results in poor management because the focus is generally based on the available investment cost and return on investment.
Many research studies have highlighted the integrated and hybrid solution for maintenance decision-making but the integration of cost, risk and performance is something which can be researched in the future scope of work. These three parameters can reduce maximum number of uncertainties from the process thus delivering a robust solution. Economic perspective in the decision-making process helps in prioritising and quantifying decisions, thus making it an important element in providing an ultimate robust solution.
5. Economic perspective in maintenance decision-making
One of the most important elements that needs to be considered in the AM decision-making process is economics, whereby all decisions are filtered through an economic prism. Industries operate on a constrained budget to carry out their operations and the decision-maker must use the resources wisely and make efficient and quick decisions. Thus, in the industry 4.0 environment, decisions which take into account economic elements play a crucial role in placing an industry in a competitive position. Asset owners taking care of complex systems have a certain maintenance budget available to them for use each year. The main challenge faced by the decision-makers is how to utilise this budget efficiently to maximise productivity and minimise the risk and cost to the business. The current approaches towards risk assessment depend on the amount of value put into addressing a risk event, which is ultimately dependent on expert opinion and experiences. This results in the designing of the risk assessment framework using largely qualitative inputs. It does not consider prioritisation of failure modes with respect to any quantitative factors like cost or failure rate. Generally, the application of a qualitative risk method is time consuming and costly. A routine maintenance strategy can lead to wastage of maintenance budget based on production loss due to unplanned downtime and neglecting any potential risk which needs to be attended to as priority. The inspection is only at the system level and not at the component level. Such approaches based on qualitative assessment are inefficient in channelising the maintenance budget.
Considerable work has been carried out around devising economic models which aid in decision making processes. Authors like Wu, et al. [50] highlight a decision model based on a novel cost model that analyses the expected degradation reduction and the preventive repairs cost. The model focuses on reduction of maintenance cost by managing and finding an optimal length of condition monitoring interval, which has a direct relationship with the monitoring cost. Although the model helps in monitoring the cost, it does not incorporate the time factor for preventive repairs. The article by Gilabert, et al. [51] focusses on assessment of predictive maintenance strategies with respect to certain business scenarios. It highlights the integrated methodology of existing reliability and maintenance business analysis techniques and standards. Haroun [52] presents the Activity-Based Costing (ABC) approach as an alternative to the traditional cost accounting system. ABC provides a costing method which centres around only those activities which are the primary object of interest. Faccio, et al. [53] suggest a quantitative framework with simple cost-benefits analysis to develop optimal maintenance policies. In order to eliminate possible uncertainties and to validate the decision-making process, simulations can be also carried out either in conjunction with the different models or exclusively. The Monte Carlo simulation, which is dependent on the reliability information, is based on the probability density function of failure for the system or component. Studies using Monte Carlo simulation have demonstrated that it positively impacts the cost-effectiveness analysis of maintenance strategies during the decision-making process. Yu and Wing-Keung [54] have developed an effective economic model by using the cost-benefit analysis method along with Monte Carlo simulation.
Cost optimisation is a widely used approach in planning maintenance activities. Louhichi, et al. [55] derived a cost model based on risk assessment for predictive maintenance, where different overheads of maintenance costs are derived along with its optimisation. The model is based on an objective function, which is the sum of all overheads of maintenance costs. The focus of the model is optimising the maintenance cost and the primary decision variable used is the Remaining Useful Life (RUL). An economic analysis method is presented in the paper by Berdinyazov, et al. [56], which helps in selecting the relevant maintenance policy with respect to the failure modes of the system. The objective function which needs to be optimised involves cost of each implemented maintenance policy. Vaurio [57] developed a cost model based on finite repair, maintenance durations and costs. The cost included factors like testing, repair, maintenance and lost production or accidents. Maillart and Pollock [58] analysed predictive maintenance policies and presented cost-minimising policies for systems exhibiting 2-phase behaviour. It also focused on determining the monitoring interval and allocation of monitoring resources. The expected cost (per unit time) is decomposed into two components: the expected cost due to maintenance actions, and the expected cost due to monitoring actions. Van Horenbeek and Pintelon [59] developed a dynamic predictive maintenance policy for complex multi-component systems aiming at minimising the long-term mean maintenance cost per unit time. Salonen and Deleryd [60] have modelled the costs of poor maintenance by studying the cost effects of quality in preventive and corrective maintenance. Tam and Price [61] have developed an investment decision model for maintenance based on the decision variables or dimensions like cost, capacity and compliance, which aims to minimise the sum of three cost categories: the costs of maintenance resources, the costs of planned downtime, and the costs of quantified risks.
Another widely used approach in maintenance strategy selection is Return on Investment (ROI). An economic evaluation of predictive maintenance techniques was proposed by Meng, et al. [62], which integrated system dynamics and evolutionary game modelling methods. In this paper, a hybrid methodology has been proposed to evaluate the economics of predictive maintenance technologies through ROI analysis. A Cost Benefit Analysis model is established by System Dynamics (SD) to estimate the value of ROI. An evolutionary game theory model is combined with the SD model to optimise the investment strategies of the enterprise. Wolf, et al. [63] suggest utilising commonly used business management concepts like Net Present Value and Internal Rate of Return due to their easy interpretation. They give an overview of the driving costs and profitability of predictive maintenance with an aim to support the decision-making process of investments in predictive maintenance. Cheng, et al. [64] present the widely used stochastic gamma process to model the system degradation and highlight the deviation of the probability distribution of maintenance cost. The proposed approach is useful for a precise estimation of prediction limits and optimisation of the maintenance cost. Dandotiya and Lundberg [65] proposed a methodology that combines the different optimum decisions into a single optimum decision while taking the economic and technical characteristics into consideration.
Another approach proposed by He, et al. [66] is introducing a decision variable defining the mission reliability state, which further reflects the production state of manufacturing system. The objective function is to implement and optimise the dynamic predictive maintenance strategy. The study focuses on two research questions. The first one is “when do we need to implement the predictive maintenance strategy?”, which is decided with respect to the mission reliability state of the equipment. Predictive maintenance strategy is implemented if the mission reliability state reaches it threshold. The second is “how to optimise the predictive maintenance strategy?”, which is obtained by minimising the comprehensive costs and its elements. The approaches taken by these studies are still very subjective towards the criticality analysis; there is a lack of quantification of risk, lack of integration of quantitative FMECA with the existing economic models, and lack of relative interdependency analysis study. In some cases, the decision criteria itself are assumed. Many MCDM methods depend extensively on expert opinions and has some applications limitations. The gaps which were observed in the economic model study include non-economic overheads such as labour contracts, undefined data ownership, missing human capital for necessary fields, etc., which must be considered to yield different results during the decision-making process.
In conclusion, maintenance decision making cannot be made in isolation without taking into account economic variables. Economic perspective is one of the main pillars of an effective and successful AM.
6. Conclusions
Over the years, the world has experienced many industrial catastrophic failures which has increased the awareness of EAM. EAM can achieve maximum success with a flexible but holistic decision-making framework. Despite past experiences, and even after having collected and analysed historical data, it is difficult to follow or benchmark a solution to a common repetitive failure because of the dynamic behaviour of a system and its dependency on other systems on the site. Thus, decision-making is much like a double-edged sword when it comes to selecting a maintenance strategy. Although industries understand the importance of EAM, they are still struggling in adopting relevant strategies suitable for maintaining their physical assets. Evolution of maintenance strategies followed the evolution of the industrial revolution. Upgrading maintenance strategies help industries gain the upper edge in a competitive market; the question which needs attention, however, is how many can afford these strategies?
It is important to approach the topic of maintenance holistically by considering the failure modes at a granular level. Even as a research topic, theoretical or practical, it is a very difficult task to carry out critical analysis at the component level considering ever changing environmental and operational conditions. This forces the industry to monitor or analyse the situation at the system level. The interdependency study of the equipment within a system of an industry cannot guarantee successful results in EAM, but it can definitely guide one towards an effective decision-making process. Different tools, methods and models have been designed to help decision makers select the ideal strategy in EAM, most of which have been highlighted in this paper. The aim of highlighted approaches is either to optimise the cost, reduce the risk or enhance the performance, while the ideal approach is to consider all 3.
Finally, in suggesting future research directions on maintenance decision-making and in also answering the earlier question on affordability, the focus should be on economic factors while deriving decision-making strategies, along with risk and performance. The reason for the same is that every organisation is bound by a specific annual maintenance budget, which is a constraint while applying affordable maintenance strategies. For instance, predictive maintenance, which comes with a high investment, helps manage the most critical components. But decision-makers might settle for preventive or corrective maintenance, which might only address less critical components, due to the constraint of managing their budget. Over time, with proper maintenance management the entire model can be shifted to predictive maintenance. Success is measured by financial performance not only for AM but also for any other segment in the industry. Therefore, decision making in AM cannot be divested from financial considerations. Decision making should be done through the prism of budgeting, while the degree of influence that the budget exercises over decision making may vary according to the scale and scope of the industry. Although research is only beginning to align with the importance of this perspective, it is irrefutable that economic factors will always be an indispensable element in decision making. Altogether, everyone understands the importance of using current trending predictive maintenance strategies, though the cost of their application is a constraint to the decision-makers. It would be advisable to accept the hybrid model of maintenance where different maintenance strategies are applied with respect to the risk level of the asset. This is becoming an emerging trend in many industries and recent research is bringing this kind of maintenance into the spotlight. This would allow the organisation to invest strategically, with respect to the organisation’s maintenance budget and help it gain a marked competitive edge.
References
-
H. Lasi, P. Fettke, H.-G. Kemper, T. Feld, and M. Hoffmann, “Industry 4.0,” Business and Information Systems Engineering, Vol. 6, No. 4, pp. 239–242, Jun. 2014, https://doi.org/10.1007/s12599-014-0334-4
-
D. Komljenovic, “Development of risk-informed, performance-based asset management in mining,” International Journal of Mining, Reclamation and Environment, Vol. 22, No. 2, pp. 146–153, Jun. 2008, https://doi.org/10.1080/17480930701562176
-
R. A. Carter, “Managing mobile assets,” Engineering and Mining Journal, Vol. 216, No. 4, p. 46, 2015.
-
J. K. Allen, S. Azarm, and T. W. Simpson, “Designing complex engineered systems,” Journal of Mechanical Design, Vol. 133, No. 10, Oct. 2011, https://doi.org/10.1115/1.4005079
-
M. S. Somia Alfatih, M. S. Leong, and L. M. Hee, “Definition of engineering asset management: a review,” Applied Mechanics and Materials, Vol. 773-774, pp. 794–798, Jul. 2015, https://doi.org/10.4028/www.scientific.net/amm.773-774.794
-
A. Shah and A. Kumar, “Review on Australian and international practices for asset management in building infrastructure,” Report 2001-010-C/004, Dec. 2003.
-
J. Davis, “What is asset management and where do you start?,” Journal AWWA, Vol. 99, No. 10, pp. 26–34, Oct. 2007, https://doi.org/10.1002/j.1551-8833.2007.tb08042.x
-
J. Amadi-Echendu, “Assessment of engineering asset management in the public sector,” in Lecture Notes in Mechanical Engineering, Cham: Springer International Publishing, 2014, pp. 1151–1156, https://doi.org/10.1007/978-3-319-09507-3_97
-
Hastings and Nicholas A. J., Physical Asset Management. London: Springer London, 2010, pp. 1–370, https://doi.org/10.1007/978-1-84882-751-6
-
B. Pas, “55-1: Asset management. part 1: Specification for the optimized management of physical assets,” PAS 55-1:2008, British Standards Institution, 2008.
-
Z. Ma, L. Zhou, and W. Sheng, “Analysis of the new asset management standard ISO 55000 and PAS 55,” in 2014 China International Conference on Electricity Distribution (CICED), pp. 1668–1674, Sep. 2014, https://doi.org/10.1109/ciced.2014.6991990
-
“ISO 55000: 2014: Asset management-overview, principles and terminology,” ISO Geneva, Switzerland, 2014.
-
R. F. Da Silva and G. F. M. de Souza, “Modeling a maintenance management framework for asset management based on ISO 55000 series guidelines,” Journal of Quality in Maintenance Engineering, Vol. 28, No. 4, pp. 915–937, Oct. 2022, https://doi.org/10.1108/jqme-08-2020-0082
-
D. L. Nastasie, A. Koronios, and A. Haider, “Integration through standards – an overview of internal information standards for engineering asset,” in Definitions, Concepts and Scope of Engineering Asset Management, pp. 239–258, Jan. 2010, https://doi.org/10.1007/978-1-84996-178-3_12
-
S. Cho, K. Nguyen, and J. M. Wetzer, “Conceptual design for asset management system under the framework,” ISO 55000, 2015.
-
D. Ghosh and S. Roy, “A decision-making framework for process plant maintenance,” European Journal of Industrial Engineering, Vol. 4, No. 1, pp. 78–98, Jan. 2010, https://doi.org/10.1504/ejie.2010.029571
-
Khaled El-Akruti, “Research methodologies for engineering asset management,” in Research Methodologies for Engineering Asset Management, Jan. 2010.
-
A. K. Parlikad, C. Pearson, J. Trueman, and S. Coomber, “Engineering asset management: issues and challenges,” in Cambridge Service Week workshop on the future of Asset Management, 2013, https://doi.org/10.1080/24725854.2020.1869871
-
R. K. Mobley, An Introduction to Predictive Maintenance. Elsevier, 2002.
-
J. Moubray, “Reliability-centered maintenance,” Industrial Press Inc., 2001.
-
M. Terminology, “CEN (European Committee for Standardization),” European Standard EN, Vol. 13306, p. 2010, 2010.
-
T. Nowakowski, A. Tubis, and S. Werbińska-Wojciechowska, “Evolution of technical systems maintenance approaches – review and a case study,” in Advances in Intelligent Systems and Computing, pp. 161–174, Aug. 2018, https://doi.org/10.1007/978-3-319-97490-3_16
-
H. Lind and H. Muyingo, “Building maintenance strategies: planning under uncertainty,” Property Management, Vol. 30, No. 1, pp. 14–28, Feb. 2012, https://doi.org/10.1108/02637471211198152
-
Ahmed El-Kamash and Mohamed Adel, “A new framework of reliability centered maintenance,” Jordan Journal of Mechanical and Industrial Engineering, Vol. 13, No. 3, Jan. 2019.
-
A. Crespo Márquez, P. Moreu de León, J. F. Gómez Fernández, C. Parra Márquez, and M. López Campos, “The maintenance management framework: A practical view to maintenance management,” Journal of Quality in Maintenance Engineering, Vol. 15, No. 2, pp. 167–178, May 2009, https://doi.org/10.1108/13552510910961110
-
S. Wan, J. Gao, D. Li, Y. Tong, and F. He, “Web-based process planning for machine tool maintenance and services,” Procedia CIRP, Vol. 38, pp. 165–170, Jan. 2015, https://doi.org/10.1016/j.procir.2015.07.018
-
W. Terkaj, T. Tolio, and M. Urgo, “A virtual factory approach for in situ simulation to support production and maintenance planning,” CIRP Annals, Vol. 64, No. 1, pp. 451–454, Jan. 2015, https://doi.org/10.1016/j.cirp.2015.04.121
-
R. Jamshidi and M. M. S. Esfahani, “Maintenance policy determination for a complex system consisting of series and cold standby system with multiple levels of maintenance action,” The International Journal of Advanced Manufacturing Technology, Vol. 78, No. 5-8, pp. 1337–1346, Jan. 2015, https://doi.org/10.1007/s00170-014-6727-1
-
S.-H. Ding, S. Kamaruddin, and I. Abdul Azid, “Maintenance policy selection model – a case study in the palm oil industry,” Journal of Manufacturing Technology Management, Vol. 25, No. 3, pp. 415–435, Apr. 2014, https://doi.org/10.1108/jmtm-03-2012-0032
-
A. Bousdekis, B. Magoutas, D. Apostolou, and G. Mentzas, “A proactive decision making framework for condition-based maintenance,” Industrial Management and Data Systems, Vol. 115, No. 7, pp. 1225–1250, Aug. 2015, https://doi.org/10.1108/imds-03-2015-0071
-
I. Martón, A. I. Sánchez, and S. Martorell, “Ageing PSA incorporating effectiveness of maintenance and testing,” Reliability Engineering and System Safety, Vol. 139, pp. 131–140, Jul. 2015, https://doi.org/10.1016/j.ress.2015.03.022
-
A. K. Verma, A. Srividya, and P. G. Ramesh, “FIS based selection of CM system design parameters from a multi-objective optimisation model using GA,” International Journal of System Assurance Engineering and Management, Vol. 2, pp. 14–20, Apr. 2011, https://doi.org/10.1007/s13198-011-0050-0
-
A. T. de Almeida and G. A. Bohoris, “Decision theory in maintenance decision making,” Journal of Quality in Maintenance Engineering, Vol. 1, No. 1, pp. 39–45, Mar. 1995, https://doi.org/10.1108/13552519510083138
-
M. Velasquez and P. Hester, “An analysis of multi-criteria decision making methods,” International Journal of Operations Research, Vol. 10, pp. 56–66, 2013.
-
E. Triantaphyllou, B. Kovalerchuk, L. Mann, and G. M. Knapp, “Determining the most important criteria in maintenance decision making,” Journal of Quality in Maintenance Engineering, Vol. 3, No. 1, pp. 16–28, Mar. 1997, https://doi.org/10.1108/13552519710161517
-
N. Kadoić, N. B. Ređep, and B. Divjak, International Series in Operations Research and Management Science. Springer US, 2006, https://doi.org/10.1007/0-387-33987-6
-
A. Shahin, H. Shirouyehzad, and E. Pourjavad, “Optimum maintenance strategy: a case study in the mining industry,” International Journal of Services and Operations Management, Vol. 12, No. 3, pp. 368–386, Jan. 2012, https://doi.org/10.1504/ijsom.2012.047626
-
W. Gu, T. L. Saaty, and L. Wei, “Evaluating and optimizing technological innovation efficiency of industrial enterprises based on both data and judgments,” International Journal of Information Technology and Decision Making, Vol. 17, No. 1, pp. 9–43, Jan. 2018, https://doi.org/10.1142/s0219622017500390
-
F. I. Khan and S. A. Abbasi, “Models for domino effect analysis in chemical process industries,” Process Safety Progress, Vol. 17, No. 2, pp. 107–123, Jun. 2004, https://doi.org/10.1002/prs.680170207
-
F. I. Khan, “Use maximum-credible accident scenarios for realistic and reliable risk assessment,” Chemical engineering progress, Vol. 97, No. 11, pp. 56–64, 2001.
-
C. Chen, G. Reniers, and N. Khakzad, “A thorough classification and discussion of approaches for modeling and managing domino effects in the process industries,” Safety Science, Vol. 125, p. 104618, May 2020, https://doi.org/10.1016/j.ssci.2020.104618
-
V. Cozzani, G. Gubinelli, G. Antonioni, G. Spadoni, and S. Zanelli, “The assessment of risk caused by domino effect in quantitative area risk analysis,” Journal of Hazardous Materials, Vol. 127, No. 1-3, pp. 14–30, Dec. 2005, https://doi.org/10.1016/j.jhazmat.2005.07.003
-
G. Antonioni, G. Spadoni, and V. Cozzani, “A methodology for the quantitative risk assessment of major accidents triggered by seismic events,” Journal of Hazardous Materials, Vol. 147, No. 1-2, pp. 48–59, Aug. 2007, https://doi.org/10.1016/j.jhazmat.2006.12.043
-
E. Renni, G. Antonioni, S. Bonvicini, G. Spadoni, and V. Cozzani, “A novel framework for the quantitative assessment of risk due to major accidents triggered by lightnings,” Chemical Engineering Transactions, Vol. 17, pp. 311–316, May 2009, https://doi.org/10.3303/cet0917053
-
A. de Montis, M. Barthélemy, A. Chessa, and A. Vespignani, “The structure of interurban traffic: a weighted network analysis,” Environment and Planning B: Planning and Design, Vol. 34, No. 5, pp. 905–924, Jul. 2016, https://doi.org/10.1068/b32128
-
W. de Haan et al., “Functional neural network analysis in frontotemporal dementia and Alzheimer’s disease using EEG and graph theory,” BMC Neuroscience, Vol. 10, No. 1, pp. 1–12, Aug. 2009, https://doi.org/10.1186/1471-2202-10-101
-
D. J. Watts and S. H. Strogatz, “Collective dynamics of ‘small-world’ networks,” Nature, Vol. 393, No. 6684, pp. 440–442, Jun. 1998, https://doi.org/10.1038/30918
-
K. Supekar, V. Menon, D. Rubin, M. Musen, and M. D. Greicius, “Network analysis of intrinsic functional brain connectivity in Alzheimer’s disease,” PLoS Computational Biology, Vol. 4, No. 6, p. e1000100, Jun. 2008, https://doi.org/10.1371/journal.pcbi.1000100
-
B. Abdolhamidzadeh, T. Abbasi, D. Rashtchian, and S. A. Abbasi, “A new method for assessing domino effect in chemical process industry,” Journal of Hazardous Materials, Vol. 182, No. 1-3, pp. 416–426, Oct. 2010, https://doi.org/10.1016/j.jhazmat.2010.06.049
-
F. Wu, S. A. Niknam, and J. E. Kobza, “A cost effective degradation-based maintenance strategy under imperfect repair,” Reliability Engineering and System Safety, Vol. 144, pp. 234–243, Dec. 2015, https://doi.org/10.1016/j.ress.2015.08.002
-
E. Gilabert, S. Fernandez, A. Arnaiz, and E. Konde, “Simulation of predictive maintenance strategies for cost-effectiveness analysis,” Proceedings of the Institution of Mechanical Engineers, Part B: Journal of Engineering Manufacture, Vol. 231, No. 13, pp. 2242–2250, Apr. 2015, https://doi.org/10.1177/0954405415578594
-
A. E. Haroun, “Maintenance cost estimation: application of activity-based costing as a fair estimate method,” Journal of Quality in Maintenance Engineering, Vol. 21, No. 3, pp. 258–270, Aug. 2015, https://doi.org/10.1108/jqme-04-2015-0015
-
M. Faccio, A. Persona, F. Sgarbossa, and G. Zanin, “Industrial maintenance policy development: A quantitative framework,” International Journal of Production Economics, Vol. 147, pp. 85–93, Jan. 2014, https://doi.org/10.1016/j.ijpe.2012.08.018
-
D. T. J. Yu and A. L. Wing-Keung, “An economic perspective on predictive maintenance of filtration units,” arXiv preprint arXiv:2008.11070, Jan. 2020, https://doi.org/10.48550/arxiv.2008.11070
-
R. Louhichi, M. Sallak, and J. Pelletan, “A cost model for predictive maintenance based on risk-assessment,” in 13ème Conférence internationale CIGI QUALITA, 2019.
-
A. Berdinyazov, F. Camci, M. Sevkli, and S. Baskan, “Economic analysis of maintenance policies for a system,” in 2009 IEEE International Symposium on Diagnostics for Electric Machines, Power Electronics and Drives – SDEMPED, pp. 1–5, Aug. 2009, https://doi.org/10.1109/demped.2009.5292766
-
J. K. Vaurio, “Availability and cost functions for periodically inspected preventively maintained units,” Reliability Engineering and System Safety, Vol. 63, No. 2, pp. 133–140, Feb. 1999, https://doi.org/10.1016/s0951-8320(98)00030-1
-
L. M. Maillart and S. M. Pollock, “Cost-optimal condition-monitoring for predictive maintenance of 2-phase systems,” IEEE Transactions on Reliability, Vol. 51, No. 3, pp. 322–330, Sep. 2002, https://doi.org/10.1109/tr.2002.801846
-
A. van Horenbeek and L. Pintelon, “A dynamic predictive maintenance policy for complex multi-component systems,” Reliability Engineering and System Safety, Vol. 120, pp. 39–50, Dec. 2013, https://doi.org/10.1016/j.ress.2013.02.029
-
A. Salonen and M. Deleryd, “Cost of poor maintenance,” Journal of Quality in Maintenance Engineering, Vol. 17, No. 1, pp. 63–73, Mar. 2011, https://doi.org/10.1108/13552511111116259
-
A. S. B. Tam and J. W. H. Price, “A generic asset management framework for optimising maintenance investment decision,” Production Planning and Control, Vol. 19, No. 4, pp. 287–300, May 2008, https://doi.org/10.1080/09537280802034042
-
H. Meng, X. Liu, J. Xing, and E. Zio, “A method for economic evaluation of predictive maintenance technologies by integrating system dynamics and evolutionary game modelling,” Reliability Engineering and System Safety, Vol. 222, p. 108424, Jun. 2022, https://doi.org/10.1016/j.ress.2022.108424
-
C. Wolf, A. Kirmse, M. Burkhalter, M. Hoffmann, and T. Meisen, “Model to assess the economic profitability of predictive maintenance projects,” in 2019 International Conference on High Performance Computing and Simulation (HPCS), pp. 976–981, Jul. 2019, https://doi.org/10.1109/hpcs48598.2019.9188221
-
T. Cheng, M. D. Pandey, and J. A. M. van der Weide, “The probability distribution of maintenance cost of a system affected by the gamma process of degradation: Finite time solution,” Reliability Engineering and System Safety, Vol. 108, pp. 65–76, Dec. 2012, https://doi.org/10.1016/j.ress.2012.06.005
-
R. Dandotiya and J. Lundberg, “Economic model for maintenance decision: a case study for mill liners,” Journal of Quality in Maintenance Engineering, Vol. 18, No. 1, pp. 79–97, Mar. 2012, https://doi.org/10.1108/13552511211226201
-
Y. He, X. Han, C. Gu, and Z. Chen, “Cost-oriented predictive maintenance based on mission reliability state for cyber manufacturing systems,” Advances in Mechanical Engineering, Vol. 10, No. 1, p. 168781401775146, Jan. 2018, https://doi.org/10.1177/1687814017751467
About this article
This study is supported by the engineering department of James Cook University, Australia and Rockfield Technologies Pty Ltd, Australia.
The datasets generated during and/or analyzed during the current study are available from the corresponding author on reasonable request.
Sagar More: conceptualization, writing-original draft preparation. Rabin Tuladhar: writing-review and editing. Daniel Grainger: writing-review and editing. William Milne: supervision.
The authors declare that they have no conflict of interest.