Abstract
All zones worldwide are working through a once again industrial revolution which is called “Industry 4.0”. It is proposed to have a huge impact on engineering, commercial and standards of livelihood. With the rise and spread of technologies, companies are investing in Construction technologies (c-techs). Still, the success of c-techs relies on individual’ acceptance and adoption of technologies. As per the research of Fortune 500 information construction sector, says that the major hurdles industries are facing are the adoption and rejection of the new technologies. This paper aims to measure the behaviour and actual usuage of individual engineers in acceptance and rejection of certain c-tech, within the framework of Technology acceptance model (TAM) constructs and the extended TAM determinant facilitating condition. The results suggested that strategists and designers must consider that construction technology factors are critical for the success of the business.
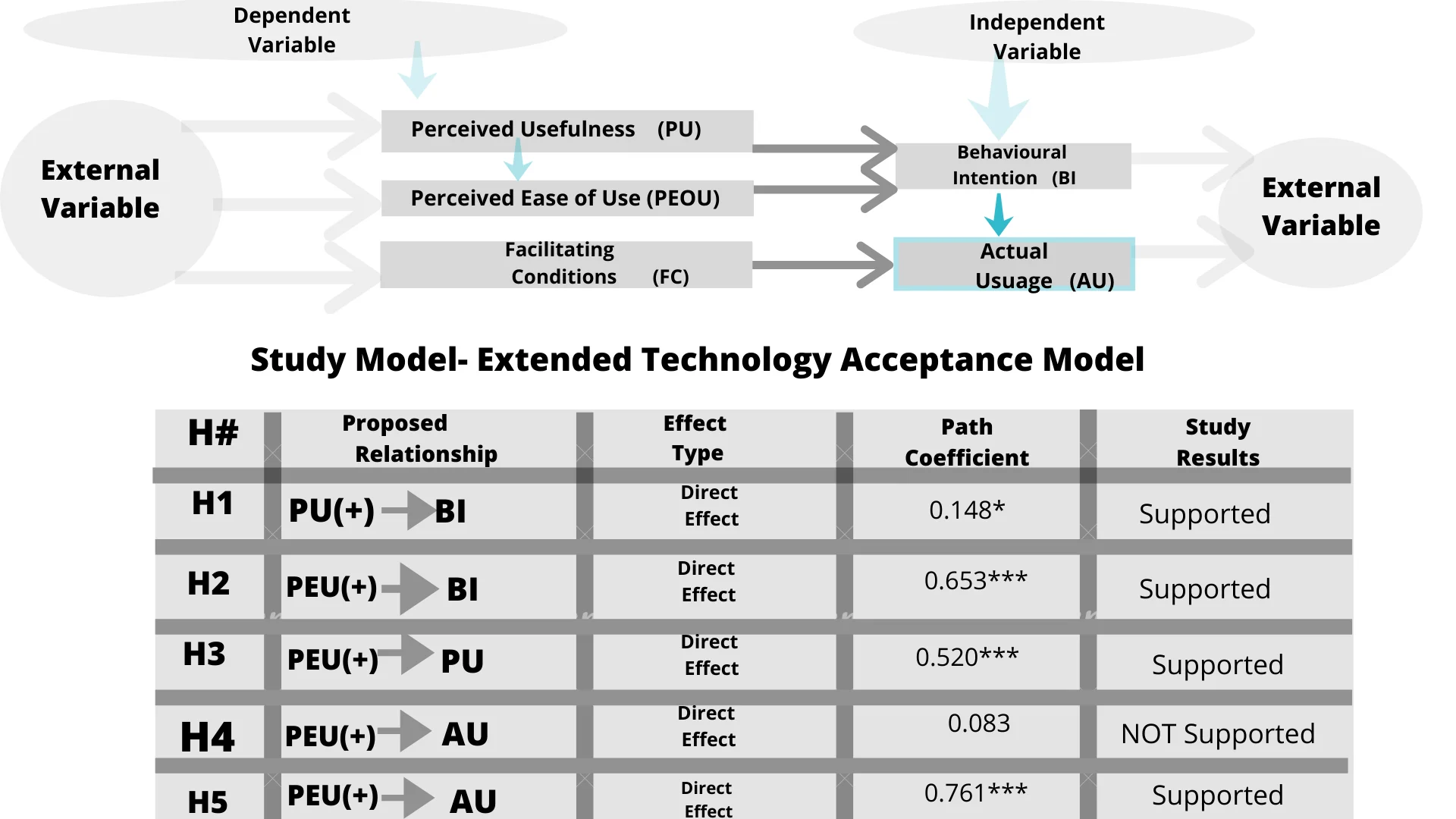
Highlights
- This paper aims to find the adoption and acceptance of emerging technologies in construction industries by engineers
- The extended technology acceptance model (ETAM) with external factors from historical records was developed for research
- The quantitative and qualitative approach has been adopted for the development of the questionnaire and survey, out of 300 questionnaires distributed 248 responses came back
- The data was collected in Statistical Package for the Social Sciences (SPSS) and Structural equation models (SEM) for analytical purposes
- This result states (c-tech) acceptance is when an individual is keen to apply c-tech tools for their responsibilities and when organizations create a structure by facilitating c-techs
- The future investigation must enhance 𝑅2 and improvement of model by putting more factors and finding meaning of non significant hypothesis and viewpoint linked to c-tech adopters and non adopters
1. Introduction
It has been recognized that technology is essential for strengthening construction ventures, still, the point of embracing technology in the construction sector has been low compared to other industries. Over-all industry approximations claim that amongst 16 to 36 per cent of all infrastructure project costs from total budget, based on United states, India, Turkey, and several European and middle east countries research, department of Commerce building evaluated a 13 per cent waste factor is equivalent to almost $95 billion [1]. Due to these mismanagements rework needs to be done otherwise huge business losses can appear. Applying construction technologies such as building information modelling (BIM), [2], cloud computing [3], machine learning [4], Acoustic Emission, this nondestructive method detects the reflected signals from subsurface structures [5], ecological energy technology, Big Data, the Internet of Things, artificial intelligence and smart construction technologies, [6, 7], by using these technologies around out environment a large magnitude of inefficiencies can be solved. The point is, advancements in new construction technology have always driven construction forward, so it’s odd that so many companies are slow to adopt new construction technologies.
A number of professionals and scientific explorers comprehend capable profits of construction technology. Still, numerous bodies are uncertain in what way these technologies can get expended. Former studies examined c-tech background reacceptance and putting into practice from several factors [8]. Maximum of the former revisions focused on architects and the entire engineering. A few of them have observed into the viewpoint from engineers’ the main focus of the investigation will remain on the individual level of engineer’s adoption and acceptance of technologies. As stated by [9], employers are the key prime move and spenders in prosperous recognition of construction technologies in industries.
As stated by [10] to identify behavioural intentions and actual usage this study applied the eminent model extended technology acceptance model (ETAM).
This investigation is organized as under- Initially, TAM previous studies have been revised and then c-techs recognition in construction sector is well-stated. Next, founded on a literature assessment, measured items of the c-techs recognition model and all-inclusive hypothesis are suggested. Then, the tactics and results are reported. In conclusion, academic and executive recommendations and guiding principle for approaching examination have discoursed. Examination model attained a sample of skilled and qualified users (architects and engineers) of these technologies. Using AMOS 23.0 version of software [21], for hypothesis analysis, Structural Equation Modelling (SEM) was employed. A two-point method was initiated, Primarily, a measurement model was valued by means of Confirmatory factor analysis (CFA) to examine the complete fit of the model and reliability. Next, the hypotheses were verified between constructs by means of the model analysis.
2. Literature evaluation
2.1. Acceptance behavior linked concepts
As stated [11], technology acceptance is about in which manner entities decide to take and accept a number of technologies for practice. Employers recognition of technology, is defined as the obvious inclination to take on information technology (IT) which is meant for everyday job [12]. However, technology is not considered valuable, unless it is recognized and practised by individuals.
The TAM is an adaptation of the philosophy of reasoned action and philosophy of planned behaviour exactly fitted for modelling user acceptance of technologies [10]. We are quite conscious, that TAM puts forward a description of the factors of technology acceptance that can define user behaviour towards actual usage of technologies in their daily schedule of work. In the time being, behavioural intentions of technology acceptance are in due course influenced by perceived usefulness and perceived ease-of-use, which are affected by means of numerous external variables. Advancement of acceptance behaviour-correlated ideas, the TAM has been largely used on the way to observe behavioural acceptance of emerging technology.
2.2. Construction technology acceptance in the construction industry
The Construction Industry Institute illustrates construction technology as “the group of innovative tools, machinery, modifications, software etc., implemented throughout the construction life-cycle of a project that allows advancement in-field of the construction techniques, including semi-automated and automated construction equipment”. According to [13, 14], c-tech recognizes the properties of each project and organization objectives and identifies the relationships between these assets. The above-mentioned allows variations to be applied instantaneously to each project assets, thus supporting more rapidly, enhanced project facilities all over the whole production method. Once c-techs are used, numerous kinds of data granted by the (AEC) engineering can be applied proficiently [5]. The below are the external factors which can be facilitated to an individual by their bosses or organization and can accepted or rejected and hence can result in one’s intentions to actual usage of these technologies.
– (ML) Machine learning models can attain big statistics of health record, productivity, quality and safety of employees in organization [4].
– (VR) Virtual reality can attain more effortless to clearly display the project work and eradicate miscommunication between designers, professionals and their clients [3, 4].
– (AI) Artificial intelligence models can prevent cost overruns, much enhanced designs for buildings via generative designs, supports risk mitigation and will address labour shortages [3, 4].
– (CC) Cloud computing models can attain Intelligent schedule administration, decision-making, cost control, quality control, Intelligent safety analysis and alarm approaches [3].
– (AE) Acoustic Emission can bring the finest nondestructive techniques between all features of structural health monitoring (SHM), it will be able to monitor and control all location of cracks without harming the structure [5].
– (TI) Technology information, companies are using data make enhanced decisions, increase productivity, better jobsite safety, and decrease risks can resolve Structure failure, falling from the rooftop while working in a restricted space with (TI) organizations can be able to turn the information they have gathered over the time-period and forecast future results on projects life-cycle [8].
– BIM (Building information modelling) assists from the conception stage to the handover stage of the project. It allows engineers to create a project on computer before building in field. It does not do clash identification, model-based amount estimation supervision, more efficient but helps to decrease material costs [2].
– (IoT) Internet of Things facilitates equipment tracking makes available firms to achieve utilization, control costs and make up smarter judgments. Real-time tracking and cloud-based data sets help out firms cut theft, improve efficiency and manage usage costs [6, 7].
The merits of c-tech in the firms contain provision intended for visual elements and an information supervision setting. C-tech not only makes available information associated with above-mentioned factors but also allows “Professional engineering services” [3]. C-tech implementation in the construction firms has been lacking because of the subsequent hindrances.
– Unsure and overturned profits of c-tech in current performs.
– Absence of acquaintance with implementing these latest technologies.
– Absence of teaching, training facilities for use of c-techs.
– Absence of resources (hardware, software) to practice c-tech means.
As discussed, c-tech allows unified information well-matched and distribution of the material in altogether phases of lifespan of projects. Accordingly, the acceptance of c-tech is probably after an individual is keen to apply the information and mechanical tools of c-tech in everyday jobs and the associated group is ready to begin a collaboration structure that functions c-tech [6].
3. Conceptual work
In this study, the main factors of c-tech acceptance were primarily carefully chosen from the aforesaid TAM theories and numerous studies [17]. Previously, an inquiry form was established to gather views from qualified handlers about c-tech recognition. The validity of the 21 items was verified by means of head-on meetings with three specialists with 6 years of involvement and recognize that c-tech may be implemented for their everyday jobs. Specialists were requested to examine the questionnaire for correctness and redundancy. Next, the discussion, factors were lowered to 18.
The pilot study was conducted from several multi-national construction companies, the feedback form was circulated to a sample of ( 60) to engineers with the c-tech companies in a period of one and a half week. As stated by [15] the trial dimensions for the pilot study must be moderately minor (equal to 100) then the members for the sample was inspected, 60 questionnaires were spread, 44 taken back which shows a peak comeback rate of (73.3 %). The figure of functioning questionnaires was 18, which were inspected. The act of taking it to the conclusion the agreed count of the inquiry form was 8 minutes. Cronbach’s Alpha was applied to verify reliability [16]. The outcomes recommend acceptable reliability, ranging between .882 for BI to .952 for PU.
The following steps involved testing out of 300, 248 responses gathered by the established questionnaire has applied the reliability of the main study was observed by calculating alpha. Outcomes recommend tolerable reliability, ranging from .897 for PEOU to .92 for FC. These measures suggested framework were positively interrelated to each other, therefore approving calculation was mathematically reliable.
Fig. 1 demonstrates the whole theoretical model and the subdivisions and validates the suggested relationships amongst the independent and dependent constructs taking into consideration previous outcomes from the several gathered works.
Fig. 1Study model: PU – perceived usefulness, PEU – perceived ease of use, FC – facilitating conditions, BI – behavioural intentions, AU – actual usage intention
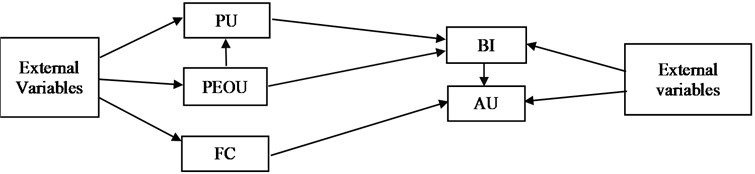
3.1. Perceived ease of use (PEOU)
(PEOU) Perceived Ease of Use is described as “the degree to which an individual trust that using a specific system would be free of effort” In the TAM, TAM 2 and (DTPB) Decomposed Theory of Planned Behavior, PEOU was hypothesized as a direct factor of BI [10]. Still, numerous scientists bring into being a reinforcement to the indirect association of PEOU on BI over PU [19]. Strong confirmation reinforced the vital part that PEOU shows in forecasting the BI.
In the framework of this research, the presence of PEOU was to explore engineers’ opinions of whether or not the system would function without much labour to predict their behavioural intention to use the c-tech structure. We recommend the subsequent hypothesis:
H1: PU will have a positive effect on the intention to use c- tech.
H3: PEOU will have a positive influence on PU to use c-tech.
3.2. Perceived usefulness (PU)
(PU) Perceived Usefulness is described as “the degree to which an individual has trust in that via a specific structure must develop his/her professional working effectiveness”. PU is alike relative gain in the model DOI and working effectiveness hope in UTAUT. In other difference of opinion, it is the range to which profits are weighty responsibilities. In the TAM, TAM2 and Improved TAM, PU was hypothesized as a direct factor of BI. Additionally, numerous investigators have presented proof of its direct factors on AU [17].
In the current circumstantial of the research, PU was used to examine the engineers’ certainty about the possible merits of using the construction-technology structure. Numerous studies have emphasized the significant regulation PU shows on BI to practice technological tools [10].
H2 – PU will have a positive impact on the intention to use the c-tech.
3.3. Facilitating service conditions (FC)
(FC) Facilitating Service Condition is described as “the extent to which a person accepts as true that a structural and methodological substructure is to encourage and bring into play practice of the system” [17]. Exactly, it takes in the approachability of external back up supply (time, money and effort), which is recognized in the works as Resource Facilitating Conditions “RFC” [18].
For instance, the association between FC and BI was discovered to be important in numerous revisions. Therefore, the significance of the outward impact of enabling circumstances on policymaking development is a vital predecessor for behavioural tasks.
H4 – FC will have a positive outcome on actual intention usage to implement c-tech.
3.4. Behavioural intentions for acceptance of construction technologies (BI)
(BI) Behavioural Intention is described as an individual’s perceived probability or personal likelihood that he or she will involve in certain behaviour. The chief variances in (TRA) Theory of reasoned action are considered in BI. It is well-thought-out an instant predecessor of behaviour which provides a recommendation regarding entities’ eagerness to do a precise work [17]. While in TAM, together PU and PEOU impact a person’s intent to practice the technology, that in likelihoods impact the routine behaviour [19].
Within this framework of the investigation, structure usage was considered by means of an endogenous variable and is repeatedly calculated by lone BI [17, 18] or by lone AU [19] or together BI and AU [18]. The present study measured both BI and AU as endogenous variables in the hypothetical background. It is expectable that BI will impact in forecasting the function behaviour of engineers to admit the construction technology in the forthcoming applying procedures. The suggested model comprises 18 observed indicators illustrating 5 latent constructs. The researcher suggests the subsequent hypotheses:
H5 – BI will have a positive result on his or her intention of construction technology.
4. Methodology and results
4.1. Descriptive analysis
Reliable with former experimental exploration in technology acceptance and technological background done by numerous academicians and practitioners like as [19], present research implemented a quantitative method to exam the projected model (see Table 1).
Data were gathered by way of self-administered questionnaires from Turkey, India and some functional managers, senior project manager respondents from across the globe via LinkedIn were also the contributors like South Korean, Middle East, Philippian who use construction technologies in their profession. The sample’s age span wide-ranging as of 24 to 55 years old, with 69.4 % (150 contributors) graduates and 45.7 % (98 contributors) master’s; their self-allied c-tech experience was what's more some or skilled, moreover an in-between or proficient rank in using emerging technologies. Males denote 58.4 % (145 contributors) of model and females denote 41.5 % (103 participants). It is important observing that all constructs and their dimensions were selected from previous works and calculated on a 5-point Likert rule, it stated to be valid and reliable to calculate constructs. Moreover, the descriptive information presented that the widely held of contributors specify positive answers are calculated in this research (see Table 1). Entire means remained greater than 5 for the exogenous constructs (PEOU, PU, FC) and 4.21 for the endogenous constructs (BI and AU). Our outcomes were expected in facilitating service condition construct provided by the administration or government as the previous literature suggests the c-tech system is still in its infancy [19].
Table 1Descriptive statistics of the constructs
Construct | Mean | Std deviation | Cronbach alpha |
Perceived ease of use (PEOU) | 3.697 | 1.281 | 0.897 |
Perceived usefulness (PU) | 3.694 | 1.319 | 0.929 |
Facilitating conditions (FC) | 3.810 | 1.297 | 0.929` |
Behavioural intention (BI) | 3.788 | 1.366 | 0.953 |
Actual usage (AU) | 3.824 | 1.433 | 0.923 |
4.2. Results
4.2.1. Item reliability
Even though all the elements put to use in this research were extracted from the published collected works and are described reliable, in AMOS software, dimension scale consistency was observed by investigative all elements, and by means of estimating the relationship among all element and its conforming paradigm. As stated by [20], the elements’ filling and the element-construct relationship must be minimum 0.60; results of all element construct relationships surpassed 0.7 Correspondingly, it determined that elements computing all constructs had acceptable consistency.
4.2.2. Construct reliability and validity
The reliability of the constructs was examined by Cronbach’s Alpha. It computes how the set of elements computes an only one direction latent construct. Unlike reliabilities, values were well-thought-out reasonable by diverse scholars. Such as, it must be at minimum 0.7 as stated by [16] or 0.6 is measured acceptable whereas a value of 0.8 or greater is favoured rendering to [16]. Assumed all constructs had absolute consistency results 0.8 and mean difference take out results greater than 0.5 and results, examined by SPSS, greater than 0.6, (See Table 1) proposes that the constructs had satisfactory reliability. Moreover, validity of constructs can be measured by means of discriminant and convergent validity. No convergent or discriminant validity at the construct or item level were found (see Fig. 2).
Fig. 2The measurement model: percei (PEU) – perceived ease of use, useful (PU) – perceived use, facilia (FC) – facilitating condition, behav (BI) – behavioural intention, actua (AU) – actual usage
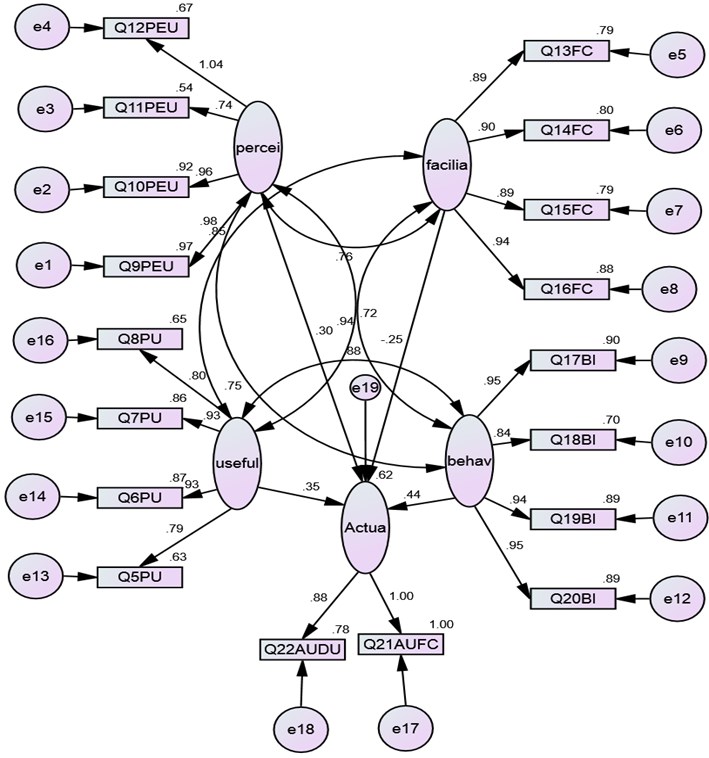
4.2.3. The structural model analysis and hypothesis testing
With its extensive and widespread support amongst researches in IS, behavioural and social science [21], the SEM, as well identified as path examination, covariance structure examination, simultaneous calculation models is brought into play to assess and inspect the hypothesized associations amongst variables in the planned theoretical prototypical see Fig. 2; Fig. 3.
Fig. 3Structural model: ease (PEU) – perceived ease of use, useful (PU) – perceived use, facilit (FC) – facilitating condition, behav (BI) – behavioural intention, actual (AU) – actual usage
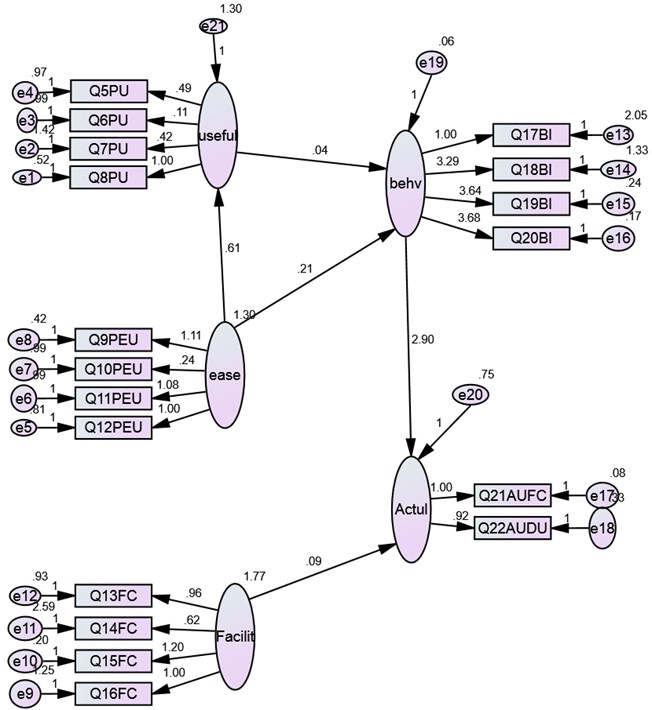
Table 2Model fit summary for the final measurement and structural model
Fit indices | Fit values | ||
Index | Criterion | Measurement | Structural |
0.05 | 286.053 | 332.273 | |
df | n/a | 112 | 112 |
/df | < 5 or < 3 | 2.55 | 2.72 |
GFI | > 0.90 | 0.896 | 0.875 |
AGFI | > 0.80 | 0.840 | 0.82 |
CFI | > 0.90 | 0.969 | 0.925 |
RMSR | < 0.10 | 0.040 | 0.51 |
RMSEA | < 0.08 | 0.079 | 0.084 |
NFI | > 0.90 | 0.951 | 0.888 |
PNFI | > 0.60 | 0.699 | 0.708 |
Predictable values of fit indices have revealed the good structural model fit to the statistics for the suggested examination model in this research see Table 2.
Table 3The summary of direct hypothesied results for the sample
H# | Proposed relationship | Effects type | Path coefficient | Study results |
Perceived usefulness, Behavioural Intention and Actual Usage Prediction | ||||
H1 | PU (+) → BI | Direct effect | 0.148* | Supported |
H2 | PEU (+) → BI | Direct effect | 0.653*** | Supported |
H3 | PEU (+) → PU | Direct effect | 0.520*** | Supported |
H4 | FC (+) → AU | Direct effect | 0.083 | Not Supported |
H5 | BI (+) → AU | Direct effect | 0.761*** | Supported |
Notes: * 0.1; ** 0.05; *** 0.001; NS 0.1 |
The outcomes of the relationships which were hypothetically proposed can be shown in Table 3, PU ( 0.148*; 0.01) and PEOU ( 0.653***; 0.001) brought into being to possess a significant positive effect on BI to use the construction-technology structure, supporting H1 and H2 and also PEOU ( 0.520***; 0.001) was found to have a statistically significant positive effect on perceived usefulness, supporting H3 hypothesis. It must be notable that engineers were found to be extremely affected by their co-workers and trainers in using technology, FC was found to be statistically insignificant, FC ( 0.083; 0.05) not supporting H4. Furthermore, the outcomes also illustrate that actual intention to use is impacted by the BI (0.761***; 0.001) which supports H5.
5. Conclusions
This investigation states that construction technology acceptance is probably when an individual is keen to apply c-tech tools for their responsibilities and when their organization is keen to create a cooperative structure by facilitating c-tech in their environment. Thus, the c-tech acceptance model recommended by this research is composed of five factors that influence the taking part to acceptance of c-tech. More exactly, the discoveries of the general structural model show that the predictors (PEOU, PU) were found to be significant determinants of behavioural intentions and BI was found to be a significant determinant of intention actual usage of the c-tech system also PEOU was found to have a statistically significant positive effect on perceived usefulness with FC found statistically insignificant in intention of usage, our outcomes were expected for FC construct as the previous studies suggest that in emerging countries c-tech system is still in its beginning stages.
The model attains a satisfactory fit and describes 62 % of its variance. The study recognizes that factors influencing c-tech acceptance from an individual engineer’s point of view and assess association amongst the factors. So, the model can be implemented to assess c-tech’s acceptance of a specific person. The outcomes recommend that individual and organizational facilitating condition factors are vital to take into consideration in describing engineers’ behavioural intention or actual usage of c-tech surroundings.
6. Limitation and future research
This study is founded on findings of specific countries. Thus, the outcomes can be examined to India, Turkey, South Korean, Middle East, Philippian or emerging economies. Further, this study was only directed to targeted individuals that were already applying construction technology, and no data was gathered from non-adopters.
The future investigation must enhance value and further improvement of the model by putting in more factors and finding the unknown meaning of nonsignificant hypothesis and viewpoint linked to c-tech adopters and nonadopters.
References
-
Sveikauskas L., Rowe S., Mildenberger J., Price J., Young A. Productivity growth in construction. Journal of Construction Engineering and Management, Vol. 142, Issue 10, 2018, https://doi.org/10.1061/(ASCE)CO.1943-7862.0001138.
-
Okakpu A., Ghaffarianhoseini A., Tookey J., Haar J. Exploring the environmental influence on BIM adoption for refurbishment project using structural equation modelling refurbishment project using structural equation modelling. Architectural Engineering and Design Management, Vol. 15, Issue 3, 2019, p. 41-57.
-
Lee P. C., Xie W., Lo T. P., Long D., Tang X. A cloud model-based knowledge mapping method for historic building maintenance based on building information modelling and ontology. KSCE Journal of Civil Engineering, Vol. 23, Issue 2, 2019, p. 3285-3296.
-
Zhang Y., Khan U., Lee S., Salik M. The influence of management innovation and technological innovation on organization performance. A mediating role of sustainability. Sustainability, Vol. 11, Issue 2, 2019, p. 495.
-
Sepasgozaar S. M. E., Shirowzhan S., Changxin C. A scanner technology acceptance model for construction projects. Procedia Engineering, Vol. 180, 2020, p. 1237-1246.
-
Pasini D., Mastrolembo Ventura S., Rinaldi S., Bellagente P., Flammini A., Ciribini A. L. C. Exploiting internet of things and building information modelling framework for the management of cognitive buildings. 2nd International Smart Cities Conference: Improving the Citizens Quality of Life, 2017.
-
Kurth M. H., Keenan J. M., Sasani M., Linkov I. Defining resilience for the US building industry. Building Research and Information, Vol. 47, Issue 4, 2019, p. 480-492.
-
Wang W., Zhang S., Su Y., Deng X. An empirical analysis of the factors affecting the adoption and diffusion of GBTS in the construction market. Sustainability (Switzerland), Vol. 11, Issue 6, 2019, p. 95-113.
-
Aleksandrova E., Vinogradova V., Tokunova Integration of digital technologies in the field of construction in the Russian Federation. Engineering Management in Production and Services, Vol. 11, Issue 3, 2019, p. 38-47.
-
David F. Perceived usefulness, perceived ease of use, and user acceptance of information technology. MIS Quarterly, Vol. 13, Issue 3, 1989, p. 319-40.
-
Ozorhon B., Cinar E. Critical success factors of enterprise resource planning implementation in construction: case of Turkey. Journal of Management in Engineering, Vol. 31, Issue 6, 2015, https://doi.org/10.1061/(ASCE)ME.1943-5479.0000370.
-
Battista V. The impact of emerging technologies on work: a review of the evidence and implications for the human resource function [version 1; peer review: 2 approved, 1 approved with reservations]. Emerald Open Research, 2019, https://doi.org/10.12688/emeraldopenres.12907.1.
-
Lu Y. Journal of Industrial Information Integration Industry 4.0: A survey on technologies, applications and open research issues. Journal of Industrial Information Integration, Vol. 6, 2018, p. 1-10.
-
Li Y., Liu C. Applications of multirotor drone technologies in construction management. International Journal of Construction Management, Vol. 35, Issue 9, 2019, p. 401-412.
-
Hertzog M. A. Considerations in determining sample size for pilot studies. Research in Nursing and Health, Vol. 31, Issue 2, 2008, p. 180-191.
-
Cronbach L. J. Coefficient alpha and the internal structure of tests. Psychometrika, Vol. 16, Issue 3, 2019, p. 297-334.
-
Venkatesh V., Morris M. G., Davis G. B., Davis F. D. An empirical analysis of the factors affecting the adoption and diffusion of GBTS in the construction market. Sustainability Quarterly, Vol. 27, Issue 3, 2019, p. 425-478.
-
Shibeika A., Harty C., Shibeika A., Harty C. Diffusion of digital innovation in construction: a case study of a UK engineering firm Diffusion of digital innovation in construction. Construction Management and Economics, Vol. 33, Issue 5, 2019, p. 453-466.
-
Gefen D., Straub D. The relative importance of perceived ease of use in is adoption: a study of e-commerce adoption. Journal of the Association for Information Systems, Vol. 1, Issue 1, 2018, p. 8.
-
Hair J. F., Ringle C. M., Sarstedt M. PLS-SEM: indeed a silver bullet. Journal of Marketing Theory and Practice, Vol. 19, Issue 2, 2019, p. 139-152.
-
Gefen D., Straub D., Boudreau M.-C. Structural equation modeling and regression: guidelines for research practice. Communications of the Association for Information Systems, Vol. 4, 2000, p. 7.