Abstract
Bearing has gradually become a critical rotational component in many mechanical systems, and its health condition will directly affect the whole system’s working life. Therefore, it is essential to accurately predict bearing’s life through collection and process of performance degradation data. Accelerated degradation testing (ADT) proves to be an effective way. By systematically raising stress levels during operation, information about degradation can be gathered and life under normal condition could be extrapolated based on certain accelerated models. This article mainly discusses the establishment of performance degradation model for ball bearings based on kurtosis index extracted from the vibration data for 2012 PHM data challenge. Then an accelerated model that describes the relationship between degradation model parameters and two common kinds of stress, rotational speed and radial load, can be obtained based on fixed effect linear model. Finally, reliability analysis is implemented based on Wiener process. Our research achievements may provide sufficient support on data and model for bearing’s lifetime prediction.
1. Introduction
Bearing has been occupying such an extremely significant position in many mechanical products that its healthy status may produce an enormous impact on safe operation as well as lifetime of these products. Therefore, continuous condition monitoring and accurate reliability analysis implemented on bearings will enjoy great theoretical significance and engineering application value.
Since vibration data contain rich information that characterizes bearings’ health status, analysis based on them is frequently applied in prognostics and health management (PHM) activities for bearings. However, raw vibration data are always chaotic, which is caused by noises from various sources. Feature extraction proves to be an effective way in reducing the impact of these noises, which, as its name implies, is to extract some typical features that are capable of representing health status of bearings from raw vibration data, and by analyzing their trends, we may make reasonable judgments about bearings’ health status.
In the actual environment, bearings are usually operating under different stress levels, which leads to different degradation and failure behaviors. ADT can be utilized for degradation data collection and analysis in which items are subjected to elevated levels of stress to accelerate the performance degradation process. Proper accelerated models should be correctly selected or established in order to find out the quantitative relationship between degradation behaviors and stress levels.
In this article, degradation model is established in section 3 according to the feature extraction results in section 2. Next, Section 4 mainly discusses the construction of accelerated model that illuminates the correlation between the shape parameter of degradation model and two common kinds of stress. Reliability analysis based on Wiener process and previous results is carried out subsequently. Section 5 contains some conclusions plus some ideas for further work. The architecture of our research can be illustrated by Fig. 1.
Fig. 1Architecture of research contents

2. Feature extraction
Feature extraction aims to analyze some typical features extracted from vibration data for that these features may show regular trends, which are much easier to model. Vibration features mainly fall into two categories. Time domain features include root mean square (RMS), crest factor and kurtosis etc. Frequency domain features include mean square frequency etc. It must be noted that different types of features are suitable for different occasions so that selecting appropriate features is an issue of leading position and crucial importance during vibration data processing.
2.1. Description of vibration data for use
We select a vibration data set of ball bearings which is adopted in IEEE 2012 PHM Data Challenge competition [1]. The data was collected by a platform named PROGNOSTIA, which is able to continuously record temperature and vibration data by performing accelerated life tests (ALT) on ball bearings. The ALTs were conducted under three operating conditions with combinations of different radial loads and rotational speeds. The stress values of each condition and corresponding bearings are listed in Table 1.
Table 1Operating conditions and corresponding bearings
Stress values | Type of Stress | Condition 1 | Condition 2 | Condition 3 |
Radial Load (N) | 4,000 | 4,200 | 5,000 | |
Rotational Speed (rpm) | 1,800 | 1,650 | 1,500 | |
Corresponding bearings | Learning set | Bearing1_1 Bearing1_2 | Bearing2_1 Bearing2_2 | Bearing3_1 Bearing3_2 |
Test set | Bearing1_3 ~ Bearing1_7 | Bearing2_3 ~ Bearing2_7 | Bearing3_3 |
The vibration data of each bearing include two parts: data from horizontal direction and vertical direction, which are collected by two accelerometers placed radially on the external race of the bearings. 2560 samples in a period of 0.1 second are collected every 10 seconds until failure, with sampling frequency being 25.6 kHz. In this article, we only choose the vertical direction data for study, and data from horizontal direction can be processed in the same manner.
2.2. Vibration data processing using band-pass filter
We select all run-to-failure data of 17 bearings offered by organizers after competition to study the degradation behavior based on one certain feature extracted from the vibration data.
A Butterworth band-pass filter with a frequency band of 500 Hz (frequency range of 5.5 kHz to 6.0 kHz) is applied to process the original data [2], while the filter order is defined to be 4. After this step, we can see that 1) the amplitudes of vibration have reduced significantly, 2) noise has dropped to a lower level, both of which will facilitate our further data processing.
2.3. Extraction of kurtosis and data smoothing
After extracting some typical features from band-pass filtered signals, we find that the kurtosis index shows a rising trend in general. In fact, kurtosis is a rather sensitive to vibration and shock signals, which codetermines its fitness for vibration data analysis.
Kurtosis is a numerical statistic that reflects the distribution characteristics of vibration signals. For a set of discrete vibration data, kurtosis can be expressed as:
where represents the number of sampling points, is the vibration amplitude, is mean amplitude and represents standard deviation. When a bearing is operating under healthy state, the amplitude distribution of vibration signals is close to normal distribution due to varieties of uncertainties, and kurtosis value is approximately equal to 3 [3]. With the emergence and development of failures, the distribution will gradually deviates from normal distribution, then kurtosis value will increase until failure.
In consideration that the extracted kurtosis may enjoy fluctuations, a moving average filter method is employed to smooth the time series kurtosis in order to clearly identify trends over time [2]. Results of training bearings and test bearings are shown in Fig. 2(a) and Fig. 2(b) respectively (with moving average of 200 data points).
Fig. 2Smoothed kurtosis extracted from vibration signals of a) training bearings, b) test bearing
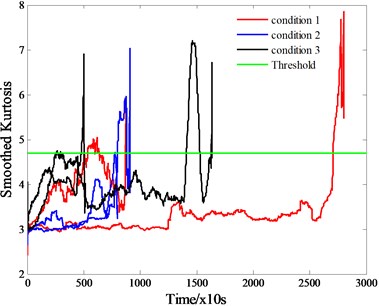
a)
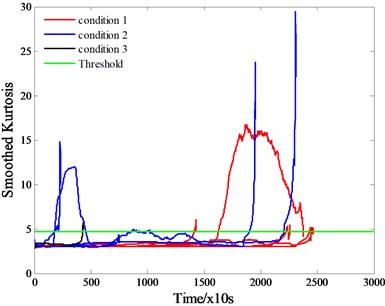
b)
3. Degradation modeling
As shown in Fig. 2, not all of the curves show an increasing trend, such as bearing 2_3 and bearing 3_3. Elimination of these exceptions is reasonably permitted.
Degradation modeling is intended to analyze the degradation behavior of bearings before failure and try to find a model to describe the degradation process. From a visual observation of all curves shown above, we can see that in most cases, the degradation of kurtosis firstly goes through a slowly changing phase, then an abrupt change appears and bearings fail in no time. If we take the whole process to model, the inevitable deviation will be too large to accept. An alternative way is to only model the former part when kurtosis values are under a pre-defined threshold, which will offer helpful guidance for life prediction since the abrupt change is quite transitory. Therefore, the former part will be saved and used for degradation modeling, with the latter mutant part being pruned in this paper.
The threshold mentioned above should be carefully identified. There are no unified standards due to that different filter orders will lead to different extraction results. After inspecting all curves, it is reasonable to make 4.7 be a common threshold. See Fig. 2.
After inspection on characteristics of all curves, an exponential model is embedded in a Wiener process model to form the following degradation model:
where is the function that describes how kurtosis value changes with time, and are fitting parameters, while can be viewed as the shape parameter of the established model which determines the concrete trend. represents a standard Wiener process, and is diffusion coefficient. The model can be simplified by taking an expectation on both sides of the equal sign:
where represents expectation of kurtosis at each time point. According to this model, we are able to obtain ideal degradation trends under various stress levels.
A regression analysis is conducted on the smoothed time series to identify model coefficients. To improve fitting accuracy, model Eq. (3) is transformed to logarithmic form:
where represents natural logarithm.
With the purpose of effectively eliminating the abnormal conditions, a two-step screening process is implemented. The first screening is according to the value of . Values that are too high or low (negative ones included) should be excluded. The second screening is based on a fitting index, R-square. R-square is the coefficient of multiple determination which measures how successful the fit is in explaining the variation of the data in order to verify the fitting accuracy. A value closer to 1 indicates a better fit. In our case, after the first screening step, the first three of the rest bearings with the highest R-square values are finally selected (two bearings of condition 3 are already foreclosed after the first elimination, so there is only one left).
Table 2Model parameters and R-square values of the selected bearings
Condition | Bearings | Time range | Estimation of | Estimation of | R-square |
1 | 1_1 | 1-2697 | 3.004166 | 2.354e-008 | 0.6089 |
1_4 | 1-1415 | 2.915380 | 1.038e-008 | 0.7025 | |
1_6 | 1-2446 | 3.028296 | 2.952e-008 | 0.4932 | |
2 | 2_1 | 1-818 | 3.010180 | 5.032e-007 | 0.817 |
2_2 | 1-778 | 2.900839 | 4.441e-007 | 0.7901 | |
2_5 | 1-2205 | 3.300256 | 2.495e-008 | 0.3868 | |
3 | 3_1 | 1-478 | 3.815226 | 6.671e-007 | 0.4404 |
Estimated model parameters and corresponding R-square values of the selected bearings is shown in Table 2.
4. Accelerated model and reliability analysis
Since the degradation model has been established, an accelerated model that describes the correlation between parameters of this degradation model and stress levels is expected, by which we are able to extrapolate the degradation behaviors under various stress levels. By calculating the mean value of and respectively under each operating condition, the following Table 3 can be obtained.
Table 3Model parameters and stress values under each operating condition
Condition | Radial load (N) | Rotational speed (rpm) | Mean value of | Mean value of |
1 | 4000 | 1800 | 2.992678 | 2.354e-008 |
2 | 4200 | 1650 | 3.070425 | 4.737e-007 |
3 | 5000 | 1500 | 3.815226 | 6.671e-007 |
For parameter , the calculated results in Table 2 are all around 3, which validates the conclusion that the kurtosis value is approximately equal to 3 when bearings are operating under healthy state. Therefore, we may reasonably make equal to 3 in the following discussion. This way, the focus in this section is to seek out the correlativity between the shape parameter and applied stresses. Here, a fixed effects linear model is adopted [4]:
where is the shape parameter, and represent rotational speed and radial load respectively, and is natural logarithm, ~ are model coefficients. This model, naturally, can be used as our accelerated model.
Matrix operations can be implemented in order to find out values of the coefficients. The final result is: Thus the final accelerated model can be expressed as:
Relationship between parameter and two types of stress is shown in a three-dimensional figure, Fig. 3(a), and corresponding contour line with a color bar are drawn in Fig. 3(b). Then the degradation trend of kurtosis under normal stress level (1200 rpm, 3000 N) can be obtained:
After the acquisition of degradation model Eq. (6), we are able to implement reliability analysis process under different stress levels. A linear Wiener process model is applied here:
where represents kurtosis and is the initial value. is degradation rate. Sample data are extracted every 20 time points from the ideal degradation data.
Fig. 3a) Relationship between b and two types of stress, b) corresponding contour line
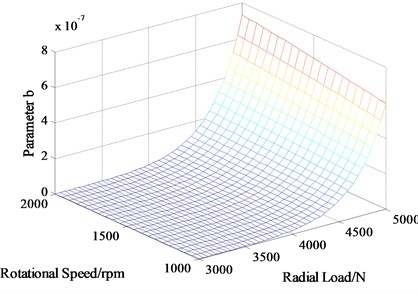
a)
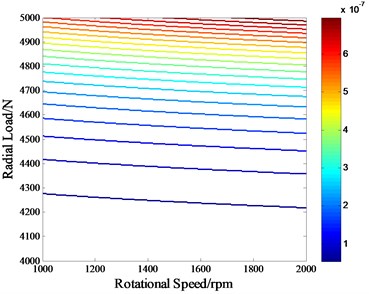
b)
First passage time is a critical parameter in Wiener process representing the time point when degradation curve passes through the failure threshold for the first time, which exactly equals to lifetime of the product. It is a random variable and follows Inverse Gaussian Distribution [5], and the corresponding reliability function can be expressed as:
where is the failure threshold defined before, namely 4.7, is the initial value, 3.0.
The reliability curves under three accelerated conditions and normal condition are drawn in Fig. 4(a) and Fig. 4(b) respectively.
Fig. 4Reliability curves under a) three accelerated conditions, b) normal condition
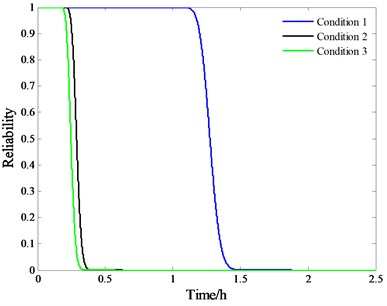
a)
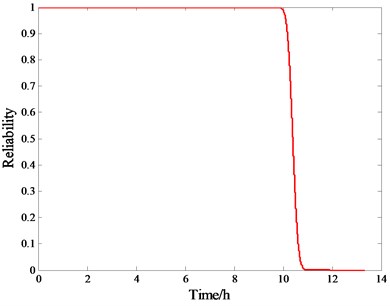
b)
5. Conclusions
This article provides a reliability analysis structure for ball bearings based on kurtosis index including a set of procedures, successively outputting degradation model, accelerated model and reliability curve, all of which may contribute to further study such as RUL prediction and failure diagnostics. However, our results are relatively ideal and inevitably divorced from reality since they are obtained using mathematical and theoretical ways. Thus, future work may focus on discussing and quantifying the uncertainties during operation that may influence the health state of bearings and finding models that are more closed to reality.
References
-
Nectoux P., Gouriveau R., Medjaher K., Ramasso E., Morello B., Zerhouni N., Varnier C. Pronostia: An experimental platform for bearings accelerated life test. IEEE International Conference on Prognostics and Health Management, Denver, CO, USA, 2012.
-
Sutrisno E., Oh H., Vasan A. S. S., Pecht M. Estimation of remaining useful life of ball bearings using data driven methodologies. IEEE Conference on Prognostics and Health Management, Vol. 1, 2012, p. 18-21.
-
Tandon N., Choudhury A. A review of vibration and acoustic measurement methods for the detection of defects in rolling element bearings, Tribology International, Vol. 32, Issue 8, 1999, p. 469-480.
-
Chunwang G., William Q. Meeker, Donna M. Detecting cracks in aircraft engine fan blades using vibrothermography nondestructive evaluation. Reliability Engineering and System Safety, Vol. 131, 2014, p. 229-235.
-
Xiaoyang L., Tongmin J. Constant-stress accelerated degradation testing of satellite assemblies. Acta Aeronautica et Astronautica Sinica, Vol. 28, 2007, p. 101-103.
About this article
This work was supported in part by the National Natural Science Foundation of China under Grant NSFC 61104182.